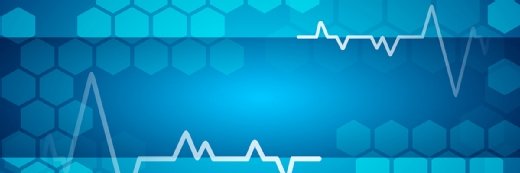
Ace2020/istock via Getty Images
Machine learning tools predict COVID-19 vaccine hesitancy, uptake
New study suggests that principles of behavioral economics – such as risk aversion – may have a significant impact on attitudes around vaccination and immunization.
Researchers from the University of Cincinnati (UC) and Northwestern University have developed machine learning (ML) models that can accurately predict trends in COVID-19 vaccine uptake using reward and aversion judgments, according to a study published last week in the Journal of Medical Internet Research (JMIR) Public Health and Surveillance.
The method uses a combination of demographic data and behavioral economics principles – including risk and loss aversion – to shed light on vaccine hesitancy and vaccination choice. The research team emphasized that so-called ‘judgment variables’ have been shown to impact vaccination choice, but these variables have yet to be used to predict vaccine uptake.
Incorporating these data into a prediction model could allow public health stakeholders to more effectively design vaccination campaigns while relying on a relatively small dataset, the researchers explained.
“We used a small number of variables and minimal computational resources to make predictions,” said lead author Nicole Vike, PhD, a senior research associate in UC’s College of Engineering and Applied Science, in a news release. “COVID-19 is unlikely to be the last pandemic we see in the next decades. Having a new form of AI for prediction in public health provides a valuable tool that could help prepare hospitals for predicting vaccination rates and consequential infection rates.”
To develop the ML models, the research team surveyed 3,476 adults across the United States in 2021. Respondents were asked questions about their income, education, ethnicity, where they lived and whether they had internet access. In addition to this information, the researchers gathered data on participants’ vaccination status and whether they followed four infection control recommendations: washing their hands, wearing a mask, social distancing and not gathering in large groups.
Participants’ demographics were similar to those of the United States per US Census Bureau data. Roughly 73 percent of respondents indicated that they had received one of the available COVID-19 vaccines, compared to 70 percent of the national population at the time.
From there, each participant was tasked with ranking 48 photographs from the International Affective Picture Set – a set of emotionally evocative images – on a seven-point Likert-style scale. This exercise enabled the research team to quantify participants’ judgments in terms of their aversion to risk and loss.
“The framework by which we judge what is rewarding or aversive is fundamental to how we make medical decisions,” said co-senior author Hans Breiter, MD, a professor of computer science at UC. “A seminal paper in 2017 hypothesized the existence of a standard model of the mind. Using a small set of variables from mathematical psychology to predict medical behavior would support such a model. The work of this collaborative team has provided such support and argues that the mind is a set of equations akin to what is used in particle physics.”
The identified judgment variables and demographic data were compared between participants who had been vaccinated versus those who hadn’t. These data were then incorporated into three ML models to test how well judgment, demographic and COVID-19 precaution variables predicted vaccine uptake.
The research team found that a small set of demographic features and 15 judgment variables could be used to forecast vaccine uptake with high precision and moderate to high accuracy. The authors further noted that these results highlight the potential value of using fewer, more interpretable variables in predictive models.
“The study is anti-big-data,” said co-senior author Aggelos Katsaggelos, PhD, an endowed professor of electrical engineering and computer science at Northwestern University. “It can work very simply. It doesn’t need super-computation, it’s inexpensive and can be applied with anyone who has a smartphone. We refer to it as computational cognition AI. It is likely you will be seeing other applications regarding alterations in judgment in the very near future.”
The research underscores the potential of ML models to improve COVID-19 care.
This week, researchers from Johns Hopkins found that a deep learning-based model can detect COVID-19 infection using lung imaging.
The tool utilizes deep neural networks (DNNs) to identify COVID-19 features in lung ultrasound B-mode images, an approach that the research team indicated could help emergency room clinicians triage patients more effectively.
The model’s training used a combination of real-world and computer-generated images, which allowed it to reliably interpret images with high accuracy.