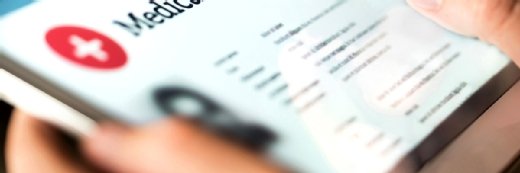
terovesalainen - stock.adobe.com
Online big data dashboard to help map enteric infectious diseases
The Planetary Child Health & Enterics Observatory dashboard aims to shed light on the global public health threat of diarrheal diseases in children.
A team from the University of Virginia (UVA) is developing an online big data dashboard to map enteric infectious disease burden in low- and middle-income countries, which researchers and public health stakeholders can use to guide decision-making.
The tool, outlined in a recent PLOS One study, is part of UVA’s Planetary Child Health & Enterics Observatory (Plan-EO) initiative, which is designed to provide an evidence base for the geographical targeting of child health interventions.
The researchers indicated that diarrheal diseases are a major cause of childhood illness and mortality, and the disease burden of these conditions is increasing as a result of climate change. Mapping transmission hotspots and predicting outbreaks has the potential to save lives, but few tools currently exist to provide these insights for enteric diseases.
The Plan-EO dashboard aims to close this gap.
“Diarrhea is very much the great unmentionable public health threat, often ignored or seen as an unavoidable experience of childhood. We want to change that,” said epidemiologist and Plan-EO initiative lead Josh M. Colston, PhD, an assistant professor in the UVA School of Medicine’s Division of Infectious Diseases and International Health, in the news release. “As patterns of infectious diarrheal diseases shift due to climate change, we want the public health community to be ready and have all the most up-to-date epidemiological estimates and predictions at their fingertips.”
The dashboard is being developed by a coalition of epidemiologists, bioinformaticians, climatologists and hydrologists. This multidisciplinary approach allows the dashboard to use big data from a variety of sources to visualize the impact of weather events – like flooding – on diarrheal disease transmission.
“Awareness is really growing that diseases have multi-faceted risk factors that encompass environmental, social and behavioral elements. We saw that with the pandemic, and we certainly see it with diarrheal diseases. That’s why a collaborative approach is crucial,” stated Margaret Kosek, MD, professor of medicine and an infectious-diseases clinician at UVA.
The dashboard, which is set to be launched later this year, will be continually updated to provide timely insights into pathogen dynamics, similar to Johns Hopkins’ COVID-19 dashboard. Users will be able to utilize a map-based interface to select data on a number of diarrhea-causing pathogens.
The research team hopes that the tool will help public health stakeholders better understand disease burden and predict its impact on children residing in endemic areas, insights that could improve outbreak response.
“Let’s say you’re an epidemiologist in Africa or South Asia and you’re interested in a specific community in a particular country to carry out a water-improvement project or vaccine trial,” said Venkat Lakshmi, PhD, hydrologist and John L. Newcomb Professor of Engineering in Civil and Environmental Engineering at UVA. “Using the Plan-EO interface, you’ll be able to navigate to that location on a map and get robust predictions of the prevalence of particular pathogens, as well as published information on studies that have been carried out in the surrounding areas. It’ll be a gamechanger.”
This research is the latest to highlight how big data tools may serve to enhance outbreak response.
In September, a research team from Yale School of Public Health (YSPH) detailed how an artificial intelligence (AI)-based patient triage platform could help predict disease severity and length of hospitalization during a viral outbreak.
The tool uses machine learning and metabolomics data to flag which patients may need intensive care unit (ICU) admission and which can be sent home, which the researchers noted could improve patient management and resource allocation.
The model utilized COVID-19 as its disease model, combining routine clinical data, patient comorbidity and untargeted plasma metabolomics data to generate predictions.