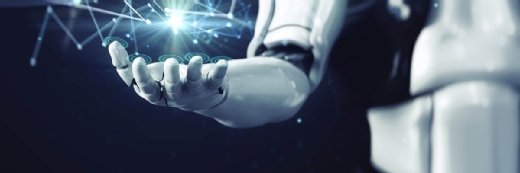
Getty Images
Machine learning tool flags undiagnosed immunodeficiency disease
A machine learning model can effectively sift through EHR data to rank patients' likelihood of having common variable immunodeficiency disease.
A research team from University of California, Los Angeles (UCLA) Health have developed a machine learning model capable of identifying undiagnosed immune disorders by analyzing EHR data, according to a recent study published in Science Translational Medicine.
The researchers focused specifically on common variable immunodeficiency (CVID) disease, a set of rare immune disorders.
“Patients who have rare diseases may face prolonged delays in diagnosis and treatment, resulting in unnecessary testing, progressive illness, psychological stresses, and financial burdens,” said Manish Butte, MD, PhD, a professor in pediatrics, human genetics and microbiology/immunology at UCLA, in a news release. “Machine learning and other artificial intelligence methods are making their way into health care. Using these tools, we developed an approach to speed the diagnosis of undiagnosed patients by identifying patterns in their electronic health records that resemble those of patients who are known to have the disorders.”
The research team indicated that CVID is a common type of inborn error of immunity (IEI) – a group of approximately 500 inherited conditions that cause immune system dysfunction. CVID is estimated to impact one in every 25,000 people, and the condition is characterized by antibody deficiencies and impaired immune response.
However, the researchers further noted that patients with CVID can go undiagnosed for significant periods because of the condition’s rarity, varied symptom presentation and the overlap between CVID symptoms and those of more common diseases.
Identifying CVID is especially challenging because the condition is typically driven by single-gene changes, but these changes can occur in one of over 60 genes. This lack of a single causal mechanism for CVID has resulted in there being no genetic test to diagnose it.
“The clinical presentation of rare immune phenotypes such as CVID intersects with many medical specialties. Patients may be seen in ear, nose and throat clinics for sinus infections. They may be treated in pulmonology clinics for pneumonias. This fragmentation of care across multiple specialists leads to long delays in diagnosis and treatment. It’s impossible to teach all these busy specialists about immune deficiencies with the hopes that, even if they could recognize which patients have an underlying immune defect, they’ll refer these patients to us. We had to find a better way to find these patients,” Butte explained.
To that end, the research team set out to design a tool to flag potential signs that a patient may have CVID. Particularly, the researchers were interested in whether the wealth of information held in EHRs could reveal phenotypic patterns signifying high likelihood of the condition.
The model, PheNet, was designed to infer disease signatures from the records of patients known to have CVID and patterns of the condition identified in medical literature. Using this data, the tool can then generate a numerical score that ranks patients based on their likelihood of having CVID.
Patients with high scores are described by the research team as “hiding in the medical system,” and would be candidates for referral to immunology specialists.
The researchers tested PheNet by applying the tool to a cohort of UCLA health patients and conducting a blinded chart review of the most at-risk individuals. Of the 100 patients flagged by the model, 74 percent were deemed likely to have CVID.
From there, the team validated PheNet using 6 million patient records from the University of California Data Warehouse and Vanderbilt Medical Center.
The research team emphasized that this early success holds promise for helping patients receive an earlier diagnosis.
“Our own patients report experiencing years to decades of symptoms before they were referred to our immunology clinic,” Butte stated. “With PheNet, dozens of patients could have been diagnosed one to four years earlier than they were, and by bringing patients to care years earlier, we should be able to reduce their costs and improve their health outcomes.”
Now, the researchers are spearheading efforts to have patients flagged by the model seen by immunology specialists on the University of California campuses in San Diego, Irvine, Davis and San Francisco.
“We show that artificial intelligence algorithms such as PheNet can offer clinical benefits by expediting the diagnosis of CVID, and we expect this to apply to other rare diseases, as well,” noted Bogdan Pasaniuc, PhD, a professor of computational medicine, human genetics and pathology and laboratory medicine at UCLA David Geffen School of Medicine. “Our implementation across all five University of California medical centers is already making an impact. We are now improving the precision of our approach to better identify CVID while expanding to other diseases. We will also plan to teach the system to read medical notes to glean even more information about patients and their illnesses.”