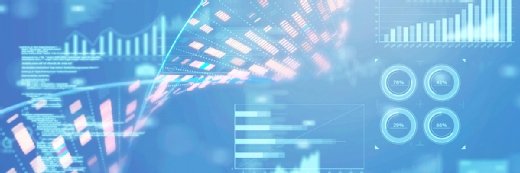
Yuichiro Chino/Moment via Getty
NYC Health Dept. Expands Work to Address Racism in Clinical Algorithms
The Coalition to End Racism in Clinical Algorithms, which examines race adjustment in clinical decision support tools, will expand its focus to include hypertension.
The Coalition to End Racism in Clinical Algorithms (CERCA), a New York City Health Department-led effort to investigate the use of race-based medical algorithms, is expanding its work to include clinical decision support tools used in hypertension management.
In the two years since its inception, the coalition has focused its efforts on race adjustment in clinical algorithms for glomerular filtration rate (eGFR) for kidney function; spirometry/pulmonary function testing (PFT); and vaginal birth after Cesarean section (VBAC).
“Medicine has not been immune to the legacy of racism, and it has infected the way we care for minoritized communities across this city and this nation for too long,” said health commissioner Ashwin Vasan, MD, PhD, in the press release. “Confronting that history and promoting the health of all New Yorkers is no small feat and compels us to look at the kind of care that people receive. Improving care, promoting equity and removing harmful practices and building trust in public health makes our entire city a healthier place.”
Using $2.9 million in funding from the Doris Duke Foundation, CERCA will continue its work in these focus areas while expanding to address the use of hypertension algorithms.
Hypertension is a significant cause of disease burden and mortality in the United States, particularly for Black New Yorkers.
Medical algorithms can play an important role in guiding clinical decision-making to treat patients with chronic conditions like hypertension. However, some algorithms rely on race adjustment – also called “race norming” or “race correction” – to assess a patient’s health.
However, the incorporation of race into clinical algorithms has come under fire in recent years, with research indicating that the approach is fraught with the potential to perpetuate bias and cause patient harm.
A well-documented example of such harm can be found in the use of race-based algorithms to assess kidney function. The Organ Procurement & Transplantation Network (OPTN) unanimously approved a proposal to require race-neutral eGFR calculations, noting that the inclusion of race in these algorithms can cause them to overestimate Black patients’ kidney function. Doing so can make Black patients appear to be less sick than their white counterparts.
Race-based prescribing for hypertension is not uncommon, which can lead to health disparities.
Nine health systems and other organizations have participated in CERCA to end race modifier use in the clinical algorithms within its three focus areas; evaluate the effect of algorithms that do not incorporate race on patient outcomes and health equity; and develop patient engagement initiatives to support patients whose care may have been influenced by race modifiers.
The coalition will continue this work, in addition to broadening its scope to include race-based hypertension prescribing. Doing so is necessary to develop race-conscious approaches to hypertension management, the press release indicates.
“The use of race-based algorithms to make clinical decisions is a practice that is behind the times,” stated Michelle Morse, MD, MPH, chief medical officer and deputy commissioner of the NYC Health Department. “Decades of research has shown that race is not biology. CERCA’s impact and ongoing work is critical to transforming our healthcare system to be fairer and more equitable in the twenty-first century.”
The role of race in clinical algorithms in perpetuating racism and bias has been a significant concern for providers as artificial intelligence (AI) and other clinical decision support tools have become more common.
Researchers writing in The Lancet Digital Health last year found that AI models can detect self-reported race using medical images, even when clinicians cannot, raising concerns about the tools’ ability to unintentionally exacerbate existing health disparities.
The research team emphasized that an AI’s ability to predict patient race via medical images isn’t a new phenomenon, but the variables or proxies that models use to come to these conclusions remain unclear. The researchers sought to evaluate what mechanisms AI tools use to identify patient race.
They observed that image type and environment, alongside anatomic and phenotypic variables, were not significant factors in the models’ predictions of racial identity, underscoring the need for further research.