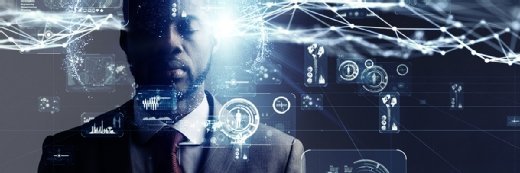
Getty Images
Large Language Models May Improve Clinician Diagnostic Performance
Probabilistic recommendations from AI chatbots may improve human diagnostic performance through collective intelligence, potentially curbing overtreatment.
Researchers from Beth Israel Deaconess Medical Center (BIDMC) found that the large language model (LLM) ChatGPT-4 outperforms clinicians in some instances of estimating the probabilities of diagnoses before and after laboratory testing.
The research team indicated that clinicians often perform poorly when tasked with estimating the pretest and posttest probabilities of disease, which can lead to overtreatment. Thus, the team chose to evaluate whether an LLM could assist in this process.
“Humans struggle with probabilistic reasoning, the practice of making decisions based on calculating odds,” explained Adam Rodman, MD, the study’s corresponding author and an internal medicine physician in the department of Medicine at BIDMC. “Probabilistic reasoning is one of several components of making a diagnosis, which is an incredibly complex process that uses a variety of different cognitive strategies. We chose to evaluate probabilistic reasoning in isolation because it is a well-known area where humans could use support.”
To assess ChatGPT-4, the researchers leveraged a previously published national survey in which 553 practitioners performed probabilistic reasoning on a set of five medical cases. Each case, along with a prompt designed to ensure that the chatbot would generate a specific pretest and posttest probability, were fed to the model.
From there, each case and its associated prompt were run in ChatGPT-4’s application programming interface (API) one hundred times to create a distribution of outputs.
The model then estimated the likelihood of a given diagnosis based on patients’ presentation. Then, when provided test results for each case – chest radiography for pneumonia, urine culture for urinary tract infection, stress test for coronary artery disease, and mammography for breast cancer – the chatbot updated its responses.
When its performance was compared to that of the clinicians in the survey, ChatGPT demonstrated less error in its pretest and posttest probability estimates following a negative test result. For positive test results, however, its performance was mixed: ChatGPT-4 was more accurate than its human counterparts in two cases, similarly accurate in two cases, and less accurate in the final case.
The researchers noted that the model’s performance in the face of negative test results could provide enhanced clinical decision support.
“Humans sometimes feel the risk is higher than it is after a negative test result, which can lead to overtreatment, more tests and too many medications,” said Rodman.
Moving forward, the research team is interested in how the incorporation of LLMs into clinical care could improve clinicians’ diagnostic performance.
“LLMs can’t access the outside world – they aren’t calculating probabilities the way that epidemiologists, or even poker players, do. What they're doing has a lot more in common with how humans make spot probabilistic decisions,” Rodman stated. “But that’s what is exciting. Even if imperfect, their ease of use and ability to be integrated into clinical workflows could theoretically make humans make better decisions… Future research into collective human and artificial intelligence [AI] is sorely needed.”
As LLMs continue to show promise across a plethora of applications, stakeholders are increasingly interested in how these tools could be utilized in healthcare.
Last week, Google launched MedLM, a suite of foundation models designed to help healthcare organizations meet their needs through generative AI.
The two models under MedLM are built on Med-PaLM 2, Google’s healthcare-tuned LLM. The first of these models is larger, designed to help users undertake complex tasks, while the second is a medium-sized model to help users scale and fine-tune the tool for various tasks.
The company plans to introduce additional tools into the MedLM family next year.