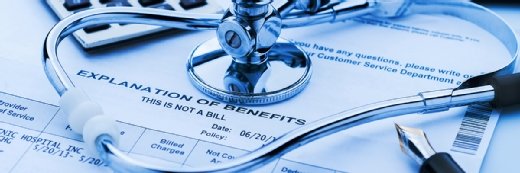
Christian Delbert - stock.adobe.
Machine learning tool accurately predicts spine surgery outcomes
A machine learning approach using wearable data, EHRs and ecological momentary assessment information outperformed existing spine surgery outcome prediction tools.
Researchers from Washington University in St. Louis have developed a machine learning (ML) approach to predict recovery outcomes following lumbar spine surgery using data from wearables and other sources.
The research team underscored that predicting post-surgical outcomes is key to improving clinical decision-making and personalizing treatments following orthopedic surgery. Spine surgery outcomes can vary significantly depending on a patient’s structural disease, along with their physical and mental health characteristics, including excessive stress leading up to surgery or physiological problems.
The researchers further noted that existing models to forecast spine surgery outcomes typically rely on patient-reported outcome measures (PROMs), which are limited in their ability to capture insights into a patient's long-term condition prior to surgery.
Many predictive tools are also focused on singular surgical outcomes, but recovery is multi-dimensional, consisting of various distinctive, but related, outcomes, such as physical function, pain interference and quality of recovery.
The advent of wearable devices and smartphones presents opportunities to more effectively capture longitudinal patient data outside of the clinical setting, and the researchers’ previous work in this area demonstrated that PROMs and wearable data improved post-surgical outcome predictions.
This research correlated Fitbit data with ecological momentary assessment (EMA) data. This information, which captured participants’ emotional and social states, was captured using smartphones. To improve this approach, the researchers turned to ML.
“We combine wearables, EMA and clinical records to capture a broad range of information about the patients, from physical activities to subjective reports of pain and mental health, and to clinical characteristics,” said Chenyang Lu, PhD, the Fullgraf Professor at the university’s McKelvey School of Engineering, in the news release.
In doing so, the ML is set to provide a more accurate “big picture” view of the factors that impact surgical recovery and how they relate to each other. The research team explained that the approach, known as “Multi-Modal Multi-Task Learning,” weighs the relatedness of the outcomes to inform its predictions.
This analysis yields a predicted change score for physical function and pain interference, which can be used to inform treatment. When tested, the ML model outperformed standard predictive approaches.
“By predicting the outcomes before the surgery, we can help establish some expectations and help with early interventions and identify high risk factors,” stated first author of the study Ziqi Xu, a PhD student in Lu’s lab.
The researchers plan to continue fine-tuning their approach, enabling it to make better predictions and help clinicians “understand what types of factors can potentially be modified to improve longer-term outcomes,” according to Jacob Greenberg, MD, an assistant professor of neurosurgery at the School of Medicine.
These efforts reflect the growing interest in how predictive analytics can enhance healthcare, with AI and ML increasingly being applied to improve risk stratification and patient outcomes.