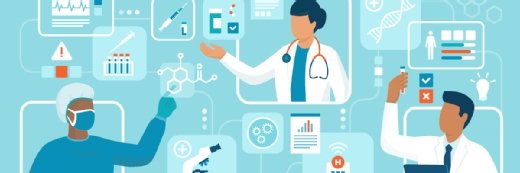
Getty Images
Deep learning tool may advance precision medicine approaches
The deep learning-based Lifelong Neural Network for Gene Regulation tool may shed light on how genetic variations influence a patient's drug response.
Researchers from Clemson University have developed a deep learning tool to better understand how gene-regulatory network (GRN) interactions impact individual drug response, according to a study published recently in Nature Biotechnology.
GRNs “map the complex interactions between genes, regulatory elements and proteins, holding the key to understanding how genetic variations influence [phenotypes] like drug response,” explained Zhana Duren, PhD, an assistant professor in the Department of Genetics and Biochemistry at Clemson, in a news release. “Each individual possesses a unique GRN shaped by their specific genotype, explaining why the same drug can elicit different responses in different people.”
The research team further indicated that the majority of known genetic variants linked to particular diseases lie in parts of a person’s deoxyribonucleic acid (DNA) that do not directly code for proteins, making it difficult to determine the role these variants play in individual health.
To help shed light on these genetic variants and their GRNs, the researchers developed a deep learning tool known as Lifelong Neural Network for Gene Regulation (LINGER).
“[We] aim to answer critical questions, (such as) how and why do genetic variants influence individual phenotypes through intricate GRN interactions,” Duren stated. “By elucidating these mechanisms, we pave the way for predicting drug response based on personal genetics, enabling the development of more-targeted therapies and minimizing ineffective treatments.”
LINGER is designed to infer GRNs from single-cell multiome data.
The researchers underscored that there are existing models designed to predict how GRNs work, but these are limited due to data sparsity.
“Because it is single-cell data, the number of observations we get at each cell is so limited,” Duren noted. “The gene regulatory network is such a complex problem that it requires large amounts of data to learn. But the available independent data — we have data from many single cells, but they are not independent — is not enough for this task.”
However, LINGER overcomes these hurdles by utilizing “atlas-scale external bulk data across diverse cellular contexts and prior knowledge of transcription factor motifs,” allowing researchers to estimate transcription factor activity and gain insights into disease drivers.
“There are many methods developed for gene regulatory network inference in the past two decades,” Duren indicated. “However, our systematic benchmarking based on experimental data shows that the accuracies of these existing methods are about 17% to 29% higher than the random predictor. The new method increases it to 125% higher than the random predictor, showing four- to seven-fold relative increase.”
“Since this is a significant improvement in fundamental research, it will have the potential to lead discovery in broad biomedical research fields,” he continued.
The research team emphasized that LINGER has multiple potential applications in molecular and developmental biology, in addition to precision medicine research.
Moving forward, the researchers will apply the tool in the context of drug addiction to help inform more effective treatments.
Others are also turning to artificial intelligence (AI) methods to better understand patients’ drug responses.
Earlier this month, a research team from Columbia University detailed how a machine learning-based approach can help assess cardiac function and drug response.
The method – known as BeatProfiler – relies on a suite of cardiac analysis tools to quantify contractile function, calcium handling and force generation in cardiac models. Using video data, the approach can analyze heart cells to characterize heart disease and dose-dependent drug response.
The method outperformed existing tools for cardiomyocyte function analysis in terms of accuracy, reliability and efficiency.