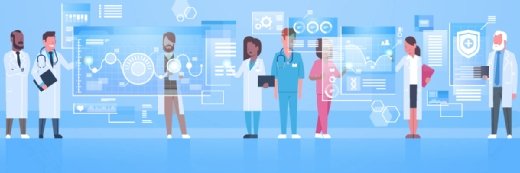
mast3r - stock.adobe.com
Machine Learning Identifies Four Autism Subtypes
Researchers at Weill Cornell Medicine have identified four different subtypes of autism spectrum disorder using machine learning.
Researchers at Well Cornell Medicine have identified four subtypes of autism spectrum disorder (ASD) linked to distinct molecular pathways in the brain using machine learning (ML).
The findings were unveiled in a recent study published in Nature Neuroscience, which sought to investigate whether molecular mechanisms could explain individual differences in ASD.
“Like many neuropsychiatric diagnoses, individuals with autism spectrum disorder experience many different types of difficulties with social interaction, communication and repetitive behaviors. Scientists believe there are probably many different types of autism spectrum disorder that might require different treatments, but there is no consensus on how to define them,” said co-senior author Conor Liston, PhD, MD, an associate professor of psychiatry and of neuroscience in the Feil Family Brain and Mind Research Institute at Weill Cornell Medicine, in the press release. “Our work highlights a new approach to discovering subtypes of autism that might one day lead to new approaches for diagnosis and treatment.”
To investigate ASD subtypes, the researchers built on previous work by Liston and colleagues that used ML to identify four biologically distinct subtypes of depression, subgroups that later research revealed respond differently to various therapies.
Using this approach, the research team sought to explore whether similar subgroups exist for patients with ASD and whether different gene pathways are responsible for variations among these subgroups.
To do this, the researchers integrated neuroimaging data with gene expression data and proteomics, which allowed the team to better understand how risk variants interact in the autism subgroups, as ASD is a highly heritable condition that is associated with hundreds of different genes.
“One of the barriers to developing therapies for autism is that the diagnostic criteria are broad, and thus apply to a large and phenotypically diverse group of people with different underlying biological mechanisms,” explained lead author Amanda Buch, PhD, a postdoctoral associate of neuroscience in psychiatry at Weill Cornell Medicine. “To personalize therapies for individuals with autism, it will be important to understand and target this biological diversity. It is hard to identify the optimal therapy when everyone is treated as being the same, when they are each unique.”
The researchers analyzed neuroimaging data from 299 people with autism and 907 neurotypical people. From this, they found that certain patterns of brain connections were linked with behavioral traits in people with ASD, such as verbal ability, social affect, and repetitive or stereotypic behaviors.
These patterns helped identify four autism subgroups, which were also found upon analysis of a separate dataset.
Two of the groups had above-average verbal intelligence. Of these, one group demonstrated severe deficits in social communication but less repetitive behaviors, while the other demonstrated more repetitive behaviors and less social impairment.
The subgroup with more social impairment had hyperactive connections between the parts of the brain that process visual information and help identify the most salient incoming information. Conversely, these connections were weaker in the subgroup with more repetitive behaviors.
The other two groups had both severe social impairments and repetitive behaviors, but individuals in these subgroups also had verbal abilities at opposite ends of the spectrum.
Despite some similar behaviors and traits, the researchers found distinct brain connection patterns within these subgroups.
Moving forward, the research team will build on these findings and study the four subgroups and potential subgroup-targeted treatments in mice. The researchers are also collaborating with other teams that have access to large human datasets to further validate and refine the ML approach leveraged in this study.