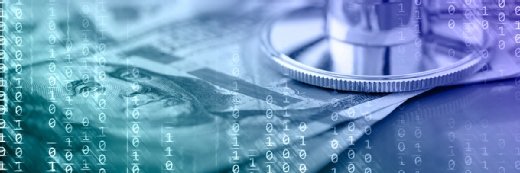
Viorika/istock via Getty Images
Deep Learning Model Predicts Mortality Following Medical Procedures
Patients classified as high risk for postoperative mortality by a new deep learning tool were nearly nine times more likely to die than their low-risk counterparts.
Researchers from the Smidt Heart Institute at Cedars-Sinai, Stanford University, and Columbia University have developed and validated a deep learning (DL) model capable of predicting postoperative mortality risk, according to a recent study published in The Lancet Digital Health.
The researchers underscored that current risk models are insufficient for predicting mortality following a medical procedure or surgery. To better assess these patients’ mortality risk, the research team set out to create an artificial intelligence (AI) tool that could stratify patients into risk categories.
“As it now stands, clinicians only have a modest ability to predict how a patient is going to do after surgery,” said corresponding author David Ouyang, MD, a cardiologist in the Department of Cardiology in the Smidt Heart Institute at Cedars-Sinai, in a press release detailing the study. “Current clinical risk prediction tools are insufficient. This AI model could potentially be used to determine exactly which patients should undergo an intervention and which patients might be too sick.”
To develop their tool, the researchers focused on patients who had received preoperative electrocardiographic diagnostic testing. Since an electrocardiogram (ECG) provides clinicians with a picture of how well a patient’s heart is functioning, the research team hypothesized that an AI tool may be able to use the tests to flag patterns relevant to predicting patient outcomes.
“This is the first electrocardiogram-based AI algorithm that predicts post-operative mortality,” Ouyang explained. “Previously, algorithms have been used to assess long-term mortality as well as individual disease states, but determining post-surgical outcomes helps inform the actual decision to do surgery.”
The researchers built the DL model by pairing patients’ preoperative ECGs with information about their postoperative outcomes. These data were pulled for 45,969 Cedars-Sinai Medical Center patients who had a complete ECG waveform image available for at least one 12-lead ECG performed within the 30 days before their procedure date between January 1, 2015, and December 31, 2019.
These patients made up the derivation cohort, which was split randomly into a 36,839-patient training dataset, a 4,549-patient internal validation dataset, and a 4,581-patient internal test dataset.
The model’s performance was measured using area under the receiver operating characteristic curve (AUC) values in the hold-out test dataset and two cohorts from Stanford and Columbia. These values were then compared with established Revised Cardiac Risk Index (RCRI) scores.
Overall, the DL tool achieved high performance.
In the held-out internal test cohort, the model predicted postoperative mortality with an AUC value of 0.83, outperforming the RCRI score, which reached an AUC of 0.67. The tool achieved similar performance in the Stanford and Columbia cohorts, with AUCs of 0.79 and 0.75, respectively
The DL model also performed similarly across procedure types, with an AUC of 0.85 for patients undergoing cardiac surgery, 0.83 for non-cardiac surgery, and 0.76 for catheterization- or endoscopy-based procedures.
While most patients were classified as low risk, the researchers found that those flagged by the algorithm as high risk had an almost 9-fold increased probability of postoperative mortality.
These results led the research team to conclude that the model has the potential to enhance risk stratification and clinical decision support for care teams determining whether surgery is appropriate for their patients.
“In cardiology, we're fortunate to have many life-saving procedures, everything from catheter-based procedures to open-heart surgery, so we're often trying to think about who are the right patients for the right procedure,” said Christine M. Albert, MD, MPH, chair of the Department of Cardiology in the Smidt Heart Institute and the other corresponding author of the study. “A better understanding of risk, particularly by using a commonly obtained diagnostic test, can inform important medical decisions.”
In the future, the researchers aim to develop a web application based on the model for use by clinicians and patients.
Deep learning tools are continuing to show promise in a variety of healthcare applications.
Last week, Mayo Clinic researchers shared how they had developed a deep neural network-based model to predict antihypertensive treatment success.
The research team noted that hypertension is a significant risk factor for other cardiovascular conditions, making management of the condition key to avoiding adverse outcomes. To better tailor individual hypertension treatment in primary care settings, the researchers built their DL model.
The model was largely successful, accurately identifying the most common and most successful treatments used by patients who achieved blood pressure control without moderate or severe adverse effects for at least a year after starting treatment.