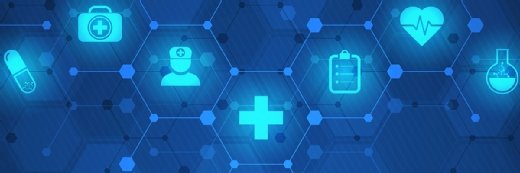
bestbrk/istock via Getty Images
Deep learning tool may reduce false-positives in screening mammography
A deep learning model accurately identified false positive screening mammograms, significantly reducing diagnostic callbacks and unnecessary biopsies.
Researchers have developed a deep learning tool capable of reducing false positives without missing true cases of breast cancer identified by screening mammography, according to a study published this week in Radiology: Artificial Intelligence.
Effective cancer screening is key to improving patient outcomes, but medical images like mammograms can be challenging for clinicians to read, potentially leading to false positives.
“False positives are when you call a patient back for additional testing, and it turns out to be benign,” explained senior author Richard L. Wahl, MD, a professor of radiology at Washington University’s Mallinckrodt Institute of Radiology (MIR) and a professor of radiation oncology, in a news release. “That causes a lot of unnecessary anxiety for patients and consumes medical resources.”
Tools like artificial intelligence (AI)-enabled assistants have the potential to help address the issue of false positives by identifying and removing low-risk mammograms from a radiologist’s workload, allowing them to focus on images suspicious for breast cancer.
However, this requires that an AI model can accurately flag the false positives without also missing true cancer cases. The researchers evaluated a model developed by technology startup Whiterabbit.ai to determine its capabilities in this area.
To do so, the research team conducted a simulation study that tasked the tool with identifying normal mammograms and ruling out cancer. From there, the researchers used real patient data to simulate what would happen if these low-risk images were removed, allowing radiologists to concentrate on the higher-risk mammograms.
The tool was trained on a set of 123,248 2D digital mammograms – 6,161 of which contained cancer – that were collected and evaluated by Washington University radiologists. The model was then validated on three independent datasets from the United States and the United Kingdom.
Using these data, the researchers determined how many patients were called back for secondary screening and biopsies, the results of those tests, and whether each case received a cancer diagnosis. The AI was then applied to the data and the negative mammograms were removed, leaving behind high-risk images to be evaluated by clinicians using standard diagnostic procedures.
For each dataset, use of the deep learning tool led to significant reductions in false positives without missing true cancer cases.
On the first US dataset, the model reduced screening examinations requiring radiologist interpretation by 41.6 percent, diagnostic examination callbacks by 31.1 percent and benign needle biopsies by 7.4 percent.
These trends were seen in the other datasets as well, with reductions of 19.5 percent, 11.9 percent and 6.5 percent in the second US dataset, alongside 36.8 percent, 17.1 percent, and 5.9 reductions in the UK dataset.
“This simulation study showed that very low-risk mammograms can be reliably identified by AI to reduce false positives and improve workflows,” Wahl noted.
These results highlight the potential for AI-driven tools to help reduce clinicians’ workloads without sacrificing care quality or patient outcomes.
“At the end of the day, we believe in a world where the doctor is the superhero who finds cancer and helps patients navigate their journey ahead,” said co-author Jason Su, co-founder and chief technology officer at Whiterabbit.ai. “The way AI systems can help is by being in a supporting role. By accurately assessing the negatives, it can help remove the hay from the haystack so doctors can find the needle more easily. This study demonstrates that AI can potentially be highly accurate in identifying negative exams. More importantly, the results showed that automating the detection of negatives may also lead to a tremendous benefit in the reduction of false positives without changing the cancer detection rate.”
The research was supported by funding from Whiterabbit.ai, and Washington University has equity interests in the company.
These findings underscore the promise of AI in medical imaging analytics.
This week, researchers from Yale School of Medicine and other institutions identified an AI-based video biomarker capable of helping clinicians more accurately flag patients who might develop or have rapidly worsening aortic stenosis.
The tool – Digital [aortic stenosis] Severity index (DASSi) – can capture the condition’s echocardiographic signature, allowing the model to stratify the risk of aortic stenosis development and progression in patients without the condition or with mild or moderate forms of the condition at baseline.