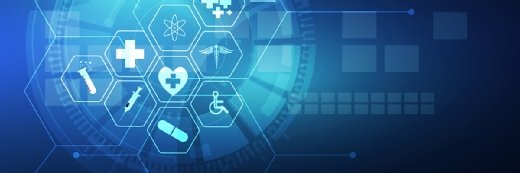
Panuwat Sikham/istock via Getty
Machine learning-driven liquid biopsy identifies lung cancer risk
A machine learning-based approach could flag individuals at high risk for lung cancer by analyzing DNA fragments found in blood, which may improve screening uptake.
Researchers from the Johns Hopkins Kimmel Cancer Center have developed a machine learning (ML)-based liquid biopsy approach that identifies lung cancer risk via analyses of DNA fragments in the blood.
The American Cancer Society indicates that lung cancer is the leading cause of cancer death in the US, accounting for roughly one-fifth of total cancer deaths annually. The organization estimates that in 2024 alone, there will be 234,580 new cases of lung cancer and 125,070 lung cancer-related deaths.
To improve outcomes, the US Preventive Services Task Force (USPSTF) recommends that individuals aged 50 to 80 with relevant smoking history be screened for lung cancer yearly with low-dose computed tomography (LDCT). Annual screening helps detect cancer early, allowing patients to pursue care when the disease is most treatable.
However, the research team underscored that only a small fraction of this group pursues lung cancer screening, often because of concerns over the low doses of radiation exposure from the scan or difficulties with making and arriving at a screening appointment. Low screening uptake is a significant contributor to adverse outcomes and preventable deaths, making overcoming barriers to screening a priority for oncologists and researchers.
To tackle these challenges, the research team sought to develop a simpler screening approach.
The team indicated that the presence of lung cancer is associated with changes in genome-wide cell-free DNA (cfDNA) fragmentation profiles – or fragmentomes – which can be detected in blood samples. In normal cells, DNA is packaged neatly, but the DNA in cancer cells is less consistently organized.
Upon the death of either cell type, DNA fragments are released into the bloodstream. Based on how these fragmentomes are organized, researchers can gain insights into whether an individual is at risk of developing lung cancer.
To develop their screening tool, the researchers trained ML to flag DNA fragment patterns associated with lung cancer. The model was trained using blood sample data from 576 participants with or without lung cancer and validated in a held-out cohort of 382 individuals.
When tested, the tool achieved a negative predictive value of 99.8%, indicating that it would only miss two in 1,000 true cases of lung cancer.
Using computer modeling, the research team demonstrated that if their method could boost lung cancer screening to 50% within the next five years, it could potentially quadruple the number of detected lung cancers and increase the proportion of cases detected early by roughly 10%. In this simulated scenario, these results would translate to 14,000 lung cancer deaths prevented.
“We have a simple blood test that could be done in a doctor’s office that would tell patients whether they have potential signs of lung cancer and should get a follow-up CT scan,” said corresponding author of the study Victor E. Velculescu, MD, PhD, professor of oncology and co-director of the Cancer Genetics and Epigenetics program at the Johns Hopkins Kimmel Cancer Center, in the news release.
“The test is inexpensive and could be done at a very large scale,” Velculescu continued. “We believe it will make lung cancer screening more accessible and help many more people get screened. This will lead to more cancers being detected and treated early.”