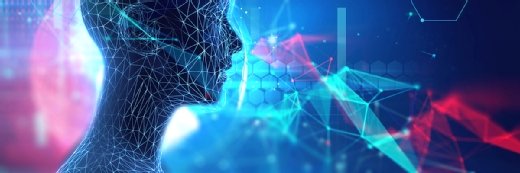
Getty Images
In-Person Screening, Machine Learning Can Help Predict Suicide Risk
A new study shows that combining face-to-face screening with an EHR-based machine-learning model can help clinicians accurately predict and classify suicide risk in adults.
Researchers found that suicide risk predictions for adult patients significantly improve when using an approach that combines in-person screening with an EHR-based machine-learning (ML) model.
The study, published in JAMA Network Open earlier this month, notes that 800,000 people worldwide die by suicide each year, which worsened during the COVID-19 pandemic.
While suicidal behavior is increasing in the US, the rate at which patients proactively disclose suicidal thoughts and behavior is not. The researchers also point out that mental health diagnoses are often absent from the medical records of those who die from suicide. This combination of factors makes better risk identification essential for improved outreach and prevention, they noted.
Previous research has shown that face-to-face universal screening and artificial intelligence (AI) prediction models have both achieved high performance in predicting suicide risk on their own, so the researchers decided to study the success of an approach utilizing both.
The researchers studied an observational cohort of 83,394 patients at Vanderbilt University Medical Center (VUMC) from June 2019 to September 2020. During that time, these patients had 120,398 encounters with the medical center in the inpatient, ambulatory surgical, and emergency department settings. All patient data from these encounters was pulled from the Vanderbilt Research Derivative, VUMC’s clinical research repository.
VUMC implemented universal suicide risk screening in June 2018 using the Columbia Suicide Severity Rating Scale (C-SSRS), a standardized assessment of suicidal ideation and behavior. Since this data was already being routinely collected at VUMC, the researchers chose to use it for the face-to-face screening aspect of their study.
For their prediction model, they used the Vanderbilt Suicide Attempt and Ideation Likelihood (VSAIL) model, which has generated real-time suicide risk predictions across the VUMC system since June 2019. The model uses historical EHR data to predict suicide risk, including demographic data like age, sex, and race, diagnostic codes, medication data, past healthcare utilization, and area deprivation index by patient zip code, which is a multidimensional evaluation of a region’s socioeconomic conditions and how they may relate to health outcomes.
The researchers extracted both C-SSRS response data and the corresponding VSAIL risk scores for each patient screened. Then, they compared the ensemble model, which combined the C-SSRS and VSAIL’s prediction results, to each method individually.
To predict suicide risk, the researchers measured suicidal ideation (SI) and suicide attempt (SA), defined using self-injurious thoughts and behaviors (SITBs) coded in ICD-9-CM and ICD-10-CM, that occurred within seven, 30, 60, 90, and 180 days after the discharge date of each documented visit during the study period.
Overall, SI was documented in 614 (0.51 percent), 1486 (1.23 percent), 2036 (1.69 percent), 2433 (2.02 percent), and 3126 (2.60 percent) cases at seven, 30, 60, 90, and 180 days, respectively. SA was documented in 84 (0.07 percent), 205 (0.17 percent), 272 (0.23 percent), 356 (0.30 percent), and 514 (0.43 percent) cases at seven, 30, 60, 90, and 180 days, respectively.
At all time intervals, the ensemble model outperformed both the C-SSRS and the VSAIL models when used individually.
These findings indicate that combining pre-trained ML models with structured clinician assessment to create ensemble prediction systems for suicide risk could significantly improve outcomes. However, the researchers note that their study will need to be replicated and validated to achieve greater generalizability.