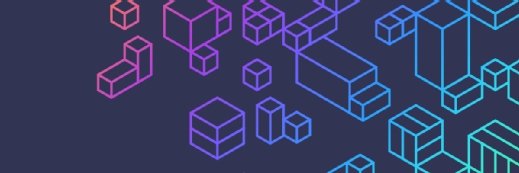
What Providers Can Do to Minimize AI-Based Image Reconstruction Risks
Distortion risks associated with AI-based image reconstruction can lead to inaccurate diagnoses, and though the overall risk is low, providers need to be aware of the issue to ensure patient safety.
Artificial intelligence is increasingly being used to reconstruct images from data obtained during magnetic resonance imaging, computerized tomography, or other types of scans. While AI has been shown to improve the quality of scans and speed up reconstruction compared with standard algorithms, there are concerns that the new technique can distort images and lead to patient safety issues.
ECRI, an independent nonprofit focused on improving the quality of care, listed this concern as one of its top 10 health tech hazards for 2022.
"What we're concerned about and why it's in the top 10 is that AI is not a magic wand," said Jason Launders, director of operations at ECRI, in a phone interview. "You have to be very careful in how it's used. The manufacturers have to be open and transparent as to exactly what are the limitations of their specific AI methodology."
Though some experts believe that this is not a significant issue in practice, there are still potential risks providers must consider when using AI algorithms for image reconstruction, including how the algorithm has been trained.
Limitations of AI-based image reconstruction
There are multiple reasons why AI is being used more often for imaging, including its ability to reduce the time taken for scans and improve the image quality without increasing radiation dose, Launders said.
But there are risks involved, including that AI is usually trained for specific use cases, explained Francisco Rodriguez-Campos, senior project officer at ECRI, in a phone interview.
"You think [about] the amount of data that will be required to train for every single possible combination of factors to be able to reconstruct those images," Rodriguez-Campos said. "That's part of this big issue at hand."
If radiologists start using AI-based imaging technology outside the bounds within which they have been developed, there could be subtle changes to the images that the radiologist may overlook, Launders added.
For example, tiny perturbations during the image capture process may result in severe artifacts, which are features that appear in an image that are not present in the original object being scanned. This could obscure small structural changes, such as tumors, which can seriously impact diagnostic interpretation.
"[If] the AI takes pathology, a subtle pathology, and makes it invisible in the final image, that is obviously going to be a problem," Launders said. "The real problem is you don't know when this is happening."
Further, radiologists may not be aware of how prevalent AI is becoming in imaging and, as a result, may not know how to predict the risks.
"If they're not aware of how much it's being used, it's impossible to estimate what the risk is," Launders said. "It's very easy for manufacturers to wave their hands around and say, 'This is what's happening. This is how we develop the images.' At the end of the day, it's the users who are looking at those images, and they have to be aware of [the] risks of how AI is being used."
How concerned should providers be?
Though there is a potential for image distortion with AI-based reconstruction, according to Greg Zaharchuk, MD, PhD, professor of radiology at Stanford Medicine and co-founder of AI imaging company Subtle Medical, the risk is low.
"I would say that the number of situations that such exactly precise perturbations of the data would occur naturally is extremely uncommon," he said in a phone interview. "All [imaging algorithms] can be manipulated in that way if you choose. So, for that reason…99 percent of practicing radiologists do not think this is a concern."
Ultimately, imaging modalities across the board are full of artifacts, and one of the jobs of a radiologist is to identify what is useful in an image, Zaharchuk added.
Further, safeguards are built into the diagnosis process, including that radiologists use multiple images for any diagnosis, which helps them identify the valuable features in the images.
"Most people, I would say, inside the imaging world, if we've seen these kinds of concerns raised about AI have really not been extremely concerned about them because we do know how we use images every day," Zaharchuk said.
What providers need to know
But, despite the seemingly low risk of image distortion, this is still an issue providers need to watch for and take steps to mitigate when possible.
"This is something that people need to be aware of," Launders said. "We're not saying AI is bad. We're not saying do not use AI. What we are saying is: be careful."
One way to mitigate risks when using AI-based image reconstruction is to ask manufacturers the right questions about their offerings and potential limitations. For example, ECRI's Rodriguez-Campos suggests making sure the AI has been trained using data from your target population.
"As you know, AI is biased," he said. "It will be biased based on the training data."
Further, Rodriguez-Campos suggests creating risk assessment committees or procedures to validate AI imaging tools in-house using target population data.
Stanford Medicine's Zaharchuk echoed Rodriguez-Campos, suggesting that providers consider trialing any new AI system they plan to implement.
"I think you need to balance the risk…I think the best way to do that for people is to use their own data and evaluate [the technology]," Zaharchuk said.
The experts mHealthIntelligence spoke with all agree that AI use in imaging will continue to grow.
For Launders, this is a crucial use of today's enhanced computer processing capabilities. But being aware of its limitations is essential.
"From a basic image science perspective, AI seems to be magic," Launders said. "But you cannot reduce the dose or the time so much that you're making an image out of no X-rays or no MR signal. Whenever that happens, you always have to ask yourself, 'Well, how is it coming up with that better image? What is it doing that we couldn't do before?' If you can't answer that question, then I'm very wary."
Correction: A previous version of this article made an inaccurate reference to a PNAS research paper. The article was updated to remove the reference on 3/11 at 3:46 pm CT.