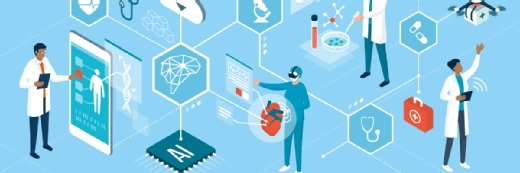
Getty Images
Predictive Models for Personalized Medicine Have Limited Generalizability
New study reveals that the predictive algorithms used to tailor individual treatments have limited effectiveness and may not generalize well across populations.
Yale researchers have found that the predictive algorithms used to forecast treatment efficacy and guide personalized medicine efforts have limited effectiveness when generalized to patient cohorts outside of those in their training data, according to a recent study published in Science.
Much of the hype around artificial intelligence (AI) technologies in healthcare centers on their potential for large-scale data mining and analysis, which could facilitate improved clinical predictions and personalized care for patients.
However, for AI to open up these opportunities, algorithms must be both accurate and generalizable across patient populations, which remains a challenge for model developers and clinical researchers.
The research team underscored that the scarcity and cost of high-quality clinical data have led some to hope that if a model is successful in one or two clinical settings or datasets, then it may be successfully generalized across others.
However, the researchers sought to investigate the validity of this assertion by evaluating how well machine learning (ML) models performed across five independent clinical trials for schizophrenia.
Specifically, the researchers tested the models’ ability to predict patient outcomes in the context of antipsychotic medication for schizophrenia.
The research team found that the models were capable of predicting patient outcomes with high accuracy, but only within the trial for which each was developed. When models were applied to trials they were not developed for, however, their performance dipped significantly.
“The algorithms almost always worked first time around,” explained Adam Chekroud, PhD, an adjunct assistant professor of psychiatry at Yale School of Medicine and corresponding author of the paper, in a news release. “But when we tested them on patients from other trials the predictive value was no greater than chance.”
The challenge, he emphasized, lies in the size of the datasets. Clinical trials are costly and time-intensive, meaning that most studies enroll less than 1,000 patients.
Many of the algorithms leveraged by clinical researchers are designed to be utilized on much larger datasets, and applying them to smaller datasets can lead to overfitting – a phenomenon in which a model has learned response patterns that are specific to a particular dataset, preventing it from performing well on new data.
“The reality is, we need to be thinking about developing algorithms in the same way we think about developing new drugs,” Chekroud noted. “We need to see algorithms working in multiple different times or contexts before we can really believe them.”
“In theory, clinical trials should be the easiest place for algorithms to work. But if algorithms can’t generalize from one clinical trial to another, it will be even more challenging to use them in clinical practice,’’ stated co-author John Krystal, MD, the Robert L. McNeil, Jr. Professor of Translational Research and professor of psychiatry, neuroscience, and psychology at Yale School of Medicine.
The researchers noted that the study highlighted the challenges of utilizing predictive models for personalized medicine across specialties.
“Although the study dealt with schizophrenia trials, it raises difficult questions for personalized medicine more broadly, and its application in cardiovascular disease and cancer,” said Philip Corlett, PhD, an associate professor of psychiatry at Yale and co-author of the study.
The research team indicated that improved data sharing by researchers and data banking by large healthcare providers could help enhance the accuracy and generalizability of future AI models.
“This study really challenges the status quo of algorithm development and raises the bar for the future,” said Chekroud. “Right now, I would say we need to see algorithms working in at least two different settings before we can really get excited about it.”
“I’m still optimistic,” he continued, “but as medical researchers we have some serious things to figure out.”
This study underscores some of the major hurdles that precision medicine efforts have yet to overcome, but research in those areas shows no sign of slowing.
Last week, a research team from the Sylvester Comprehensive Cancer Center at the University of Miami Miller School of Medicine shared that they have successfully developed a first-of-its-kind individual risk prediction model for multiple myeloma.
The model improves upon current prognostic tools for the disease by assessing tumor biology and treatment regimen. By taking a precision medicine approach to tumor genomics, the researchers were able to accurately predict an individual’s personalized multiple myeloma prognosis.
During their study, the researchers also used these insights to identify and classify 12 distinct subtypes of multiple myeloma.