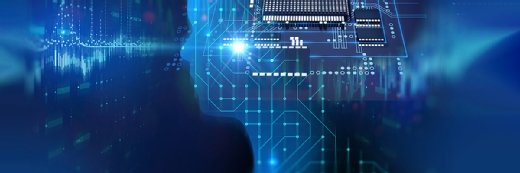
Getty Images
AI Framework Cuts Time, Effort Needed to Predict Critical Care Outcomes
Scientists from the University of Washington developed an artificial intelligence framework that can reduce the time, effort, and resources required to predict patient outcomes in critical care settings.
Researchers from the University of Washington’s School of Medicine and the Paul G. Allen School of Computer Science & Engineering have developed an artificial intelligence (AI) framework that can reduce the costs associated with predicting patient outcomes in critical care settings, such as the time and effort taken to gather data.
Predictive analytics models can enable care providers to assess various patient risk factors and help them improve patient outcomes. However, research published in Nature Biomedical Engineering found that the large amount of time and effort linked to creating predictive models prevents providers from leveraging AI in emergency services and critical care settings.
“With sufficient data and the right parameters, AI models perform quite well when asked to predict clinical outcomes. But in this case, ‘sufficient data’ often translates as an impractical number of patient features to collect in many care settings,” stated Gabriel Erion, an MD-PhD student in the university’s Medical Scientist Training Program (MSTP) and co-author of the study, in the press release. “The cost, in terms of the time and effort required to collect that volume and variety of data, would be much too high in an ambulance or intensive care unit, for example, where every second counts and responders need to prioritize patient care.”
To reduce these costs, the researchers developed the Cost-Aware Artificial Intelligence (CoAI) model. CoAI is a framework designed to dramatically reduce the time, effort, and resources required to predict patient outcomes and inform clinical decision-making without sacrificing accuracy.
CoAI was trained using three large datasets containing prediction labels, patient features, expert annotations of feature costs, and a budget representing total acceptable cost, which serves to reduce the number of clinical risk factors that need to be collected in real time. The researchers then calculated quantitative measures of the predictive powers of each feature in the dataset. This allows CoAI to calculate the importance of a group of features relative to their cost.
From there, CoAI recommends which subset of features would enable the most accurate prediction of patient risk within a specified budget. The researchers reported that first responders only have 50 seconds to score patient risk factors when performing life-saving medical interventions, meaning that CoAI needed to be able to work under this constraint, unlike other models. Under these circumstances, CoAI prioritizes a subset of features to gather while achieving the same or better results than other, less cost-aware models.
CoAI also has a significant advantage over similar cost-aware AI models.
“A notable difference between CoAI and other approaches is its robustness to ‘cost shift,’ wherein features become more or less expensive after the model has been trained. Since our framework decouples feature selection from training, CoAI continues to perform well even when this shift occurs,” noted Joseph Janizek, another MD-PhD student at the MSTP and co-author of the study, in the press release. “And because it’s model-agnostic, CoAI can be used to adapt any predictive AI system to be cost-aware, enabling accurate predictions at [a] lower cost within a wide variety of settings.”
In their study, the researchers found that CoAI lowered the cost of data acquisition by about 90 percent for predictions related to trauma response for patients en route to the hospital and the in-hospital mortality risk of critical care patients in the ICU.