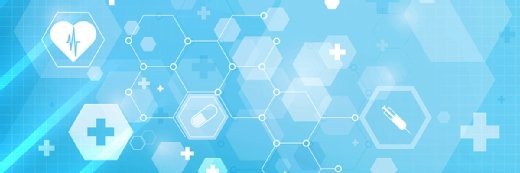
Rifqyhsn Design/istock via Getty
Machine Learning Models Predict Mortality Among Dementia Patients
New machine learning tools accurately predicted patient mortality at one, five, three, and 10 years across eight dementia subtypes.
Researchers at the Icahn School of Medicine at Mount Sinai have developed machine learning (ML) models to identify mortality predictors in dementia patients, according to a study published this week in Communications Medicine.
The research team emphasized that the varying disease progression and outcome trajectories associated with dementia make caring for affected patients a challenge, particularly near end-of-life. Dementia is a major driver of mortality in the United States, resulting in an estimated 66.7 deaths per 100,000 people in 2017. The Centers for Disease Control and Prevention rank Alzheimer's disease as the seventh leading cause of death in the US.
As life expectancy increases, the healthcare burden of dementia is expected to grow as well. Being able to predict dementia prognosis could help address these challenges and improve patient outcomes.
The researchers noted that dementia patient mortality is impacted by a host of factors, and identifying the most significant contributing factors could support risk stratification.
To identify key mortality predictors, the research team turned to ML. The models were developed using data from 45,275 patients and 163,782 visit records from the US National Alzheimer’s Coordinating Center (NACC). These data were used to predict mortality at one, three, five, and 10 years across eight dementia types using clinical and neurocognitive features.
The analysis revealed that dementia-related predictors such as neuropsychological test results were some of the strongest predictors of mortality, while other age-related features, such as cardiovascular conditions and stroke, were less salient.
The findings also highlighted both shared and distinct mortality predictors across dementia types. Overall, all models achieved an area under the receiver operating characteristic curve (AUC-ROC) of over 0.82 at one-, three-, five-, and 10-year survival thresholds.
"Our findings are significant as they illustrate the potential of machine learning models to accurately anticipate mortality risk in dementia patients over varying timeframes," said corresponding author Kuan-lin Huang, PhD, assistant professor of Genetics and Genomic Sciences at Icahn Mount Sinai, in the press release. “By pinpointing a concise set of clinical features, including performance on neuropsychological and other available testing, our models empower health care providers to make more informed decisions about patient care, potentially leading to more tailored and timely interventions."
"The implications of our research extend beyond clinical practice, as it underscores the value of machine learning in unraveling the complexities of diseases like dementia. This study lays the groundwork for future investigations into predictive modeling in dementia care,” Huang continued. “However, while machine learning holds great promise for improving dementia care, it's important to remember that these models aren't crystal balls for individual outcomes. Many factors, both personal and medical, shape a patient's journey."
Moving forward, the research team aims to further refine its predictive models by exploring the potential of deep learning techniques and incorporating additional data, such as genetic information and treatment effects.
Recently, predictive analytics has helped shed light on the drivers of dementia onset and progression.
Last week, researchers from West Virginia University (WVU) detailed how a deep learning model can leverage metabolic biomarkers to predict the development of Alzheimer's disease before clinical symptom onset.
The team utilized the Least Absolute Shrinkage and Selection Operator (LASSO) algorithm to identify potential biomarkers via Ultra Performance Liquid Chromatography Mass Spectrometry (UPLC-MS/MS) data.
A set of 21 biomarkers was selected as most relevant and incorporated into ML models. The models achieved high performance, underscoring the potential of artificial intelligence (AI) for improving Alzheimer's detection.