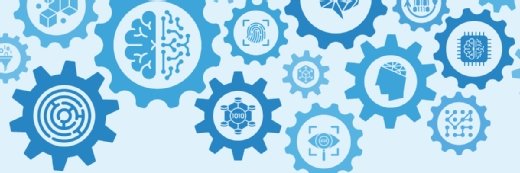
Getty Images
Machine-Learning Models Can Accurately Predict Hypertension Risk
A study finds that machine-learning algorithms can predict hypertension on par with conventional risk prediction models.
A study published this week in Scientific Reports comparing hypertension incidence prediction models found little difference in performance between machine-learning (ML) models and conventional approaches, indicating that ML-based risk prediction models can accurately predict hypertension.
Hypertension creates significant chronic disease management and population health burdens, making identifying those at risk of developing the condition a major priority in clinical research, the study authors noted. Various risk prediction models have been developed for hypertension, but there is a growing interest in ML approaches, indicating the need to evaluate their performance and potential clinical applications.
This study aimed to compare ML algorithms’ predictive performance with a conventional Cox proportional hazards (PH) regression model's performance in assessing hypertension risk prediction in a survival setting, for which there is a lack of research, the authors explained.
The study analyzed 24 candidate features from 18,322 participants in the Alberta’s Tomorrow Project (ATP) dataset to develop different prediction models, including the conventional Cox PH model and five ML models: penalized regression Ridge, Lasso, Elastic Net (EN), random survival forest (RSF), and gradient boosting (GB).
The top features were selected using five feature selection methods. Each model’s predictive performance was assessed using the concordance index (C-index), a statistical metric common in survival analysis that describes how well a predicted risk score describes an observed sequence of events.
Some major predictive features included the age of the study participants, body mass index, waist-hip ratio, diastolic blood pressure, systolic blood pressure, total physical activity time, and total sitting time, alongside demographic data such as sex, ethnicity, marital status, and education level.
Overall, the performances of the models were similar across the board, with each model achieving significant predictive accuracy. Average C-indexes were 0.78, 0.78, 0.78, 0.76, 0.76, and 0.77 for the Ridge, Lasso, EN, RSF, GB, and Cox PH models, respectively.
The researchers also found that during the median 5.8-year follow-up, 3.41 percent of participants newly developed hypertension, with most of the relevant features varying significantly between those who developed hypertension and those who did not, including age, sex, body mass index, waist-hip ratio, diastolic blood pressure, systolic blood pressure, total household income, highest education level completed, diabetes, cardiovascular disease, smoking status, working status, total sleep time, total sitting time, vegetable and fruit consumption, and job schedule.
Conversely, the other features, including marital status, residence, ethnicity, depression, family history of hypertension, alcohol consumption, total physical activity time, and physical activity, were not significantly different between the two groups.
These findings indicate that the ML models can perform similarly to conventional hypertension risk prediction models and achieve good prediction accuracy. But the authors warned that comparison of algorithms in clinical research could be subject to various pitfalls, including biases toward newer algorithms.
These led the researchers to take a more neutral comparison approach, and they noted that future comparison studies should also aim for a neutral approach.