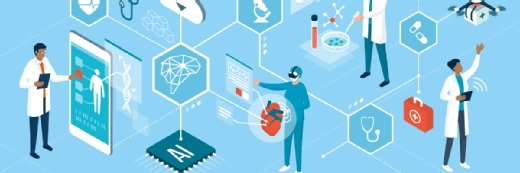
Getty Images
Artificial Intelligence Method Enables Data Mining from Pathology Reports
The Cancer Genome Atlas (TCGA)-Reports, a set of 10,000 machine-readable pathology reports, may bolster large language model applications in oncology research.
Cedars-Sinai researchers successfully utilized artificial intelligence (AI) to make pathology reports machine-readable, which could improve cancer patient recruitment in clinical trials.
The research team emphasized that cancer patients’ pathology data are valuable but difficult to obtain through traditional data mining approaches.
“Cancer is a complex disease, and rich information is contained in the notes that a pathologist makes when they review a patient’s cancer underneath the microscope,” said senior author of the study Nicholas Tatonetti, PhD, vice chair of Operations in the Department of Computational Biomedicine at Cedars-Sinai and associate director of Computational Oncology at Cedars-Sinai Cancer, in the news release. “But because these notes are in the form of scanned PDFs, the text they contain has been inaccessible to computers—until now.”
The researchers sought to create a machine-readable set of pathology reports using The Cancer Genome Atlas (TCGA), which contains pathology data from thousands of cancer patients across the United States.
“The pathology reports in the atlas are scanned in at all angles and in different formats from each of the institutions that provided them,” Tatonetti stated. “They’re messy and their scan quality is relatively poor—not unlike pathology forms you would find in patient records.”
To overcome these quality issues, the research team used AI and optical character recognition (OCR) techniques. This processing allowed each pathology report to be transformed into a machine-readable format.
Tatonetti indicated that doing so could enable researchers to train algorithms to extract relevant pathology information, which could be used to bolster clinical trial recruitment and studies investigating novel disease markers.
The resulting dataset, called TCGA-Reports, contains publicly available, machine-readable pathology reports from nearly 10,000 cancer patients. The format of each report is one commonly used by computer scientists and computational biologists to help make data more usable.
The research team also noted that the approach could be utilized to extract pathology information from datasets outside of TCGA.
“The true story of a patient’s condition, such as detailed information about their cancer and the effects of various therapies, is found in clinicians’ notes,” noted Cedars-Sinai Cancer director Dan Theodorescu, MD, PhD, the PHASE ONE Foundation Distinguished Chair and director at the Samuel Oschin Comprehensive Cancer Institute. “Tools that help us mine this information further our efforts to conduct translational studies that bring the promise of precision medicine to each of our patients.”
The research team is now looking at how to train models to extract cancer staging information from the dataset.
“Our model can extract that information when it is present in the notes, but it can also accurately infer the stage when it is not explicitly stated,” Tatonetti said. “For instance, the pathologist might make a note about a secondary lesion or [about] evaluating a sample of a breast cancer... These notes don’t include the word metastatic, but they do imply it.”
The researchers also aim to apply their method to Cedars-Sinai’s Molecular Twin Precision Oncology Platform, an AI-driven precision medicine tool to advance cancer research.
“AI enhancements to optical character recognition are the key to extracting a wealth of data from some of the most clinically relevant portions of patient records,” said Jason Moore, PhD, chair of the Department of Computational Biomedicine at Cedars-Sinai. “This data will fuel new studies by researchers across specialties, including research clinicians, clinical trial investigators and investigators working to improve tools that allow computers to interpret clinical language.”
Efforts to enhance precision medicine through the use of AI and other technologies continue as researchers seek to unlock the potential of clinical data.
Last month, a research team from University of Utah Health shared that it had developed a pharmacology platform to help shed light on drug dynamics in pediatric cancer patients.
Drug dynamics provide insights into the molecular, biochemical, and physiological impacts of medications, which can be affected by factors like a patient’s medical history and age.
However, data on drug dynamics for medications used to treat pediatric cancers is often lacking, which can put patients at risk.
The newly-developed platform helps address this by analyzing data from patients’ blood draws to flag signs of drug toxicity, investigate drug-chemotherapy interactions, and explore factors that influence drug movement.