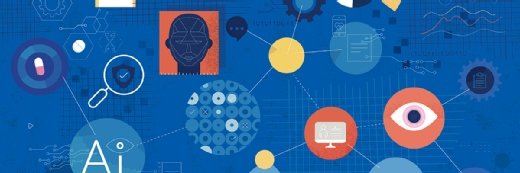
DrAfter123/DigitalVision Vectors
Yale Researchers Develop ML Tool for Personalized Blood Pressure Management
Researchers at Yale University have developed a machine learning-based clinical decision support tool to personalize recommendations for blood pressure management treatment goals.
A team of Yale University researchers has developed a machine learning (ML)-based clinical decision support tool to personalize recommendations for pursuing intensive or standard blood pressure treatment goals among individuals with and without diabetes.
The tool, described in a study published earlier this week in The Lancet Digital Health, is designed to facilitate shared decision-making between providers and patients with hypertension through a data-driven approach. Hypertension is defined as a sustained blood pressure greater than 140/90 mm Hg and is a leading cause of heart disease and mortality.
In the study, the researchers noted that while lowering blood pressure is crucial to reducing these risks, the level to which blood pressure should be lowered has been contested. This is particularly so for patients with Type 2 diabetes because clinical trials evaluating aggressive blood pressure control for these patients have been inconclusive, they stated.
To address this, the research team sought to develop an ML-based model to personalize blood pressure management treatment goals for patients with and without diabetes.
The researchers began by gathering data from two randomized clinical trials: Systolic Blood Pressure Intervention Trial (SPRINT), which did not include patients with diabetes, and Action to Control Cardiovascular Risk in Diabetes Blood Pressure (ACCORD BP), which included only patients with diabetes. In both trials, patients were randomized to an intensive or routine systolic blood pressure goal of 120 mm Hg or 140 mm Hg.
The researchers chose these trials because SPRINT demonstrated the value of lowering blood pressure, while the ACCORD BP trial showed that intensive blood pressure treatment was ineffective. The team leveraged data from SPRINT to identify 59 variables — including kidney function, smoking, and statin or aspirin use — to develop an ML model, known as PREssure Control In hypertension (PRECISION), designed to identify features of patients that benefitted the most from intensively lowering blood pressure.
The researchers then assessed PRECISION’s value when applied to ACCORD BP. They found that the tool could identify patients with diabetes who benefitted from aggressive blood pressure management compared to standard treatment.
“Identifying the appropriate blood pressure targets and treatment course for patients with hypertension and diabetes can be challenging,” said Rohan Khera, MD, assistant professor at the Yale School of Medicine and director of the Cardiovascular Data Science (CarDS) Lab, in the press release. “Here, we used machine learning to enhance inference from two landmark clinical trials in assessing a personalized cardiovascular benefit of intensive blood pressure control. The key finding is that the benefit profile derived in patients without diabetes seems to define those with diabetes that benefit from such a treatment strategy.”
The researchers stated that these findings indicate that PRECISION may provide reliable, practical insights to inform decision-making regarding intensive versus standard systolic blood pressure management among patients with diabetes. However, they further noted that additional testing in diverse patient populations is needed to better understand how various factors contribute to the risks and benefits of an intensive blood pressure-lowering strategy.
This research comes amid concerns about blood pressure control and rising chronic disease prevalence.
Research published in the American Heart Association’s Hypertension last year evaluating hypertension control in the US from 2009 to 2018 found that rates of blood pressure control in the US are falling, indicating a larger population health issue. Using data from more than 4,000 adults between 2015 and 2018, the research team discovered that the rate of control had fallen by 7.5 percent, representing a loss of 10 years in hypertension control progress in just four years.