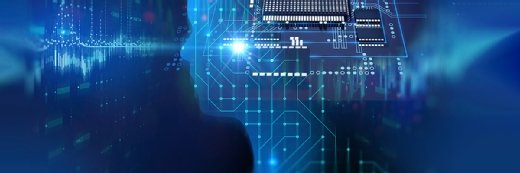
Getty Images
Machine-Learning Algorithm Enhances Stroke Diagnosis
New research describes how a machine-learning tool can use patient data to diagnose a stroke with 83 percent accuracy.
Published in the Journal of Medical Internet Research, findings from Florida International University’s College of Business (FIU Business) indicate that a new machine-learning (ML) algorithm could leverage hospital and social determinants of health (SDoH) data to improve the speed and accuracy of stroke diagnoses.
According to the Centers for Disease Control and Prevention (CDC), over 795,000 people in the US have a stroke annually, 610,000 of which are first-time strokes.
An FIU news release also noted that stroke is a prevalent and misdiagnosed condition. Timeliness in stroke detection is key, as those who receive treatment within one hour following symptom onset generally experience favorable outcomes.
Considering this, researchers from FIU Business created an ML algorithm that considers demographics such as age, race, and underlying health conditions of potential stroke patients, along with SDoH. With this information, the tool can provide valuable insight to help diagnose strokes.
“As we add more data it’s learning data,” said Min Chen, PhD, associate professor of information systems and business analytics at FIU Business and one of the researchers, in a press release. “Our algorithm can incorporate a lot of variables to analyze and interpret complex patterns, which will allow emergency department care teams to make better and faster decisions. This data-driven approach will help make it easier to identify stroke patients and ensure that they get the care they need in a timely manner.”
The data researchers used to create the ML algorithm consisted of emergency department and hospitalization records from Florida hospitals between 2012 and 2014, along with SDoH information from the American Community Survey.
The ML algorithm is used after a patient arrives at a hospital with stroke-like symptoms. A computer-based screening tool that leverages the algorithm reviews patient data to predict stroke risk. The emergency department team receives a notification if a patient is high-risk.
The researchers analyzed the tool's efficacy using data from 143,203 patients. Of these, 73 percent had a stroke. The study shows that the algorithm was able to diagnose the strokes with 83 percent accuracy.
Researchers noted that the tool might be able to fill in gaps in stroke care. Generally, ML methods for stroke detection use clinical notes and diagnostic imaging results. Although these tools are useful, they may not always be available within hospitals in underserved communities.
As artificial intelligence (AI) use continues to grow, researchers continue to create new tools to enhance the detection of various conditions.
In March, for example, research from Rutgers, the State of New Jersey, described how AI and ML could examine genes within DNA to assist clinicians in predicting cardiovascular diseases.
Following an analysis of healthy patients with cardiovascular disease, researchers used ML models to establish an association between genes and disease manifestations. This process allowed them to define a group of genes that correlated with cardiovascular disease and assisted them in confirming that age, gender, and race were contributing factors.
Another study from April 2022 found that an ML algorithm helped high-risk patients who missed a colonoscopy by providing them with information about the type of treatment needed.
After collecting more than 25,000 patients whom researchers defined as overdue for colorectal cancer screening, they used an ML algorithm to determine the patients at risk for developing cancer. The tool did this by considering factors such as age, gender, and outpatient blood count.