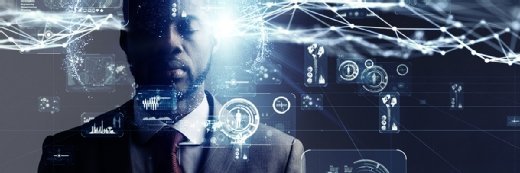
Getty Images
ML Model Accurately Predicts Need for Massive Transfusion During Surgery
A recent study described the success of a machine-learning model in predicting the need for massive transfusion during surgery, allowing for early intervention among high-risk patients.
Published in JAMA Network Open, a recent study described how adding preoperative data and intraoperative hemodynamic monitoring data to a machine learning (ML)-based prediction model led to accurate real-time predictions of the need for massive transfusion during surgery.
Many healthcare providers are using predictive analytics to improve the timeliness and quality of treatment while reining in costs. For example, a study published in December found that a disease management outreach program supported by predictive analytics effectively reduced medical spending among chronic heart failure patients.
In various clinical settings, massive hemorrhage is the leading cause of death. When managing this condition, acute bleeding control and supplementation for intravascular volume and blood component deficiencies are required, according to the study.
Researchers noted the importance of massive transfusion in preventing complications amid uncontrolled intraoperative hemorrhage. Further, because of the time required to prepare blood product for massive transfusions and the need for additional medical personnel, they noted that the ability to predict the need for massive transfusion early is critical for necessary hemorrhage management.
Thus, researchers conducted a study that involved the inclusion of preoperative data and intraoperative hemodynamic monitoring data into a real-time prediction model for massive transfusion.
They gathered data from patients who had surgery that involved invasive blood pressure monitoring at Seoul National University Hospital (SNUH) between 2016 and 2019, as well as at Boramae Medical Center (BMC) from 2020 to 2021. Both hospitals are located in South Korea.
For the study, researchers defined massive transfusion as a transfusion of at least three units of red blood cells within an hour.
Upon review of 12,986 patients from SNUH, researchers found that 416 received massive transfusions during their operation. When comparing the real-time prediction model, which was developed with preoperative and intraoperative parameters, and the preoperative prediction model, researchers found that the former significantly outperformed the latter. The real-time prediction model also displayed "excellent performance" in the external validation data set, the study noted.
Based on these findings, researchers concluded that the performance of the real-time prediction model for massive transfusion during surgery showed high accuracy, enabling early intervention for high-risk patients.
But the researchers also noted several study limitations, including that the prediction model was created using a retrospective database and that the model performance was validated using data from patients at various times and locations.
In general, predictive tool use in healthcare has grown.
A study from November, for example, described how a risk-scoring tool could help predict dementia risk, allowing clinical teams to take action and begin treatment in a timely manner.
Using data from a prospective cohort study, researchers performed multiple analyses of patient data, including individual analyses of time endpoints at the first dementia diagnosis during follow-ups. The researchers also identified risk factors for dementia, including socioeconomic adversity and sleep phenotypes.
The risk score model achieved almost 100 percent prediction accuracy for 13-year dementia risk in both men and women.
Based on the results, researchers concluded that the risk score tool could be used for individual prediction of dementia risk, helping individuals in determining their potential risk profile.