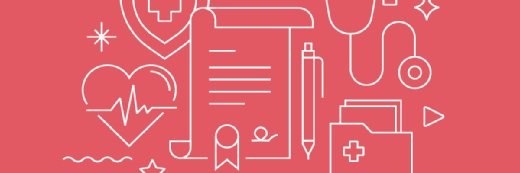
cnythzl/DigitalVision Vectors vi
Deep learning framework predicts drug effectiveness, treatment outcomes
New deep learning approach outperformed seven existing models and recommended the same stroke treatments as four randomized clinical trials.
Researchers from Ohio State University (OSU) have developed a deep learning-based framework designed to emulate randomized clinical trials (RCTs) and estimate treatment effects in stroke patients, according to a recent study published in Patterns.
The research team emphasized that treatment effect estimation (TEE) is key to understanding the impacts of medical interventions on patient outcomes. RCTs are the gold standard for identifying these impacts, but conducting them is time- and resource-intensive.
Methods like AI and machine learning have been proposed to help address these challenges, but the use of real-world clinical information for TEE hinders these efforts, as there is often a scarcity of labeled data. To overcome this, the researchers sought to investigate whether a pre-trained foundation model could predict the impact of various treatments on stroke prevention in patients with heart disease.
The deep learning approach, CaUsal tReatment Effect estimation (CURE), was trained using data points from MarketScan Commercial Claims and Encounters from 2012 to 2017, including information from 3 million patients.
This pre-training approach makes the model more general, allowing for fine-tuning based on the use case. In this case, the tool focused on stroke risk and was tasked with estimating the causal effects of each treatment to identify which would work best for each patient.
The model’s performance was compared to seven existing models and four RCTs. CURE outperformed the seven models and provided the same recommendations as the RCTs.
“No existing algorithm can do this work,” said senior author Ping Zhang, PhD, associate professor of computer science and engineering and biomedical informatics at OSU, in a press release. “Quantitatively, our method increased performance by 7% to 8% over other methods. And the comparison showed other methods could infer similar results, but they can’t produce a result exactly like a randomized clinical trial. Our method can.”
While the researchers do not intend to replace RCTs with their framework, they hope that the model could speed up the RCT process, saving time and money.
“Our model could be an acceleratory module that could help first identify a small group of candidate drugs that are effective to treat a disease, allowing clinicians to conduct randomized clinical trials on a limited scale with just a few drugs,” said first author Ruoqi Liu, a computer science and engineering PhD student in Zhang’s lab.
The researchers further indicated that the model has potential applicability across other drugs and diseases.
“We can pre-train the model on large-scale datasets without limiting it to any treatments. Then we fine-tune the pre-trained model on task-specific small-scale datasets so that the model can adapt quickly to different downstream tasks,” Liu explained.
The approach also benefits from the use of biomedical knowledge graphs, which help fill in gaps in patients’ records.
“We also proposed KG-TREAT, a knowledge-enhanced foundation model, to synergize the patient data with the knowledge graphs to have the model better understand the patient data,” said Liu, who authored recent work on knowledge graph applications in this area.
The incorporation of knowledge graphs enhanced CURE’s performance, and the research team noted that someday, the framework could provide valuable clinical decision support.
“This model is better than a crystal ball: Based on big data and foundation model AI, we can have reasonable confidence to be able to say what treatment strategy is better,” said Zhang. “We want to put physicians in the driver’s seat to see whether this is something that can be helpful for them when they’re making critical decisions.”