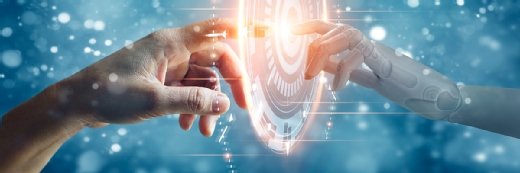
Getty Images
Synthetic tumor data enhances training for cancer detection AI
An artificial intelligence model trained solely on synthetic liver tumor data performed on par with models trained using images of real tumors.
A Johns Hopkins University-led team of researchers has created a method to generate large datasets of synthetic liver tumor computed tomography (CT) scans, which could aid in the training of cancer detection algorithms.
The generation of artificial, automatically annotated tumor images could help address an ongoing scarcity of high-quality data used to train AI to identify early-stage cancer. Flagging tumors on medical scans is a time-consuming process that often requires interpreting pathology reports and waiting for biopsy confirmations, making large-scale datasets difficult to curate.
The researchers indicated that currently, there are roughly 200 publicly available annotated CT scans of liver tumors, and this limited number is not sufficient for AI training and testing.
To tackle this issue, the research team designed a four-step framework for generating high-quality synthetic tumors: choosing locations for artificial tumors to avoid collisions with surrounding blood vessels, adding random “noise” to the data to simulate the irregular textures of real tumors, generating shapes that mimic variations in real tumors and simulating changes in tumor appearance resulting from their tendency to push on their surroundings.
The resulting synthetic tumors were difficult to distinguish from their real counterparts, passing the Visual Turing test.
From there, the research team trained an AI algorithm using only this synthetic tumor data. The model successfully outperformed similar approaches and achieved comparable results to AI trained on real tumor data.
"Our method is exciting because, to date, no existing work utilizing synthetic tumors alone has achieved a similar or even comparable performance to AI trained on real tumors," said Qixin Hu, a researcher from the Huazhong University of Science and Technology, in the news release. "Furthermore, our method can automatically generate numerous examples of small—or even tiny—synthetic tumors, which has the potential to improve the success rate of AI-powered tumor detection. Detecting small tumors is critical for identifying the early stages of cancer."
The researchers emphasized that their method can be used to generate datasets and train AI for other types of cancer, as well. Currently, the team is exploring advanced image processing approaches to generate synthetic liver, pancreas, kidney and other tumors.
"The ultimate goal of this project is to synthesize all kinds of abnormalities—including tumors—in the human body to be used for AI development so that radiologists don't have to spend their valuable time conducting manual annotations," stated Hu. "This study makes a significant step towards that goal."
Research efforts like these speak to ongoing debates about the pros and cons of synthetic healthcare data. While many argue that synthetic data is a privacy-preserving alternative to the use of real-world patient information for research and analytics, others posit that issues like data quality limit the utility of the approach.