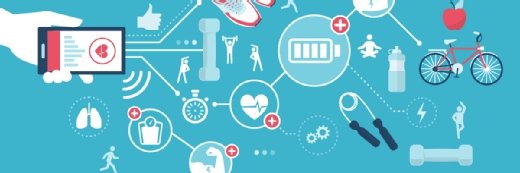
Getty Images
Precision medicine tool predicts breast cancer immunotherapy response
New InteractPrint tool analyzes how breast cancer epithelial cells impact cancer-immune interactions, enabling prediction of immune checkpoint inhibition response.
Researchers from the University of Texas (UT) Southwestern Medical Center have developed a computational tool to predict breast cancer immunotherapy response by analyzing how cancer and immune cells interact.
The research team indicated that forecasting patients’ therapy response has significant potential to inform and improve treatment, as current immunotherapies are limited.
“Immunotherapies have made incredible strides in extending survival for cancer patients, but they only work about 20% of the time. To make immunotherapies more beneficial, we need to have a much better understanding of the cellular composition of specific tumors and how those cells interact with each other,” said Isaac Chan, MD, PhD, assistant professor of Internal Medicine and Molecular Biology and in the Harold C. Simmons Comprehensive Cancer Center at UT Southwestern, in the press release.
The researchers noted that existing immunotherapies rely on the knowledge that many types of cells – including cancer and immune cells – are present in tumors. These immune cells can either attack tumors or promote them.
Current immunotherapies often stimulate T cells, a type of immune cell, to fight cancer. However, other immune cells, such as natural killer (NK) cells, can also help attack cancer cells.
But the research team emphasized that in some cases, interactions between NK cells and cancer cells can “reprogram” the NK cells to promote tumor growth, rather than hinder it. In previous research, Chan and colleagues identified reprogrammed NK cells in breast cancer samples and found that patients with high levels of these cells faced worse outcomes than those with lower levels.
To investigate the potential for therapeutic targeting of reprogrammed NK cells, the research team used single cell RNA sequencing (scRNA-seq) to assess individual gene expression and cancer-immune cell interactions.
Combining eight existing scRNA-seq databases, the researchers developed a single-cell breast tumor atlas consisting of 119 tumor samples from 88 breast cancer patients. The atlas allowed the research team to analyze information from over 236,000 cells.
The analysis revealed 10 categories of cancer cells based on epithelial cell heterogeneity and gene expression.
Currently, three categories of cells – triple negative, hormone positive and HER2 positive – are used to guide therapy planning.
The researchers also determined that the distributions of the 10 cell categories varied among tumors, with most samples containing a mix of cell subtypes. The study further showed that patients with certain combinations of cell subtypes had worse outcomes than their peers.
Using these insights, the research team developed and tested InteractPrint, a precision medicine tool designed to predict breast cancer immunotherapy response. The tool demonstrated high performance when deployed to predict response to immune checkpoint inhibition (ICI) in breast cancer during two clinical trials testing neoadjuvant anti-PD-1 therapy.
The research team highlighted that InteractPrint could prove useful for helping clinicians evaluate the likelihood of immunotherapy response in patients across cancer types, enabling them to choose the treatment with the highest odds of success.
Others are also developing predictive analytics techniques to advance precision medicine.
In April, researchers from Arizona State University detailed the development of a machine learning model to predict how a patient’s immune system would respond to foreign cells.
The tool, a convolutional neural network called human leukocyte antigen (HLA)-Inception, utilizes individualized data on molecular interactions to assess how Major Histocompatibility Complex-1 (MHC-1) proteins affect immune response.
These proteins are critical to the immune system’s ability to recognize and respond to foreign cells.
HLA-Inception sheds light on how MHC-1s interact with foreign peptides, allowing researchers to forecast immune response and potentially personalize treatment.