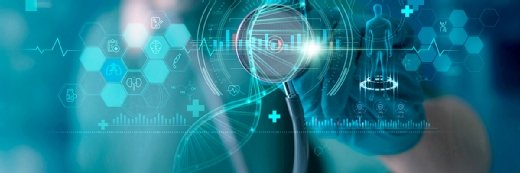
ipopba/istock via Getty Images
Machine Learning Model Estimates Optimal Treatment Timing for Sepsis
New research shows that a machine learning model can help clinicians decide when to administer antibiotics to patients with a suspected case of sepsis.
Researchers from Ohio State University (OSU) have developed a machine learning (ML) model that can accurately estimate optimal treatment timing for sepsis cases and support clinical decision-making, according to a study published last week in Nature Machine Intelligence.
Sepsis is a life-threatening condition that can rapidly lead to tissue damage, organ failure, and death without timely treatment. However, the symptoms of sepsis—such as breathing problems, high heart rate, low blood pressure, and fever—can resemble those of multiple other conditions, making diagnosing sepsis a challenge.
The press release discussing the study’s findings also highlighted that federal guidelines for sepsis treatment mandate quick treatment using broad-spectrum antibiotics, but this approach requires that clinicians take action on a sepsis diagnosis before results confirming infection can come back from the lab.
To support clinical decision-making in this situation, the research team developed an ML model to estimate the optimal time to administer antibiotics to a patient with a suspected case of sepsis.
The model was trained on a publicly available critical care database known as MIMIC-III. Using these data, the tool was tasked with making treatment timing estimates using indicators of illness severity and type of infection, like lab results, vital signs, and risk-related demographic information.
The model also incorporated the Sequential Organ Failure Assessment (SOFA) score, which helps assess how an ICU patient’s organ systems are performing at regular intervals based on results from six lab tests.
The tool was tested on different portions of MIMIC-III and an additional dataset from the Amsterdam University Medical Centers Database (AmsterdamUMCdb). The outcomes measured during model testing and validation were patient survival at 30 and 60 days following sepsis treatment.
Outcomes in patients whose actual treatment matched the model’s recommended treatment timeline were compared to outcomes for patients whose actual treatment had differed from what the model would have recommended based illness severity and infection type indicators.
Overall, the researchers found that the model’s recommendations helped improve sepsis outcomes.
“We showed that when the actual treatment and artificial intelligence agree, we have a lower mortality rate. If they don’t agree, the mortality rate can be as high as 25%,” explained senior author Ping Zhang, PhD, assistant professor of computer science and engineering and biomedical informatics at OSU, in the press release.
Despite this success, the researchers emphasized the need for clinician input when making any treatment decision, especially with a condition as serious as sepsis. Because the symptoms of sepsis are shared with other conditions, not every patient who meets the criteria will have sepsis, so the risks of administering antibiotics must be considered carefully by the care team.
“What this paper starts to get at is, can we use information available to the clinicians, sometimes at the forefront and sometimes not, to say: Things are changing in a way that suggests the patient will benefit from antibiotics,” said study co-author Katherine Buck, MD, assistant professor of emergency medicine in the College of Medicine and director of the Geriatric Emergency Department at Ohio State Wexner Medical Center. “A decision-support tool could tell clinicians if it matches what we’re already thinking or prompt us to ask ourselves what we’re missing. Hopefully, with time, all the electronic health record data we have will reveal signals – and from there it’s a matter of figuring out how to use them and how to get that to clinicians.”
From here, the research team indicated that more research in this area is needed.
“Our paper is the first to use [artificial intelligence] to pursue an antibiotic recommendation for sepsis, using real-world data to help clinical decision making,” said Zhang. “Any research like this needs clinical validation – this is phase one for retrospective data analysis, and phase two will involve human-AI collaboration for better patient care.”