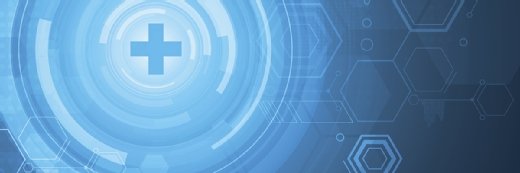
traffic_analyzer/DigitalVision V
What Are the Top Challenges of Clinical Decision Support Tools?
Clinical decision support tools can provide actionable information, but issues like alarm fatigue can increase clinician burnout.
Clinical decision support tools can help organizations manage large volumes of data while enabling them to deliver quality, value-based care.
Designed to sort through large amounts of data and provide clinicians with actionable insights, these tools can suggest next steps for treatment, catch potential problems, and alert providers to information they may have missed.
However, if poorly designed or implemented, clinical decision support systems can cause more problems than they solve. Alarm fatigue, physician burnout, and medication errors are all detrimental side effects of unintuitive clinical decision support technology, with these events harming patient outcomes and organizations’ bottom lines.
What are the major challenges with clinical decision support implementation and use, and how can developers and provider organizations overcome these barriers?
CLINICAL DECISION SUPPORT SYSTEMS AND BURNOUT
Clinical decision support systems embedded into the electronic health record (EHR) have the potential to reduce care errors and improve medication adherence rates.
But these tools can also contribute to clinician frustration and burnout. According to a national survey co-authored by the American Medical Association (AMA), physician burnout rates spiked to 63 percent at the end of 2021.
In a 2020 study conducted by researchers at Stanford University School of Medicine, the group stated that an estimated 35 to 60 percent of clinicians experienced symptoms of burnout and called for increased efforts to address it.
The team recommended that healthcare stakeholders consider several factors when designing and implementing clinical decision support to minimize clinician burnout.
“End-users should be involved in all aspects of design, pre-testing, and implementation. [Clinical decision support] requires ongoing maintenance based on feedback and outcomes, as well as updates to clinical practice standards,” the group said.
Previous research has highlighted the importance of user feedback and involvement in clinical decision support tool development. A 2018 study published in JAMIA showed that using natural language processing (NLP) techniques to analyze user feedback and override comments in clinical decision support systems could help organizations identify malfunctioning or broken alerts.
This could help eliminate unnecessary notifications, reducing clinician burnout and fatigue.
The research team emphasized that user feedback provided via override comments is an underutilized but valuable data source for improving clinical decision support tools. They recommended that healthcare organizations with the resources to do so should evaluate all override comments and use these to guide system improvement efforts.
However, increased integration of advanced analytics technologies like NLP in clinical decision support tools comes with additional challenges that healthcare organizations must navigate.
THE GROWING INFLUENCE – AND POTENTIAL RISK – OF AI
With the rise of artificial intelligence (AI) and machine learning (ML) in healthcare, researchers and provider organizations have begun to apply these technologies to clinical decision support tools.
However, combining these systems with advanced analytics often introduces its own set of challenges.
Experts from Mass General Brigham and Brigham and Women’s Hospital have cautioned that physicians may blindly accept the outputs generated by AI and ML systems, which could lead to unintended harms stemming from racial bias in algorithms and impaired clinical decision-making.
Healthcare leaders are familiar with concerns around automation bias and clinician dependency on AI, but these anxieties are growing as health systems increasingly pursue AI deployment.
However, many agree that the risk of clinicians becoming over-reliant on AI and ML is low as long as care teams understand how these tools make their recommendations and view the technologies as assistants, instead of replacements for clinical expertise.
Further, healthcare already has some strategies that have worked in the past to help prevent and address potential over-reliance, as the implementation of EHRs and other tools also created concerns about automation bias.
Creating transparency around how these tools work and how they should be used is a key part of addressing these concerns, and involving clinicians in that process can help build trust and ensure the responsible use of AI and ML.
Building accountability into the AI-driven decision-making process by establishing governance infrastructure and ensuring that a human being is involved at every step of a tool’s use can provide additional safeguards.
In doing so, monitoring and flagging an AI-driven clinical decision support system if issues arise becomes easier.
Clinician involvement can also improve the on-site development of clinical decision support tools in health systems with the resources to do so. Clinical knowledge is useful for troubleshooting why a model may not be successful for a particular use case or helping guide modifications to enhance an algorithm’s performance.
Some healthcare systems have already been utilizing this ‘human in the loop’ approach to improve clinical decision making and care delivery.
For example, AI and ML tools can act as “real-time listeners,” that utilize clinician dictations to generate reports. This streamlines workflows for creating reports and can provide clinical decision support by making recommendations for next steps based on relevant report details.
However, before clinicians use these tools, they should undergo educational training to understand best practices for leveraging the technologies in different use cases.
Some organizations have also found success pulling patient cases and running them through the tools in order to test their performance against a provider’s actual recommendations. In doing so, healthcare organizations can ensure suggestions are aligned with clinical practice standards.
Organizations should also have multiple real-time feedback mechanisms for clinicians to report any false negatives or false positives generated by the tools, or any other issues that may arise. Feedback should be sent to the organization’s data science team for troubleshooting and model retraining in the event of a tool providing incorrect outputs.
Having a framework to monitor and address potential issues with the clinical decision support tools ensures that the tools can make the workflow more efficient for clinicians, while the educational and feedback components help avoid automation bias.
But if a clinical decision support tool is missing critical information, its utility will be severely limited.
ADDRESSING MISSED INFORMATION, DIAGNOSTIC ERRORS
Diagnostic errors are a massive patient safety hazard, resulting in care gaps, unnecessary procedures, and patient harm.
Sometimes, these errors can be attributed to missing information or interoperability issues present in clinical decision support systems. While the use of AI has the potential to reduce some of these errors, these technologies cannot currently solve the problem.
Diagnostic errors are common in the United States, with the Agency for Healthcare Research and Quality (AHRQ) estimating that one in 20 adults experiences such an error in outpatient settings and 250,000 Americans experience them in hospitals each year.
These errors – which result from a diagnosis being delayed, poorly communicated, or incorrect – can have serious consequences for patients. The Society to Improve Diagnosis in Medicine indicates that evidence of a major diagnostic error is found in anywhere from 10 to 20 percent of US autopsies, suggesting that these errors contribute to 40,000 to 80,000 deaths annually.
Clinical decision support tools can help prevent diagnostic errors by flagging incidental findings in a patient’s record that may warrant follow-up or by identifying ordered tests that haven’t yet been completed.
Clinical decision support tools that incorporate ‘hard stops’ – in which a response is required before a user can move forward with a task – can help improve patient outcomes when used appropriately.
Hard stops are one of three alert categories common in clinical decision support systems, alongside ‘soft stops’ and passive alerts, according to a 2018 JAMIA study.
The researchers defined soft stop alerts as “those in which the user is allowed to proceed against the recommendations presented in the alert as long as an active acknowledgement reason is entered.” Passive alerts are those “in which information is presented but does not interrupt the user workflow and does not require any interaction on the part of the user.”
In a 2023 article, the Institute for Safe Medication Practices (ISMP) demonstrated that alert fatigue – a phenomenon that occurs when clinicians become desensitized to alerts due to the sheer number and frequency of such alerts – can lead providers disregarding valuable information that could prevent patient harm.
Passive alerts are easiest to ignore as they require no user action, but soft stops may only require clinicians to acknowledge the alert with minimal or no action, making them relatively easy to ignore.
Hard stops, however, completely halt the progression of a process like medication prescription or administration that could harm a patient. In certain cases, a hard stop can help identify and prevent a potential adverse event before it occurs, improving patient outcomes.
But hard stops are not without their pitfalls.
ISMP further indicated that clinicians must sometimes work around barriers like hard stops in order to provide patient care, leading them to circumvent these alerts. Often, this occurs because they do not recognize the issue the alert is trying to prevent or that the rationale for the hard stop is unclear.
While alerts in clinical decision support tools play a key role in preventing diagnostic errors, the presence of alert fatigue and inappropriate alerts – like a hard stop that clinicians must work around – can undermine patient safety by increasing cognitive load for clinicians.
To combat this, the ISMP recommends certain best practices for hard stop implementation, including establishing oversight, evaluating EHR systems, assessing if and where hard stops are necessary, using hard stops judiciously, developing an escalation process to tackle hard stop workarounds, using objective measures to evaluate alert appropriateness and utility, performing functional testing, gathering feedback, and collaborating with clinical decision support technology vendors.
Across the board, user testing and feedback are critical to ensure that clinical decision support systems are pulling and flagging all the information needed to guide decision-making in a way that positively impacts diagnostic error rates and patient safety.