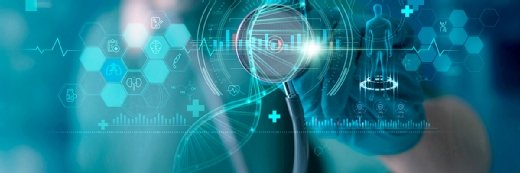
ipopba/istock via Getty Images
Machine learning predicts hospitalization during cancer treatment
Machine learning models using daily step counts and other wearable data accurately predicted unplanned hospitalization during concurrent chemoradiotherapy.
Machine learning tools can accurately forecast an unplanned hospitalization event during concurrent chemoradiotherapy (CRT) using patient-generated health data from wearable devices, according to a study published recently in JAMA Oncology.
The research team indicated that the toxic effects of CRT can result in treatment interruptions and hospitalizations. These can, in turn, lead to increased healthcare costs and reduced treatment efficacy.
The researchers further noted that data from physical activity monitoring has the potential to help identify patients at higher risk for hospitalization who may benefit from proactive interventions.
To assess this hypothesis, the research team developed and validated machine learning tools designed to incorporate daily step counts from the wearable devices of cancer patients undergoing CRT and participating in prospective clinical trials.
The analysis pulled data from 214 patients receiving CRT for a variety of cancers who were enrolled in three prospective, single-institution trials of activity monitoring via wearable devices from June 2015 to August 2018.
Patients in the cohort were followed up during CRT and 1 month following treatment.
Cohorts for model training and validation were generated temporally, stratifying patients based on cancer diagnosis so that 70 percent of participants were in the training sample, and the remaining 30 percent were in the validation sample.
Using these data, the research team trained random forest, neural network, and elastic net–regularized logistic regression (EN) models to predict risk of short-term hospitalization using a combination of patient-generated activity data and clinical information.
To effectively assess the role of wearable data and step counts on predictions, the researchers trained some tools based only on activity-monitoring features and others only on clinical features. Each model’s performance was measured in terms of the receiver operating characteristic area under curve (ROC AUC).
The EN model that incorporated both clinical information and step counts demonstrated the highest performance with an ROC AUC of 0.83 compared to the random forest and neural network approaches, which achieved ROC AUCs of 0.76 and 0.80, respectively.
In the ablation study, which removed either step counts or clinical features to better understand the impact of each, the researchers found that the EN model based on only step counts showed greater predictive performance than the EN model using both clinical features and step counts. The step count-only model achieved an ROC AUC of 0.85, significantly outperforming the clinical feature-only EN, which reached an ROC AUC of 0.53.
These findings led the research team to conclude that patient-generated health data may be valuable, advancing the predictive capabilities of machine learning models to forecast risk for unplanned hospitalizations during CRT.
Moving forward, the EN model based on step counts and clinical features will be assessed in an upcoming multi-institutional, cooperative group randomized trial to further validate the study’s findings.
This research is one example of how machine learning and other approaches can help advance cancer care.
In March, researchers from Washington University School of Medicine in St. Louis shared that they had developed a deep learning-based prediction approach to flag which non-small cell lung cancer (NSCLC) patients were likely to experience brain metastasis.
Brain metastases occur in a significant number of NSCLC patients, but no reliable methods to identify high-risk patients currently exist.
To close this research gap, the team built a deep learning-driven model to predict brain metastasis risk using lung biopsy images. The approach was able to detect abnormal features within a biopsy image significantly better than clinicians.