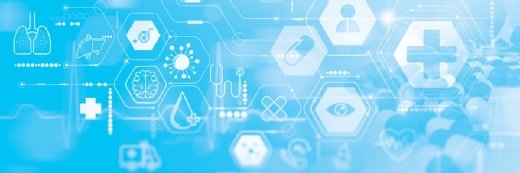
Deep learning model personalizes adaptive therapy for prostate cancer
A deep reinforcement learning model designed to tailor adaptive treatment schedules could double the time to progression for prostate cancer patients.
A research team from Moffitt Cancer Center and the University of Oxford has created a deep learning framework to help personalize adaptive therapy schedules for patients with prostate cancer.
The researchers underscored that conventional treatment strategies typically emphasize maximal cell killing, an approach that can fall short in treating metastatic cancers as a result of drug resistance. Adaptive therapy strategies offer a potential solution, as these focus on adjusting treatment decisions as tumor dynamics evolve in an effort to hinder cancer progression.
However, tailoring adaptive therapy based on a patient’s needs can be difficult.
To combat this, the researchers set out to develop a framework to generate interpretable and personalized adaptive treatment schedules.
The research team began by using a virtual patient model to train a deep reinforcement learning system.
Under this approach, patient blood tests are used to track the size of tumors, and these insights are incorporated into a mathematical model to simulate a virtual patient. From there, the virtual patient is used to finetune the deep learning tool, allowing researchers to generate personalized treatment schedules and inform future treatment.
This framework allows users to account for various tumor dynamics, enabling model adaptability when provided with data from individual patients to guide adaptive drug scheduling.
“By integrating evolutionary principles and mathematical models into adaptive therapy decision-making, our research opens new avenues for translating innovative treatment approaches into clinical practice,” said study lead Sandy Anderson, PhD, co-director of the Center of Excellence for Evolutionary Therapy at Moffitt, in the news release.
When calibrated to prostate cancer dynamics, the deep learning approach optimized adaptive treatment schedules, outperforming clinical standard-of-care protocols and doubling time to progression.
The success of the approach lies in its interpretability, which machine learning tools often lack.
“Interpretability has long been a significant hurdle to integrating machine learning approaches into clinical practice. While these frameworks are a black-box, and we can’t understand how they derive treatment recommendations, we can’t be confident applying these in the clinic,” noted first author Kit Gallagher, a University of Oxford student.
Moving forward, the research team plans to further refine the framework and investigate its potential application for other cancers.
“Model driven personalized medicine is the future of cancer treatment,” Anderson said. “By harnessing the power of deep reinforcement learning, we can optimize treatment strategies for each patient, leading to better outcomes and improved quality of life.”