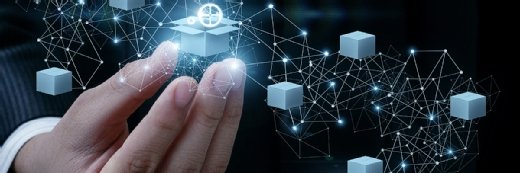
Getty Images/iStockphoto
Why UCI Researchers Created a Framework for Analyzing Wearables Data
The framework provides researchers using wearables data in clinical studies with minimum reporting thresholds to ensure data standardization and validity.
Wearables — electronic devices that can be worn as accessories — are gaining steam in healthcare, with Deloitte predicting that 320 million consumer health and wellness wearable devices will ship worldwide in 2022.
These devices enable patients to monitor various health metrics, like daily steps, sleep quality, and heart rate, to help them achieve their health goals.
For providers, wearables offer a whole new category of data that can be used to enhance patient outcomes and care delivery. But collecting, standardizing, and validating the data, which are necessary precursors to data analytics, can be a challenge for many health systems.
To help remedy this problem, a team led by University of California, Irvine researchers developed a framework for standardizing wearables data reporting and validation. They published the framework and details about the development process in the International Journal of Medical Informatics.
The problem
Alexandre Chan, PharmD, corresponding author of the framework, first identified the issues related to wearables data management a few years ago when he was heading a study examining cancer patients' response to treatment.
All the patients in that study received a Fitbit so researchers could track activity levels during their treatment and how those levels correlated with potential side effects.
"The amount of data that we were getting for each patient, we were starting to wonder — how can we see through all that data?" said Chan, who is founding chair and professor of clinical pharmacy at UC Irvine's department of clinical pharmacy practice, in a phone interview. "Especially because with this sort of big data related to trackers, it's not as straightforward… we are getting data almost every second, every minute or so, so we need to make sure that there's a way that we can clean up data."
At the time of the cancer study, Chan and his fellow researchers set aside the larger data management issue as they were dealing with small sample sizes.
But now, literature focusing on wearables data has grown, and Chan and his team discovered many discrepancies in collecting and classifying the data.
For example, different studies are using different metrics to measure activity levels. Some use total step counts, some use average step counts, while others look at activity intensity — light, moderate, vigorous —and how much time people are spending in these activities in addition to quantifying activity levels, Chan explained.
Then there is the issue of validating the data collected in a standardized way. Different studies have different rules in place to validate data.
"Some studies are more stringent and say, 'Well, you must have at least five valid days [of data],'" Chan said. "That means I can show some evidence that somebody's wearing the watch for at least five out of seven days. But some studies, I think they know that patients are simply not wearing [the device], so [they say], 'Let's be more lax on that rule. Let's make it three days.' So, you already see a discrepancy, right? How do I know whether I can trust the data?"
Thus, there is a need for a minimum threshold framework for reporting, validity period, and physical activity measures in clinical studies.
"It's easy for us to say that we can get data from these activity trackers and try to correlate it with clinical outcomes, but if you don't do [data management] well and just assume that data will just flow in…we may be actually getting not-so-useful information," Chan said.
The framework
To develop the framework, Chan and his fellow researchers performed a systematic review of clinical studies using the Pubmed and Embase databases. They selected 27 studies published between 2009 and March 2021, which enrolled at least 1,000 human subjects and involved the use of activity trackers of any brand.
The team, which included Daniella Chan, a UCI pharmaceutical sciences student, found several inconsistencies and certain commonalities in measurement and reporting data.
Based on their analysis, the research team offered the following minimum threshold framework for studies involving wearables data:
- Studies should report at least one measure of device adherence, for example, the percentage of days the activity trackers were worn.
- Studies should report whether participants have demonstrated adequate wear time per day and per week.
- Several physical activity measures should be reported, including step count, sedentary activity, acceleration levels, energy expenditure, and activity intensity.
"[You have] got to come up with a plan even before walking into that research study because if you just assume that you provide that tracker to a patient and data will just come in and then you can do just use the data directly without any processing, I think that's way too naïve," Chan said. "Making sure that certain rules are being set up and deciding whether or not certain data points are valid [before] we even go into analyzing the data, I think that is very important."
Proposed benefits
One of the most significant benefits of this framework is to help researchers develop the appropriate formula and syntax for analyzing wearables data.
For example, it may not be best for researchers to analyze the average step count within a specific time period because some patients may show a spike in their step count if they have an unusually active day, thereby skewing the data being collected. Instead, researchers can consider analyzing step counts within different blocks of time.
Having a minimum threshold for reporting not only helps researchers select the correct variables for analysis but also helps ensure that the data is clinically meaningful.
"Without that sort of framework…you would not be generating data that is meaningful at the end," Chan said. " And I think that's kind of sad because you may be involving tons of patients, spending lots of time… at the end, if the data is not valid? Why should we even bother doing the study to start with then?"
Ultimately, the framework can help ensure the data used for analysis is standardized, which is essential for developing comprehensive wearables-based treatment recommendations for health conditions.
"The bottom line is that if the step data that we are getting from patients and the way how we process them is not standardized, we are comparing apples and oranges when we look at different studies," Chan said. "And we simply just cannot be coming up with concrete solutions where we look at all these different studies out there."