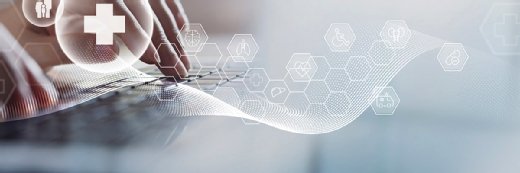
tippapatt - stock.adobe.com
Machine learning predicts risk of OUD treatment discontinuation
New machine learning framework reveals that almost 15% of opioid use disorder patients discontinued recommended buprenorphine treatment prematurely.
A research team from the University of Florida (UF) has developed a machine learning framework to identify which opioid use disorder (OUD) patients are at high risk of prematurely discontinuing buprenorphine treatment.
The researchers indicated that buprenorphine is one of three FDA-approved treatments for OUD that has been shown to effectively treat both addiction and pain. However, premature treatment discontinuation can undermine its effectiveness, increasing patients’ risk of overdose and death.
Identifying at-risk OUD patients could help healthcare providers build patient support systems and improve treatment retention rates, but research investigating the predictive value of known treatment discontinuation risk factors is limited.
“We know that sticking with a buprenorphine treatment plan is beneficial. Premature discontinuation could increase the risk of hospitalization, drug overdose and most importantly, mortality,” explained Md Mahmudul Hasan, PhD, an assistant professor in the UF College of Pharmacy department of pharmaceutical outcomes and policy with a joint appointment in the UF Warrington College of Business department of information systems and operations management, in the press release.
“If we can use AI to predict which patients are at a higher risk of this behavior, clinical practitioners can get to the root cause, make more informed decisions and design more targeted interventions for those patients,” he continued.
To that end, the research team developed a machine learning-based clinical decision support framework to predict buprenorphine discontinuation within 12 months of treatment initiation.
The analysis pulled MarketScan commercial claims data from 2018 to 2021 to build a study cohort of insured individuals with OUD between the ages of 18 and 64. These patients initiated buprenorphine treatment from July 2018 to December 2020 and had no prescriptions for the drug six months prior to the study period.
From there, the researchers used the data to measure buprenorphine prescription discontinuation gaps of 30 or more days during the first year after initiating treatment. Multiple predictive models were developed for the analysis: decision tree classifier, logistic regression, Adaboost, random forest, XGBoost and random forest-XGBoost ensemble.
Using these models, the research team assessed discontinuation risk at the time of treatment initiation and one and three months afterward. The cohort was then stratified into risk subgroups.
Over 30,000 patients initiated buprenorphine, and of these, nearly 15 percent did not complete their yearlong recommended treatment. Further, 46 percent of the cohort discontinued treatment within the first three months.
Multiple factors were found to be associated with treatment discontinuation, including age, gender, use of stimulants or antipsychotics, early treatment adherence and how many days’ supply of buprenorphine a patient was initially prescribed.
“Younger patients are at a higher risk of prematurely stopping treatment, along with those with a history of stimulant use, including nicotine,” Hasan said. “We also found patients with lower buprenorphine adherence at the early treatment stage are more at risk of premature treatment discontinuation.”
Patients facing treatment access barriers, like living in a rural area with no treatment facilities close by, were also at increased risk of buprenorphine discontinuation.
The researchers emphasized that the use of predictive technologies like theirs could help flag those who might struggle with buprenorphine treatment, enabling clinicians to better understand which patients are likely to respond well while developing strategies for others who may need additional support to improve treatment adherence.
“Primary care physicians are already overburdened and overworked, and they have limited resources. A tool like this that can reliably predict which patient will be high-risk could be helpful,” Hasan said. “Within a short time and without increasing their workload, health care providers can identify the interventions needed for each patient, allowing them to best allocate their limited resources.”
The research comes as US overdose deaths have dipped for the first time in five years, a potentially positive sign as healthcare organizations struggle to combat the effects of the ongoing opioid crisis.