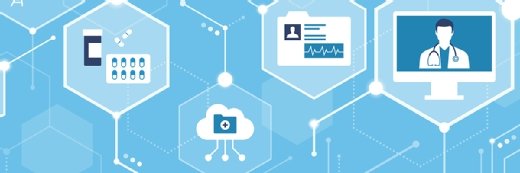
elenabs/istock via getty images
Machine Learning Model Predicts Individual Risk for Multiple Myeloma
A new computational model can forecast an individual’s personalized multiple myeloma prognosis based on their tumor genomics and treatment regimen.
Researchers have developed an individual risk prediction model for multiple myeloma, which could significantly enhance prognosis and treatment, according to a study published this week in the Journal of Clinical Oncology.
The model, called individualized risk in newly diagnosed multiple myeloma (IRMMa), is designed to improve upon existing prognostic tools by incorporating tumor biology. Assessing tumor genomics is becoming more prevalent for various cancers, but the research team underscored that doing so for multiple myeloma is critical, as the disease is highly variable.
By utilizing a more precise approach, researchers can gain new insights into the cancers they’re investigating. Over the course of IRMMa’s development, the research team successfully identified and classified 12 distinct subtypes of multiple myeloma.
“The future of the field will have to be focused on precision medicine,” emphasized senior author C. Ola Landgren, MD, PhD, chief of the Division of Myeloma and director of the Sylvester Myeloma Institute at Sylvester Comprehensive Cancer Center at the University of Miami Miller School of Medicine, in a news release. “There’s no other way forward.”
The original method for assessing multiple myeloma, developed in the 1970s, relied on staging solid tumors. With the treatments that were available at the time, this approach was relatively accurate, as it was based on the amount of cancer present.
However, the researchers underscored that the oncology landscape has advanced significantly since then, with various new treatment approaches available to patients. To select the right treatment for their patients, clinicians must be able to accurately evaluate each therapeutic choice in the context of a patient's prognosis.
Doing so can be a challenge, as tools to predict patient outcomes are limited. The research team further noted that while some of the newer prediction models incorporate tumor genomics, there are more recent findings around genomic risk factors that have remained largely unexplored in such a tool.
The researchers also indicated that current prediction tools often rely on population-level averages to help group patients into categories of low, standard, and high risk. Forecasting risk in this manner, they explained, does not consider individualized risk and how it can be impacted by treatments.
“Our model is based on the idea of predicting the risk of the individual patient rather than that of the group,” said Francesco Maura, MD, an assistant professor at Sylvester and first author on the study.
To build IRMMa, the researchers leveraged clinical, treatment, and genetic data from 2,000 patients newly diagnosed with multiple myeloma from Sylvester Comprehensive Cancer Center, Memorial Sloan Kettering Cancer Center, NYU Langone Health, Moffitt Cancer Center, and Heidelberg University Hospital.
Using patient DNA sequences, the research team identified 90 “driver genes” — genes with mutations in the cancer cells that seem to drive tumor growth. From there, the researchers matched patients’ treatment outcomes to their individual tumor genetic sequences.
The model uses machine learning to identify patterns in these data, which are then used to make risk predictions. IRMMa is designed to be flexible, in the event that a patient’s prognosis changes following treatment. As new therapies become available, data from patients receiving those treatments can also be added to help improve the model’s predictions.
Currently, the model is aimed at researchers, but the research team hopes to enhance the model by adding more patient data.
“This model can only grow with the help of the research community,” Maura stated. “The next challenge is to keep feeding it with the right datasets so at a certain point it will be usable for clinical purposes.”
Using advanced technologies like artificial intelligence (AI) and machine learning to improve risk prediction is becoming more prevalent in healthcare.
This week, researchers shared that they have successfully developed a risk score to predict intensive care unit (ICU) admission and ICU survival among community-dwelling older adults.
Existing models typically rely on clinical data from emergency departments and cannot identify outpatients who may be admitted to the ICU in the future. As utilization of critical care services grows because of aging populations living longer, models to predict future ICU admission, survivorship, and mortality are needed.
Thus, the research team developed a population-level risk model. The model achieved high performance in discriminating between patients who died versus those who remained alive without ICU admission and between ICU survivors versus those alive without ICU admission.