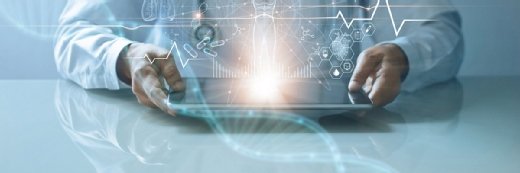
Getty Images/iStockphoto
Computational models forecast pediatric heart valve leakage
New computational models simulate heart valve shape, possible weak spots, and blood flow to help predict potential issues and guide surgery-based preventive efforts.
Researchers from the University of Oklahoma (OU) have developed computational models designed to predict heart valve leakage in children and help guide surgical decision-making.
These tools identify the shape of a child’s valves, possible weak spots and how blood moves through the valves, which may allow clinicians to forecast the future structural integrity of the heart and inform potential surgical steps to prevent adverse outcomes.
The research brings together experts in cardiology and biomedical engineering to better personalize pediatric cardiovascular care.
“This is truly translational medicine,” said Harold Burkhart, MD, an OU Health pediatric heart surgeon and a professor and chief of cardiovascular and thoracic surgery in the OU College of Medicine, in the press release.
“Because of our multidisciplinary collaboration, we have the knowledge together to create a computational model that goes beyond what we are able to see with both 2D and 3D echocardiogram. It allows us to go a step further and visualize the heart as it would be in real life with the characteristics of each individual,” Burkhart continued. “With that understanding, we can test what would happen if we put a stitch here or tighten a valve there — does it put too much stress on the valve, or does it address the problem? It can potentially give us a lot more direction before we even go into the operating room.”
This research began with developing models for hypoplastic left heart syndrome – a birth defect in which the left side of a fetus’ heart doesn’t form correctly, necessitating that the right side of the heart must pump blood to the lungs and the rest of the body.
Children with the condition must undergo a series of three open-heart surgeries to correct this defect. While these initial operations are typically successful, follow-up surgeries are sometimes necessary to repair leaky valves.
The same is true for atrioventricular canal defects – also known as a hole in the heart.
In cases of hypoplastic left heart syndrome, approximately 25 percent of children are estimated to have leaky valves by the time they enter preschool. Children with atrioventricular canal defects can experience valve leakage anywhere from six months to two years after the initial surgical repair.
The reasons for valve leakage in these cases are not yet well understood, but the researchers indicated that the follow-up surgeries to correct this issue are typically only half as successful as the original procedure. Additionally, if the valve cannot be repaired, a child may need a mechanical heart valve inserted, a process that comes with potentially serious risks.
Being able to predict valve damage could address some of these risks, but current techniques to help surgeons view the heart during surgery – such as inflating the valves – are limited.
“Even with 3D echocardiogram, it’s hard to see the finer details of the valves,” explained Arshid Mir, MD, an OU Health cardiologist and an associate professor of pediatric cardiology in the OU College of Medicine. “Most heart valves are like Pringle chips — they are more angular and have high and low points. We want to learn about these valves and try to predict which valves, right at birth, would have a risk of becoming leaky when the child is between 1 and 5 years old, so that we can try to address them surgically at the time of the first repair.”
“What we are doing is moving toward personalized medicine,” Mir added. “How do we think about each patient differently rather than thinking of a condition as a single disease? What is unique about this child’s anatomy that makes him at higher risk for reoperations? This is the way we will be thinking about these diseases as we head into the future.”
The computational models are designed to use 2D and 3D echocardiograms and other data to make their predictions, and the research team aims to continue adding patient data to improve the tools over time.
“Through this image-based computational model, we want to provide the missing information — which patients will have valve dysfunction,” stated Chung-Hao Lee, PhD, a former OU biomedical engineering researcher now at the University of California, Riverside. “If we can better understand which valves have weak spots and may start leaking, surgeons may plan their surgeries differently and cardiologists may want to monitor or follow the patient more closely after surgery.”
This research is the latest effort to build personalized approaches to cardiovascular care.
This week, researchers from the University of Virginia reported that a machine learning tool can accurately assess and predict adverse outcome risks for patients with advanced heart failure with reduced ejection fraction (HFrEF).
The research team underscored that existing models have no robust way to account for missing data or to incorporate invasive hemodynamic data.
The model is designed to tackle these challenges by using either invasive hemodynamics alone or a feature set incorporating noninvasive hemodynamics data to predict patient risk across five categories.