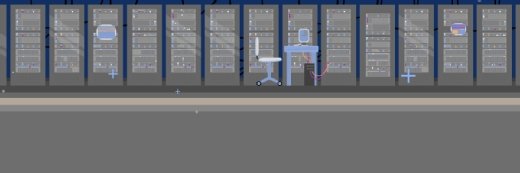
Getty Images
AI Can Detect Race When Clinicians Cannot, Increasing Risk of Bias
New research shows that artificial intelligence can accurately predict race using various medical images, but clinicians cannot do the same, indicating significant risks for AI use in healthcare.
Researchers have found that artificial intelligence (AI) models can accurately detect self-reported racial identity using medical images, but the mechanisms they use to do so are unclear to researchers, indicating that models already in use may accidentally exacerbate health disparities.
According to a study published in The Lancet Digital Health, research has shown that the ability of AI to detect a person's race using medical images is well documented, but there are no clear image-based variables or proxies to race present in the images that would allow clinicians to do the same. Therefore, the researchers sought to investigate what mechanisms AI models use to make their predictions.
The researchers began by evaluating the performance of AI models in predicting race using medical images alone. They gathered images from both public and private datasets to assess the models’ performances on data that varied significantly in terms of image type and the environment in which the images were taken, in case either of these variables impacted the models’ predictions.
In addition, the researchers used regression models to assess possible anatomic and phenotypic variables that could be used to detect race. The researchers evaluated the ability of each of these variables to detect race on their own. They were then incorporated into the AI models to see how they impacted prediction performance.
Finally, the researchers analyzed what effect corrupting or altering the medical images could have on the AI models’ predictions of race to investigate what image-specific factors may contribute to model performance. The researchers applied low- and high-pass filters to transform the images, some of which were altered to the point that the researchers stated they wouldn’t have been able to identify what the images were had they not already known.
The researchers found that the AI models all demonstrated a high ability to detect race across all image types and datasets, indicating that image type and environment were not significant factors for racial identity prediction.
The researchers also discovered that they could not identify any image-based variables or proxies for race that could explain the high performance of the models.
Body-mass index (BMI), tissue density, breast density, age, disease labels, bone density, sex, and a combination of these factors were all evaluated on their ability to influence the racial identity predictions of the models, but none showed significant results. This indicates that these anatomic and phenotypic variables did not strongly correlate with the models’ ability to classify race.
Distorting the images achieved mixed results, with high levels of image degradation from the low-pass filter resulting in significantly poorer model performance. High performance was generally maintained with the addition of a high-pass filter. There was also no evidence that any specific anatomical regions or body segments contributed to model performance.
These findings, combined with the knowledge that clinical radiologists cannot identify race based on medical images, have significant implications for deploying AI medical imaging models. The study results indicate that the models do not rely on local idiosyncrasies in how imaging studies are conducted. This suggests that the actual factors that AI models use are more general.
While some of the factors identified in the experiment may play a small role, other factors need to be discovered and studied to accurately understand how an AI model detects race, the researchers noted. If the factors that enable models to predict race accurately are both very general and not obvious to humans, AI models that are currently being used could accidentally be perpetuating racial disparities in healthcare.