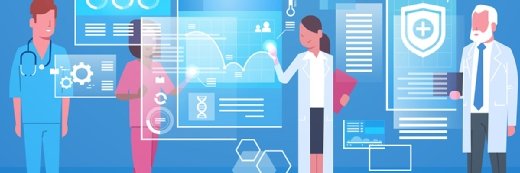
gmast3r/istock via Getty Images
Machine Learning, ‘Liquid Biopsy’ to Bolster Early Cancer Detection
Researchers leveraging a machine learning-based “fragmentomics” approach may be able to detect cancer sooner using smaller blood samples than traditional methods.
Researchers from City of Hope and its precision medicine research organization, the Translational Genomics Research Institute (TGen), have developed a machine learning (ML) approach that could facilitate the early detection of cancer using significantly less blood than current diagnostic tools, according to a study published recently in Science Translational Medicine.
The research team underscored that a significant portion of patients will survive for at least five years after their initial diagnosis if the cancer is caught at stage 1, whereas this number drops steadily when patients are diagnosed at later stages.
“A huge body of evidence shows that cancer caught at later stages kills people. This new technology gets us closer to a world where people will receive a blood test annually to detect cancer earlier when it is more treatable and possibly curable,” said Cristian Tomasetti, PhD, corresponding author of the study and director of City of Hope’s Center for Cancer Prevention and Early Detection, in a press release.
The tool is designed to identify and classify cancers based on material present in a patient's bloodstream. Alu elements – highly repetitive deoxyribonucleic acid (DNA) sequences found throughout the human genome – can be associated with cancerous cells, but their application as a biomarker has been somewhat limited.
However, when cells die, they break down and shed cell-free DNA (cfDNA) into the bloodstream. Signs of cancer can appear in cfDNA, as normal cells and cancer cells are known to break down differently.
“Fragmentomics,” an approach to identify cancer cfDNA fragments – which are believed to be more common in repetitive regions of the genome, like areas with a high volume of Alu elements – may allow researchers to detect cancer.
Identifying differences in fragmentation patterns within normal and cancer cfDNA would typically require research teams to look for one displaced letter out of billions present in a patient’s genetic code, meaning that a larger biopsy sample is often required for analysis.
Since the fragmentomics approach focuses on cfDNA present in specific regions of the genome, it would require approximately eight times less blood than traditional whole genome sequencing.
“Our technique is more practical for clinical applications as it requires smaller quantities of genomic material from a blood sample,” explained Kamel Lahouel, PhD, co-first author of the study and an assistant professor in TGen’s Integrated Cancer Genomics Division. “Continued success in this area and clinical validation opens the door for the introduction of routine tests to detect cancer in its earliest stages.”
Using fragmentomics data from patient blood samples, the research team developed Alu Profile Learning Using Sequencing (A-Plus), an ML model to profile Alu elements and identify the presence of cancer.
A-Plus was applied to 7,657 samples from 5,980 patients. Of these, 2,073 patients had solid cancer, and a large portion of this sample was diagnosed with early-stage disease without metastatic lesions. The rest of the participants were without cancer.
The tool successfully flagged half of the cancers present across 11 cancer types, with false positives appearing in one of every 100 cases.
The researchers are set to open a clinical trial in the coming months to compare their fragmentomics-based ‘liquid biopsy’ approach with standard-of-care in a cohort of patients between the ages of 65 and 75. In doing so, the research team aims to establish the tool’s efficacy in detecting earlier stages of cancer, which are more treatable.
This research is the latest effort to use artificial intelligence (AI) to improve biomarker-based diagnostics.
Last week, researchers from Cedars-Sinai shared that they used an AI-based precision medicine tool to design a highly accurate blood test for pancreatic cancer outcome prediction.
The model, known as the Molecular Twin Precision Oncology Platform (MOVER), uses genetic and molecular information to predict disease survival. In tests, the tool accurately forecasted pancreatic cancer survival in 87 percent of patients.
Using insights gained from the model’s development, the research team was able to create a blood-protein test that outperformed the only Food and Drug Administration-approved pancreatic cancer test.