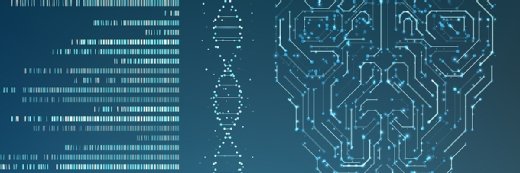
Nobi_Prizue/istock via Getty Ima
Machine Learning Links Age, Intensive Care to Pressure Ulcer Risk
Researchers used a machine-learning approach to discover the various factors, including demographics and level of care, that correspond with the development of pressure ulcers.
A machine-learning approach helped clinicians determine how factors such as age, level of care, anesthesia, and ventilation, impact the development of pressure ulcers in inpatient settings, according to research published in Scientific Reports.
Researchers used a machine-learning approach called the Bayesian Additive Regression Trees (BART), a system designed to define relationships between risk factors and outcomes.
Researchers used BART to examine 149,006 inpatient pressure ulcer cases between 2014 and 2018, all from a German university hospital. Researchers detected pressure ulcers in 4,663 or 3.1 percent of these cases.
They also found that 49.7 percent of cases included surgery and anesthesia use, more than 50 percent of cases took place in only one ward, and 19.6 percent involved intensive care. Of the patients that received intensive care, 4.1 percent needed ventilation, while 15.5 percent did not.
Using various statistical analyses, researchers found that the probability of pressure ulcers correlated with a patient’s presence in intensive care units, age, length of anesthesia, and the number of wards where treatment took place.
The likelihood of pressure ulcers appearing within the ICU was eight times higher if ventilation was needed than in cases that did not include intensive care or ventilation.
The probability of pressure ulcers also increased with an increase in anesthesia levels. In this study, researchers observed that among cases with 50 to 120 minutes of anesthesia, the chance of pressure ulcers developing increased with time.
As age increased, the chances of incident pressure ulcers did as well. The likelihood of pressure ulcers increased slightly for patients between the ages of 35 and 50. However, it tripled between the ages of 50 and 90 years.
Also, the chance of pressure ulcers developing was 1.5 times more likely if other hospitals transferred a case than in-hospital referral admissions.
Not only that, but when more than one hospital ward participated in the care process, the probability of pressure ulcers increased.
Researchers acknowledged that the single setting in which they conducted research may serve as a limitation and that "a randomized controlled trial in a large sample would be valuable."
Using machine-learning approaches to enhance clinical care delivery is becoming a common practice.
For example, central Pennsylvania-based Geisinger Health System implemented a protocol that included using artificial intelligence and machine learning to discover colorectal cancer risk.
Another study published in Cardiovascular Research described a machine-learning approach that could predict the risk of a heart attack. The process included scoring coronary artery calcium using non-contrast computed tomography and determining the relationship between its progression and the likelihood of cardiovascular disease.