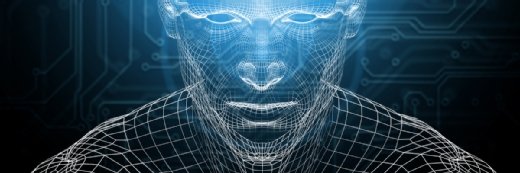
Getty Images
Epic Risk Model Moderately Successful at Predicting Acute Kidney Injury
Epic’s Risk of hospital-acquired acute kidney injury model was found to have high predictive value in some cases, but also high false-positive rates.
Researchers from Mass General Brigham Digital demonstrated that the commercially available, machine learning-based Epic Risk of hospital-acquired acute kidney injury (HA-AKI) model is moderately successful at predicting the condition, according to a study published recently in NEJM AI.
The Epic Risk of HA-AKI model works by combing inpatient encounters hourly for early signs of kidney injury, like increases in serum creatinine levels. However, the researchers noted that HA-AKI’s etiology makes the condition difficult to predict.
Artificial intelligence (AI) and machine learning present opportunities to improve predictive analytics for hospital-acquired conditions, but some stakeholders have raised concerns about the performance of these models and their impact on patient outcomes.
To assess Epic’s model, the research team tasked the tool with predicting the risk of HA-AKI in a cohort of 39,891 adult patients with an inpatient hospital stay within the Mass General Brigham network from August 2022 to January 2023. Outcomes were defined using the Kidney Disease: Improving Global Outcomes (KDIGO) framework for HA-AKI stages.
Patient demographics, comorbidities, principal hospital diagnoses, serum creatinine laboratory results, predictive model scores, and other data were pulled from the cohort’s electronic health records (EHRs) for analysis of encounter-level and prediction-level model performance. The tool’s performance was assessed using the area under the receiver operating curve (AUROC) and the area under the precision recall curve (AUPRC).
The incidence of stage 1 HA-AKI in the cohort was 24.5 percent. At the encounter level, the model’s AUROC was 0.77, and the AUPRC was 0.49. Given a prediction horizon of 48 hours, Epic’s tool reached an AUROC of 0.76 and an AUPRC of 0.19.
The research further indicated that the model had a positive predictive value of 88 percent with a median lead-time warning of 21.6 hours prior to stage 1 HA-AKI.
The researchers concluded that the Epic Risk of HA-AKI tool was moderately successful, but underscored significant limitations.
They noted that the tool was more reliable when evaluating lower-risk patients, confidently identifying low-risk individuals who would not develop HA-AKI. However, the model struggled somewhat with predicting when higher-risk individuals might develop the condition.
Further, the research team found that the tool’s performance varied depending on HA-AKI stage, with predictions for stage 1 disease being more accurate than those for later stages.
The findings also suggest a lower performance of the Epic Risk of HA-AKI model than detailed in the company’s internal validation, in which the tool had a prediction-level AUROC of 0.85 and an encounter-level AUROC of 0.81.
The researchers cautioned that deployment of the model may result in high false-positive rates.
“We found that the Epic predictive model was better at ruling out low-risk patients than identifying high-risk patients,” said lead study author Sayon Dutta, MD, MPH, of Mass General Brigham Digital’s Clinical Informatics team, and an emergency medicine physician at Massachusetts General Hospital, in a news release. “Identifying HA-AKI risk with predictive models could help support clinical decisions such as by warning providers against ordering nephrotoxic medications, but further study is needed before clinical implementation.”
As predictive analytics has become more prominent in healthcare, increased scrutiny of model performance has shed light on the need for clinical validation prior to deployment.
Last week, a research team from the University of Michigan highlighted potential pitfalls of the Epic Sepsis Model (ESM).
Past studies assessing the ESM have indicated that the tool may perform less accurately than expected and lack the timeliness needed to effectively support clinical decision-making.
The University of Michigan team asserted that clinicians often recognize and treat sepsis before a patient meets clinical criteria for the condition, raising questions about the clinical benefits of existing prediction models.
The ESM was evaluated based on its predictive performance throughout patients’ hospitalization and treatment. The analysis revealed that the tool’s accuracy was significantly impacted by whether its predictions were restricted to data collected before a patient met sepsis criteria, indicating that the ESM may unintentionally use clinicians’ suspicions of sepsis to guide its predictions.