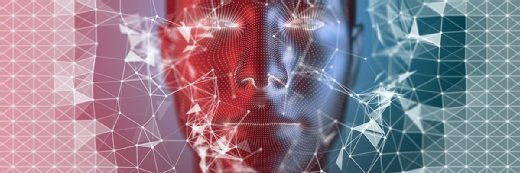
Getty Images
Machine-Learning Algorithm Flags High-Risk Colorectal Cancer Patients
A machine-learning algorithm helped clinicians detect patients at high risk for colorectal cancer, enabling them to schedule colonoscopies for these patients, a new study shows.
A machine-learning algorithm was able to provide high-risk patients who missed a colonoscopy with information regarding the type of treatment needed, according to a study conducted by Geisinger and Medial EarlySign.
Geisinger, which includes 10 hospital campuses, a health plan that cares for more than 500,000 members, a research Institute and the Geisinger Commonwealth School of Medicine, worked with software company Medial EarlySign to study the algorithm. Findings were published in NEJM Catalyst Innovations in Care Delivery.
The study consisted of 25,610 patients who Geisinger defined as overdue for colorectal cancer (CRC) screening. Researchers then used a machine-learning algorithm to determine which patients were at risk for developing cancer.
The algorithm analyzed various factors such as age, gender, and a recent outpatient complete blood count.
Based on the analysis, nurses called high-risk patients to schedule a colonoscopy.
They were able to schedule a colonoscopy for about 68 percent of high-risk patients. Further, about 70 percent of patients flagged by the algorithm had a significant finding.
“When carefully implemented and supported by healthcare providers, machine learning can be a low-cost, noninvasive supplement to other colorectal cancer screening efforts,” said Keith Boell, DO, co-author of the study and chief quality officer for population initiatives at Geisinger, in the press release. “This technology can act as a safety net, potentially preventing missed or delayed diagnosis among some patients who may already have undiagnosed signs of disease.”
Although there are various benefits associated with routine CRC screening, the National Cancer Institute found that nearly one-third (32 percent) of adults in the United States do not follow screening guidelines. Early detection of CRC is critical as it can significantly decrease the probability of death.
As with CRC, machine learning has been proven effective in detecting and treating other diseases.
In October 2021, engineers from Johns Hopkins University created a non-invasive optical probe to study tumors. They developed a machine-learning model to monitor changes in tumors after immunotherapy. Researchers trained the algorithmic model with 7,500 spectral data points from 25 tumors.
During the pandemic, machine learning has also been used to predict which patients may be at risk for developing severe COVID-19.
A study from Infectious Diseases of Poverty, which included COVID-19 patients from JinYanTan hospital in Wuhan, China, tested three different machine-learning techniques. Researchers found that one of the techniques could reliably and accurately predict the severity of the disease.