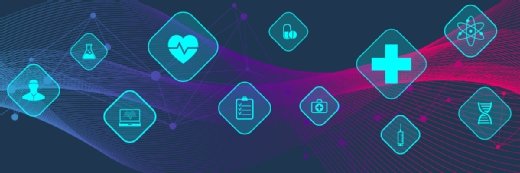
BAIVECTOR - stock.adobe.com
Machine Learning Predicts Cancer Risk in Liver Disease Patients
Machine learning can forecast which metabolic dysfunction-associated steatotic liver disease patients are likely to be diagnosed with liver cancer in the future.
A research team from UC Davis Health developed a machine learning (ML) tool to identify which patients are at increased risk of developing hepatocellular carcinoma (HCC), a common type of liver cancer.
The model leverages predictive analytics to provide risk assessments for patients with metabolic dysfunction-associated steatotic liver disease (MASLD).
“MASLD can lead to HCC, but the disease is quite sneaky, and it’s often unclear which patients face that risk,” indicated study co-author Aniket Alurwar, MS, clinical informatics specialist at the UC Davis Center for Precision Medicine and Data Sciences, in the news release. “It doesn’t make sense to biopsy every patient with MASLD, but if we can segment for risk, we can track those people more closely and perhaps catch HCC early.”
To improve HCC screening and mitigate risk, the researchers turned to ML. They began by testing nine preliminary open-source algorithms based on their ability to learn connections between clinical variables and use those connections to predict HCC in a cohort of 1,561 UC Davis Medical Center patients.
From these algorithms, five were shortlisted for further evaluation based on their high performance. These models were validated and compared to one another using data from a separate cohort of 686 patients at UC San Francisco Medical Center.
The Gradient Boosted Trees algorithm outperformed the others in terms of accuracy, specificity, and sensitivity. By incorporating the algorithm into a pilot model, the researchers could not only predict HCC risk, but also identify relevant risk factors for further assessment.
The analysis revealed that advanced liver fibrosis and cirrhosis – defined in terms of high Fibrosis-4 Index (FIB-4) scores – were among the most reliable predictors of HCC. In addition, four other risk factors associated with liver function were flagged: hypertension, high cholesterol, and abnormal levels of bilirubin and alkaline phosphatase (ALP).
These insights helped shed light on which patients may be at high risk for HCC but not eligible for screening under clinical guidelines. Patients with low FIB-4 but high cholesterol, hypertension, and bilirubin fall into this category, highlighting the model’s potential to improve screening.
“We got 92.12 [percent] accuracy when predicting which MASLD patients would develop HCC, which is very good for a pilot model,” Alurwar stated. “Patients with low FIB-4 are typically considered low risk and do not get referred for further assessment. By showing which of these ‘low risk’ patients could develop HCC, we can get them referred for liver biopsies or imaging.”
Moving forward, the research team hopes to enhance the model by incorporating additional data, like clinical notes, using natural language processing (NLP).
The researchers noted that a successful risk prediction model could eventually be integrated into electronic health records (EHRs) to help clinicians identify when a MASLD patient is at increased risk for HCC.
“We believe we can improve the algorithm by incorporating the clinical notes and perhaps other information,” said Alurwar. “Embedding this data should create an even more powerful model that we can then test to see how it performs.”
Advanced technologies like artificial intelligence (AI) and ML have significant potential in advancing risk stratification efforts for a variety of conditions, but these models require robust validation prior to deployment.
A research team from Mass General Brigham recently found that the Epic Risk of hospital-acquired acute kidney injury (HA-AKI) model is only moderately successful and exhibits several limitations.
The researchers noted that the tool’s predictive performance varied based on HA-AKI stage, with predictions for earlier stages being more accurate than those for later ones. The model was also more reliable when assessing lower-risk individuals, whereas it struggled to flag higher-risk patients.
Further, the research team indicated that deploying the model could result in high false-positive rates.
These findings underscore the importance of clinical validation for predictive analytics tools.