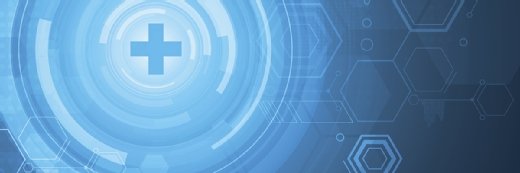
traffic_analyzer/DigitalVision V
Deep learning tool targets ‘black box’ AI problem in medical imaging
Novel deep learning approach designed to flag disease biomarkers in medical images can generate a ‘map’ to explain its diagnostic reasoning.
A research team from the University of Illinois Urbana-Champaign’s Beckman Institute for Advanced Science and Technology has developed a deep learning-based medical imaging approach designed to tackle the ‘black box’ problem in healthcare artificial intelligence (AI).
The ‘black box’ problem – a phenomenon in which an AI’s decision-making process remains hidden from users – is a significant challenge for the technology’s application in healthcare.
Neural networks, a type of deep learning that mimics the neural networks of the human brain to perform complex tasks, are valuable for differentiating between images and flagging imaging anomalies, but are also prone to the ‘black box’ phenomenon.
“[These models] get it right sometimes, maybe even most of the time, but it might not always be for the right reasons,” explained lead study author Sourya Sengupta, a graduate research assistant at the Beckman Institute, in the news release. “I’m sure everyone knows a child who saw a brown, four-legged dog once and then thought that every brown, four-legged animal was a dog.”
However, a child can explain their decision-making process, “but you can’t ask a deep neural network how it arrived at an answer,” Sengupta continued.
To address this, the researchers worked to build a medical imaging approach capable of explaining its reasoning.
"The idea is to help catch cancer and disease in its earliest stages — like an X on a map — and understand how the decision was made. Our model will help streamline that process and make it easier on doctors and patients alike,” said Sengupta.
The research team trained their model on over 20,000 medical images across three diagnostic tasks – simulated mammograms for tumor detection, optical coherence tomography (OCT)-based retinal images for macular degeneration detection and chest x-rays for cardiomegaly detection.
Following training, the tool’s performance was compared to that of existing black box models. The model matched its counterparts, achieving accuracy rates of 77.8 percent for mammograms, 99.1 percent for retinal images and 83 percent for chest x-rays.
The researchers indicated that this high performance is the result of utilizing a non-linear deep neural network.
“The question was: How can we leverage the concepts behind linear models to make non-linear deep neural networks also interpretable like this?” said principal investigator Mark Anastasio, PhD, a Beckman Institute researcher and the Donald Biggar Willet Professor and Head of the Illinois Department of Bioengineering. “This work is a classic example of how fundamental ideas can lead to some novel solutions for state-of-the-art AI models.”
This neural network architecture allows the model to quickly analyze images and generate a value between zero and one to denote the presence of an anomaly like a tumor. A value less than 0.5 indicates that the image is presumably absent of tumors, whereas a value higher than 0.5 suggests that a tumor is likely present.
These values can help a clinician decide which images may warrant further investigation, but most models do not provide any explanation of how they generated each value.
The new tool, however, does.
The model is designed to provide both a value and what’s known as an equivalency map (E-map). The E-map is a transformed version of the original medical image that highlights medically interesting regions of the image that may be useful for predicting anomalies and assigns each region a score. These scores combined yield the original value assigned to the image, showing users which areas of an image the tool deemed important to arrive at its decision.
“For example, if the total sum is 1, and you have three values represented on the map — .5, .3, and .2 — a doctor can see exactly which areas on the map contributed more to that conclusion and investigate those more fully,” Sengupta stated.
“The result is a more transparent, trustable system between doctor and patient,” he continued.
The researchers emphasized that this more transparent approach can help clinicians ‘double-check’ the model’s performance and help patients better understand the AI’s role in the diagnostic process.
In the future, the research team hopes to expand the tool to detect anomalies in other parts of the body.
“I am excited about our tool’s direct benefit to society, not only in terms of improving disease diagnoses, but also improving trust and transparency between doctors and patients,” Anastasio said.
Others are also designing approaches to tackle the ‘black box’ problem in healthcare AI.
Last month, researchers from Stanford University and the University of Washington shared that they have developed an auditing framework to provide insights into the decision-making process of health AI.
The research team underscored that ‘black box’ AI tools in the healthcare space are a significant barrier to adoption and that increased explainability is necessary.
To foster this, the framework combines human expertise with generative AI to evaluate classifier algorithms, which are used to categorize data inputs. The analysis revealed that five dermatology classifiers use both undesirable features – such as skin texture and color – alongside those utilized by clinicians – like pigmentation patterns.
The findings could help researchers determine whether their AI models are relying on spurious correlations in the data that could lead to flawed outputs.