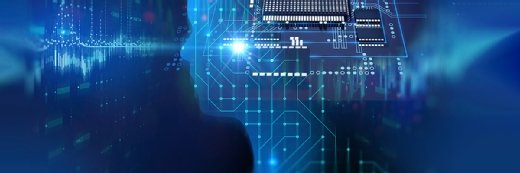
Getty Images
WEDI Conf. looks at AI for ROI & interoperability
WEDI’s Spring Conference saw experts discussing best practices and emerging trends in artificial intelligence and health information exchange.
Healthcare leaders came together this week virtually for the 2024 Workgroup for Electronic Data Interchange (WEDI) Spring Conference to explore trends, standards, lessons learned and best practices for data exchange in healthcare.
In addition to conversations about prior authorization and cybersecurity, stakeholders spoke about the opportunities and pitfalls of emerging technologies to improve care quality, drive innovation and reduce costs.
These presentations were dedicated to detailing how advanced technologies are set to transform the interoperability landscape and helping healthcare organizations effectively take advantage of tools like AI.
HOW APIs, AI & APPs WILL TRANSFORM INTEROPERABILITY
The adoption of EHRs means healthcare stakeholders have more opportunities than ever to utilize that information to improve care delivery, but the pursuit of interoperability is ongoing. A host of roadblocks have slowed national data exchange efforts over the past 15 years.
Ryan Howells, program manager at the CARIN Alliance and principal at Leavitt Partners, emphasized that the use of application programming interfaces (APIs), AI, and consumer and provider applications are set to help make data actionable and transform digital health in the next 15 years.
Before healthcare stakeholders can begin to take advantage of these tools, however, Howells noted that trust is the foundation of any effort to improve interoperability and innovation.
“Trust is the new currency in the application economy,” he explained. “If we're going to have a digital health ecosystem and nationwide interoperability, we have to figure out this conundrum of trust and how we solve it as a country. Trust and transparency go together, and for us to have trust in the ecosystem, we need to figure out ways where that transparency can take place as well.”
Howells indicated that establishing and maintaining that trust on a national scale will be challenging, but that stakeholder collaboration could help.
“One sector does not an industry make,” he said. “We have plenty of problems as a country in terms of healthcare access, affordability, the list goes on and on and on… but we can't rely on one sector to actually solve all those problems. We need everyone at the table trying to find ways to do that.”
While interoperability is exciting to many in the industry, funding for data exchange efforts is often lacking, Howells stressed. While public sector stakeholders have worked to conceptualize interoperability and potential requirements to advance it, Howells stated that making the business case for interoperability’s importance is also necessary.
Additionally, in conversations around the technologies and tools that will drive interoperability, he indicated that differentiating between innovation, functionality, commodities and platforms is key.
“There are certain technologies that are out there that are certainly innovative when they first come out, but they quickly move to functionality, and ultimately, commodities that are on top of platforms over time,” Howells said. “What are the true innovations that are out there that we need to support? And what other feature functions and commodities do we need to make available on platforms for providers and consumers in the future?”
These considerations are critical when discussing the future of interoperability, Howells noted. Legacy providers are key to digitizing health records, but that the use of APIs, cloud and AI tools will become increasingly important both from a regulatory and innovation perspective.
“How do we find ways to be able to answer more questions at the right time in the right place for the right patient to be able to provide better outcomes at the point of care? What are the opportunities and challenges we have to making this dream a reality?”
Take, for example, the Change Healthcare cyberattack. Many healthcare providers didn’t even know that the company was involved in processing their payments, let alone how to find answers to their questions in the wake of the attack, he said.
“This is a risk to the ecosystem because we have situations where so much of the infrastructure that we have in healthcare technology is still new, and somewhat brittle, especially nationwide, and we haven't tested it the way that we're going to test it in the years ahead,” Howells posited.
There will be more attacks, and finding ways to combat them, recover from them and protect patients from the fallout is a major consideration in the interoperability conversation, he stated.
Increased oversight and governance of healthcare data exchange—such as the Department of Homeland Security’s Cybersecurity and Infrastructure Security Agency (CISA) proposed rule, which would create cyber incident and ransom payment reporting requirements—are critical to building the foundation of transparency and trust that interoperability must be built on, he continued.
Alongside trust and transparency, technological support for initiatives like the Trusted Exchange Framework and Common Agreement (TEFCA) remains a pain point for interoperability. APIs, AI and apps have significant potential to enhance scalability and support critical infrastructure, Howells indicated.
“The volume of information and data that is going to be exchanged—both clinical data and claims-related data—is more substantial than we've ever done in the history of the country. We don't know if the current infrastructure is going to support that, and we're going to need to ask those questions. We're going to need to use cloud-based technologies to ensure we have enough [computing power],” he stated.
Despite this, Howells emphasized that caution is needed when considering the role of tools like AI.
“We don't know how [AI] works,” he asserted. “We cannot provide consistent responses yet with AI. That provides a policy challenge for us, a significant policy challenge, because it's very difficult for the policies to then be able to address the needs that are happening in AI today.”
He further explained that the federal government is currently ill-equipped to regulate the technology, and determining appropriate regulatory infrastructure is not straightforward. Issues also arise when thinking about how AI deployment will impact costs.
While many assert that AI will decrease software development and other costs, this may not necessarily be the case. Howells explained that, based on the Jevons paradox, the cost of developing software and AI may decrease in the short term in line with advances in efficiency, but low costs and high efficiency may create high demand. As demand for these technologies increases, the costs may, as well.
“So, I wouldn't necessarily view AI as just a reducer in administrative costs,” he noted. “I would actually look at AI as potentially increasing costs over time, but also providing better outcomes, more democratized access to information.”
As healthcare stakeholders navigate these hurdles and the changing interoperability landscape, Howells recommended that developing corporate strategies for APIs and other technologies will be crucial in the coming years.
CHOOSING THE RIGHT AI USE CASES FOR ROI
For healthcare organizations that already have enterprise strategies for APIs and AI, identifying the most valuable use cases for the tools is a top priority. However, actually choosing an AI solution capable of meaningfully contributing to KPIs remains a challenge.
For Chris Lance, chief product officer at Edifecs, the question of how to adopt AI begins with a basic understanding of the types and potential applications of the technology.
“Machine learning is a discipline that involves training a model for a statistical computer program, ultimately, to use data from the past or from a specific instance or use case to infer future results,” he explained, noting that while many people think of AI and machine learning separately, AI is the context in which machine learning exists.
He further noted that AI tools are deployed using the concept of a model, wherein an AI can approximate or replicate how different scenarios could play out in the real world.
“This model allows us to interrogate it, and poke at it, and prod it, and try to ask it questions—and hopefully it responds in the same way that the world would, or will—so that we can predict things that haven't occurred yet, or so we can better understand those scenarios without having to go into the world and do those things,” Lance stated.
He explained that these models are built using algorithms that generally fall into three categories: regression, classification or generative.
Regression algorithms are useful for making predictions based on historical data, while classification algorithms can help group or categorize similar data points or inputs to make them easier to analyze.
Generative models, as the name suggests, are designed to create content using large-scale statistical models and vast datasets. Lance highlighted that one type of generative AI, known as foundation models, has been particularly transformative. Foundation models are pre-trained, allowing developers to then take the model and fine-tune it using a smaller amount of data.
By building on top of a foundation model, stakeholders could more easily build complex, customized AI.
From there, Lance emphasized that stakeholders must have confidence that they can affect change within their organization by responsibly and pragmatically considering the potential use of AI.
Lance outlined three pillars to approach AI adoption, the first of which underscores that AI is a tool, not a reason.
He explained that stakeholders already know the reasoning behind what they do in their organizations, and the hype around AI is not cause to adopt it. Instead, Lance advised that organizations should look at the problems they’re facing and consider how a tool like AI could enable them to do things differently or do something new that would positively impact the way they solve that problem. Additionally, this provides an opportunity to look at previously unsolvable problems to determine whether AI is part of a potential solution.
The second pillar is concerned with understanding the operating context of the organization and its problems.
“If you're going to improve something, you need to understand what your baseline and benchmark is,” Lance noted. “Understand the levers, the drivers and the performance characteristics of the environment that you're trying to improve before you start trying to solve for it. You may find again that AI is not the solution for the job, or it's overkill.”
The final pillar emphasizes returning to the “why” behind an organization’s desire to pursue AI adoption – the potential ROI.
“You're doing this to improve something. The return is generally financial, but it doesn't have to be,” Lance stated. “But there's an investment that you're making and a risk that you're taking. Make sure that you're focused on what it is you're actually trying to achieve.”
“What are the problems you have that are hard for you to solve today, and are those the sorts of things that may be able to be addressed now using new technologies such as AI?” he continued.
Alongside these considerations, Lance highlighted that responsible adoption of any tool is critical, noting that models approximate the real world, but are not substitutes for it. In that vein, he indicated that the outputs of AI models must be understood as predictions, rather than guarantees.
For example, for an algorithm predicting whether an email is spam, the pros outweigh the cons. In that case, the convenience of not having to sift through spam emails manually is likely to outweigh the handful of times when the tool misses a spam email or accidentally labels something as spam incorrectly.
But this doesn’t apply in a scenario like payroll, in which an employer may want to use an algorithm to predict what employees’ paychecks ought to be.
Being able to determine when an approximation versus a more exact approach is necessary to successfully identify AI use cases for ROI in a healthcare organization, Lance noted.
The second concept related to responsible AI adoption centers on bias.
“Models only know what you teach them, and if you only feed them information in narrow contexts, they will only be able to predict a reason in that context,” Lance explained, indicating that models, like children, can be taught about diversity of information sources, people, contexts and other factors. “Make sure that you are providing a diverse experience to your models so that they can provide diverse and appropriate predictions for all contexts.”
For healthcare organizations at the beginning of their AI journeys, Lance recommended that they focus on going inch by inch, rather than yard by yard.
“What you really want to do is build your capabilities incrementally,” he said. “Don't try to boil the ocean or go for the silver bullet. You want to make sure that you understand the application of the individual types of models, the combinations of those models, the influence that they have on one another and the efficacy of their output. Before you start trying to do large complex things or making investments across all the different model types or algorithms, you might find that there are only one or two of them that are truly useful in your context.”