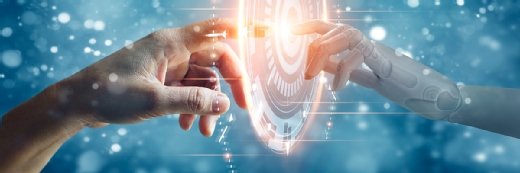
Getty Images
ML Model Predicts Complications Following Cardiovascular Interventions
A machine learning model outperformed standard tools for predicting death and other serious complications in patients undergoing percutaneous coronary intervention.
A research team from the University of Michigan has developed a machine learning tool capable of accurately predicting death, major bleeding events, and the need for blood transfusion in patients undergoing angioplasty and stent placement, according to a study published this month in the European Heart Journal.
Percutaneous coronary intervention (PCI), formerly known as angioplasty with stenting, is a minimally invasive procedure that uses a catheter to place a stent in coronary arteries to help treat blockages. While PCI is considered less risky than more invasive procedures, it can carry significant risks that vary widely.
Effectively stratifying PCI risk remains a challenge for clinicians. To tackle this, the researchers developed an artificial intelligence (AI) algorithm to help guide treatment planning.
“The risks for patients undergoing percutaneous coronary intervention vary greatly depending on the individual patient, and both patients and clinicians have historically both over and underestimated the harms associated with PCI,” explained David E. Hamilton, MD, a cardiology-critical care fellow at Michigan Medicine who led the study, in a news release.
“Precise risk prediction is critical to treatment selection and the shared decision-making process. Our tool can recognize a wide array of outcomes after PCI and can be used by care providers and patients together to decide the best course of treatment,” he continued.
To develop the tool, the research team gathered data from a cohort of 107,793 patients who underwent PCI procedures at 48 hospitals in Michigan between April 1, 2018 and December 31, 2021 in the Blue Cross Blue Shield of Michigan Cardiovascular Consortium (BMC2) registry. These data were then divided into training and validation cohorts.
Using these data, the researchers identified 23 features that were most relevant to predict post-PCI outcomes like mortality, new nephropathy requiring dialysis, acute kidney injury, stroke, major bleeding, and need for blood transfusion.
To help personalize the model’s risk stratification, the research team incorporated patient feedback from 66 individuals regarding the shared decision-making process prior to PCI.
“We combined the predictive model with patient feedback from the PCI Patient Advisory Council to transform machine learning into this patient-centered, individualized risk prediction tool,” said senior author Hitinder Gurm, MBBS, interim chief medical officer at U-M Health.
The tool was externally validated using the Cardiac Care Outcomes Assessment Program database, which contained 56,583 procedures from 33 hospitals in Washington.
Outcome rates varied across the cohort: the mortality rate was 1.85 percent, acute kidney injury was 2.51 percent, new-onset dialysis was 0.44 percent, stroke was 0.41 percent, major bleeding was 0.89 percent, and transfusion was 2.41 percent.
Overall, the model achieved high performance – measured in terms of area under the receiver-operating characteristic curve (AUC) – across all outcomes. The tool achieved an AUC of 0.930 for mortality; 0.893 for acute kidney injury; 0.951 for new-onset dialysis; 0.751 for stroke; 0.917 for transfusion; and 0.997 for major bleeding.
These findings underscore the potential of a model that utilizes patient feedback and pre-procedural risk factors to predict post-PCI outcomes.
The tool has been released as a free computer and smartphone application for others to explore.
“In the age of widespread smartphones and electronic medical records, this computerized risk score could be integrated into electronic health systems and made easy to use at the bedside,” Gurm noted. “It would not only help relay complex information to the provider quickly, but it could also be used to enhance patient education on the risks related to PCI.”
This is the latest research seeking to evaluate how AI and machine learning can advance cardiovascular care.
Last week, a research team from Children’s National Hospital detailed the development of a deep learning system to detect latent rheumatic heart disease (RHD) in children.
RHD, caused by heart valve damage from bacterial infections, is a major cause of death and disability in those under 25, particularly in low- and middle-income countries.
Early detection of the condition is possible, and necessary for effective treatment, but access to cardiologists in the most-impacted areas is often limited.
To address this, the researchers used AI to develop an ultrasound interpretation algorithm that accurately identifies RHD features, including heart size, without the need for a cardiologist. The system aims to improve RHD detection and treatment globally, potentially improving identification and treatment in low-resource areas.