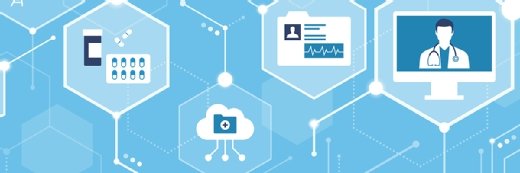
elenabs/istock via getty images
AI reveals association between body composition, lung cancer outcomes
New research shows that changes in muscle mass and fat quality during immunotherapy treatment are linked to poor outcomes in metastatic lung cancer.
An AI tool developed by Mass General Brigham researchers has revealed that changes in muscle mass and fat quality over the course of immunotherapy are associated with poorer outcomes for patients with advanced non-small cell lung cancer (NSCLC), according to a recent JAMA Oncology study.
The American Cancer Society indicates that lung cancer is the second most common cancer in the United States among men and women, leading to about one-fifth of all cancer deaths annually.
Treatments like immunotherapy are key to improving cancer outcomes, but the success of these approaches depends largely on a patient’s response to them. In a research spotlight published by Mass General Brigham, the research team underscored that clinical decision support tools to predict treatment response and outcomes could bridge this gap.
To build such a tool, the researchers turned to AI and medical imaging to assess the relationship between body composition and lung cancer treatment outcomes.
“Previous studies linked body mass index (BMI) with lung cancer outcomes and immunotherapy drug side effects. However, BMI is a limited measure that doesn't capture details about different body tissues and their interaction with cancer therapies,” explained lead author Tafadzwa Chaunzwa, MD, a researcher in the Artificial Intelligence in Medicine (AIM) Program at Mass General Brigham and a senior resident physician at the Harvard Radiation Oncology Program, and senior author Hugo Aerts, PhD, director of the AIM Program and associate professor at Harvard University.
They further noted that medical imaging-based body composition analyses are being explored, but such studies in the context of NSCLC have been limited.
The researchers began by developing a deep learning platform to analyze computed tomography (CT) scans to segment patients’ skeletal muscle (SM), subcutaneous adipose tissue (SAT) and visceral adipose tissue.
The model was applied to a mixed cohort of patients who received immunotherapy given alone or in combination with chemotherapy at the Dana-Farber Brigham Cancer Center (DFBCC), along with patients from the phase 1/2 Study 1108 and the chemotherapy arm of the phase 3 MYSTIC trial.
Outcomes were evaluated using baseline body composition measurements and any changes noted at the first follow-up scan, with associations among body composition, overall survival (OS) and progression-free survival (PFS) captured via hazard ratios.
Of the 1,791 patients in the analysis, 27.2% received chemoimmunotherapy at DFBCC, 46.1% received ICI monotherapy at DFBCC, 12.4% were treated with durvalumab monotherapy on Study 1108 and 14.3% were treated with chemotherapy on MYSTIC.
The findings revealed that a loss in SM mass was associated with worse outcomes across groups, but the association was most prevalent among males. Increases of five percent or more in SAT density were associated with poorer OS across cohorts and poorer PFS in the DFBCC group. However, this association was primarily observed among female patients.
“Our results demonstrate the potential of this analysis framework to provide a more nuanced understanding of the relationship between body composition and response to immunotherapy in NSCLC compared to crude BMI measurements. This may have important clinical implications for patient selection, treatment, and monitoring,” Chaunzwa and Aerts stated.
The research team is offering its software as an open-source AI tool in the hopes that doing so will accelerate research in this area and improve precision oncology efforts.
This research is the latest to explore how advanced predictive tools could enhance cancer care.
Last month, a research team from the University of Texas (UT) Southwestern Medical Center detailed how a computational tool could help predict breast cancer immunotherapy response and improve treatment.
The tool, known as InteractPrint, is designed to analyze how breast cancer epithelial cells impact cancer-immune interactions, enabling prediction of immune checkpoint inhibition response.
By shedding light on the cellular composition of specific tumors and how those cells interact with each other, the tool has significant potential to make cancer treatments more beneficial for patients.