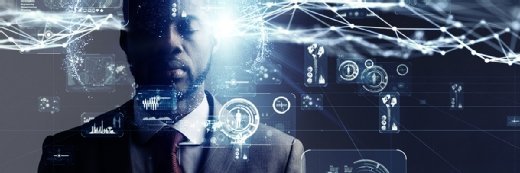
Getty Images
Self-supervised foundation models mitigate bias in histopathology AI
Image classification models perform more accurately in white patients than in Black patients, but foundation models may help close these health equity gaps.
A research team from Mass General Brigham found that standard computational pathology models performed differently across demographic groups, but demonstrated that foundation models can partially mitigate these disparities, according to a recent Nature Medicine study.
The researchers indicated that while artificial intelligence (AI) tools have shown significant potential to advance pathology, the effective use of such technologies is limited due to the underrepresentation of minoritized patient populations and the resulting health equity concerns associated with AI training datasets.
To address this, the research team set out to quantify and reduce the performance disparities of these models across groups via bias mitigation techniques.
Using data from the Cancer Genome Atlas and the EBRAINS brain tumor atlas – both of which include information from mostly white patients – the researchers built computational pathology systems for breast cancer subtyping, lung cancer subtyping and glioma IDH1 mutation prediction.
The models were then tested using histology slides from a cohort of 4,300 cancer patients from Mass General Brigham and the Cancer Genome Atlas. The results were stratified by race to explore potential biases and disparities.
The analysis revealed that overall, the models performed more accurately in white patients than their Black counterparts, with performance gaps of three percent for breast cancer subtyping, 10.9 percent for lung cancer subtyping and 16 percent for IDH1 mutation prediction.
In an effort to reduce these disparities, the research team utilized machine learning-based bias mitigation approaches, such as emphasizing examples from underrepresented populations as part of the model’s training. However, this method only marginally reduced the observed biases.
From there, the researchers examined whether self-supervised vision foundation models – AI tools trained on large-scale datasets for use across a variety of clinical tasks – could further decrease performance gaps. These models allowed the research team to obtain richer feature representations from histology images in an effort to reduce the likelihood of bias.
The foundation model approach led to significant improvements in performance.
“There has not been a comprehensive analysis of the performance of AI algorithms in pathology stratified across diverse patient demographics on independent test data,” said corresponding author Faisal Mahmood, PhD, of the Division of Computational Pathology in the Department of Pathology at Mass General Brigham, in a press release. “This study, based on both publicly available datasets that are extensively used for AI research in pathology and internal Mass General Brigham cohorts, reveals marked performance differences for patients from different races, insurance types, and age groups. We showed that advanced deep learning models trained in a self-supervised manner known as ‘foundation models’ can reduce these differences in performance and enhance accuracy.”
However, despite these improvements, there were still substantial performance gaps across demographic groups, highlighting the need for further model refinement. The research team also indicated that the study was limited in its scope due to the limited number of patients and demographic groups represented in the data used.
Moving forward, the researchers will explore how multi-modality foundation models based on multiple forms of data, like genomics or electronic health records (EHRs), can help overcome these obstacles.
“Overall, the findings from this study represent a call to action for developing more equitable AI models in medicine,” Mahmood noted. “It is a call to action for scientists to use more diverse datasets in research, but also a call for regulatory and policy agencies to include demographic-stratified evaluations of these models in their assessment guidelines before approving and deploying them, to ensure that AI systems benefit all patient groups equitably.”
These efforts are the latest to investigate how AI could advance health equity.
Earlier this month, researchers from George Washington University (GW) School of Medicine and Health Sciences (SMHS) and the University of Maryland Eastern Shore (UMES) were awarded a two-year, $839,000 grant from the National Institutes of Health to support the development of explainable, fair risk prediction models.
The project, known as “Trustworthy AI to Address Health Disparities in Under-resourced Communities” (AI-FOR-U), centers on a theory-based, participatory AI development approach designed to help frontline healthcare workers tackle disparities in the communities they serve.
Teams involved in the project will develop, deploy and assess the fairness and explainability of AI-based risk prediction models within the context of behavioral health, cardiometabolic disease and oncology.