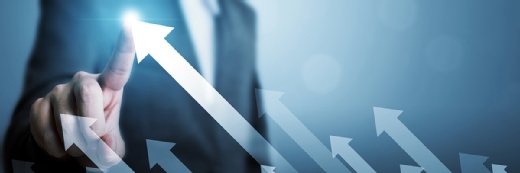
Getty Images/iStockphoto
Using Big Data Analytics for Patient Safety, Hospital Acquired Conditions
Big data analytics can provide valuable insight into avoiding patient safety events and reducing the incidence of hospital acquired conditions.

Prediction and prevention are the two main goals for patient safety experts seeking to avoid adverse events and reduce the prevalence of hospital acquired conditions (HACs).
While workflow strategies, staff training, and human factors play a critical role in helping hospitals get ahead of infections, falls, pressure ulcers, and medication errors, big data analytics tools are becoming increasingly important in the age of digital care.
The electronic health record – when implemented well – can become a valuable hub for information and a key tool for communication across care teams.
When predictive analytics and machine learning are applied to EHR data and input from bedside devices, healthcare providers can access powerful clinical decision support that may help to catch human errors and prevent costly adverse events.
In conjunction with improved guidelines, federal support, and pressure from financial penalties, big data analytics tools are helping to rapidly reduce the incidence of potentially deadly infections and other HACs.
Patient safety events decreased by 17 percent between 2010 and 2013, according to HHS, saving more than $12 billion and avoiding the unnecessary deaths of approximately 50,000 people.
How can healthcare organizations continue this positive trajectory by taking advantage of predictive analytics, clinical decision support tools, and other data-driven strategies, especially in the inpatient setting?
What is a hospital acquired condition?
Hospital acquired conditions and healthcare associated/hospital acquired infections (HAIs) are reportable incidents that originate or occur in the healthcare setting.
The term has specific meaning for Medicare, which uses an MS-DRG reimbursement code to identify HACs and present on admission (POA) conditions and apportion reimbursement accordingly.
Healthcare organizations do not get reimbursed for the cost of treating a condition that was acquired in the hospital, such as a hip fracture after a preventable fall from an inpatient bed.

If the condition is present upon admission, assuming the admission is not subject to other programs with readmissions reduction criteria, the hospital can receive reimbursement for necessary care.
Since 2013, the list of HACs has included:
- Object left in patient during surgery
- Air embolism
- Blood incompatibility
- Pressure ulcers (stage III and IV)
- Falls and trauma
- Manifestations of poor glycemic control
- Catheter associated urinary tract infection (CAUTI)
- Vascular catheter associated infection
- Surgical site infection for specific conditions
- Deep vein thrombosis (DVT) or pulmonary embolism (PE) following surgery
- Iatrogenic pneumothorax with venous catheterization
CMS tracks a number of other patient safety events, as well, and scores hospitals on quality through the HAC Reduction Program (HACRP).
In addition to the above, hospitals can lose points on their CMS Recalibrated Patient Safety Indicator (PSI) 90 scores by being responsible for high rates of postoperative sepsis, respiratory failure, acute kidney industry, and wound dehiscence rates, as well as performing poorly on central-line associated blood stream infection (CLABSI) rates, MRSA infections, and Clostridium difficile (C. diff) infections.
Low PSI 90 scores result in reimbursement reductions. The lowest-performing 25 percent of hospitals receive a 1 percent cut in their Medicare reimbursements, regardless of whether or not they improve over their own baseline during the monitoring period.
For this reason, the program has been controversial, with the American Hospital Association (AHA) coming out strongly against the notion that more than 700 hospitals will lose revenue every year despite dramatic overall reductions in harm.
“The HACRP is poorly designed and imposes arbitrary, excessive penalties that disproportionately impact hospitals tending to care for the sickest patients,” the AHA said in a 2016 statement to the House Ways and Means Committee.
“Data show that hospitals treating complex patients are disproportionally penalized, in part because the HACRP uses claims-based patient safety indicators (PSIs) that are unreliable and do not reflect important details of a patient’s risk factors and course of care.”
Inaccurate reporting is a key pain point for the program. Hospitals that are better at identifying and reporting patient safety events will naturally have higher rates of HACs, creating a fundamental disincentive for providers to take a responsible, data-driven approach to flagging HACs when they occur.
In this complex financial environment, it is important for hospitals to focus on the prevention of potentially costly events so as to avoid the issue all together.
How are organizations using data analytics to predict and prevent HACs?
Big data analytics can provide patient safety teams with the insights they need to get ahead of HACs, but developing effective processes and tools can be a significant challenge.
Data on HACs and HAIs is often difficult to collect, leaving healthcare organizations with gaps in their knowledge about what causes these conditions and how to prevent them.
“Unfortunately, many of the current HAI surveillance strategies are labor intensive and subject to limitations as a result of poor inter-rater reliability in applying standard definitions and variable implementation of case-finding strategies,” noted the National Action Plan to Prevent HAIs in 2013.
“Strategies that make use of existing electronic clinical data sources for creating process and outcome measures may have a number of important advantages, including decreasing the burden of data collection, reducing error introduced by poor inter-rater reliability, and providing the ability to track adverse events longitudinally over the spectrum of a particular patient’s receipt of health services.”
At the time, AHRQ, the CDC, NIH, and CMS were concerned that the basics of biological science, epidemiology, infection control interventions, and implementation science needed major attention before organizations could see progress with prediction and prevention.
Since then, a number of new analytics tools, including an emerging generation of machine learning and AI algorithms, have rapidly accelerated the industry’s understanding of the causes and impacts of infection agents, injury pathways, and the human factors leading to other patient safety events.
At Augusta Health in Virginia, for example, combining EHR data with geospatial mapping tools provides a uniquely useful visual exploration of how multi-drug resistant organisms (MDROs) spread between patients.
“We used images of the hospital floor plan imported into Tableau, then we geocoded the locations of the patient rooms on top of that using XY coordinates on those images, so it looks like the rooms correspond to the floor plan,” explained Decision Support Manager Penny Cooper, DHSc, to HealthITAnalytics.com.

“Then we incorporate the positive organisms, the date, and all of the rooms the individual was in – they might move from the ICU to a step-down unit before they might be discharged from our medical unit – so you would see the time period that they spent in each one of those locations.”
Tracing patients along their journey through the physical environment has helped the health system understand patterns in C. diff infections and incidences of MRSA.
As a result of combining multiple sets of data, the organization is better equipped to develop and deploy infection control protocols that are tailored to the real-world challenges of controlling the spread of disease in a complex environment.
“Once we understand those patterns, we can work with our infection prevention experts to say, for example, that we need to be sure to use ultraviolent cleaning techniques in these rooms, or spend extra time making sure that room to the right of the entrance is receiving a little extra monitoring,” added Augusta Health Chief Medical Officer Dr. Richard Embrey.
At Phoenix Children’s Hospital, big data analytics strategies are helping to unite disparate data sets to identify new acute kidney injuries (AKI) before they develop into high-severity events.
Dr. Kanwal Kher, Division Chief of Nephrology, uses real-time surveillance and EHR data to send color-coded alerts for patients who are entering the AKI danger zone.
“Every six hours, we pick up the EHR laboratory data and scan it for patients who have been prescribed medications that can harm the kidneys,” explained Kher. “Then we check to see whether these patients have had kidney function tests ordered for them.”
“If they have, we calculate their net kidney function in the background and determine whether the results indicate they are at risk of AKI or already experiencing one of the three stages of kidney failure.”
Using a relatively new biomarker called NGAL to monitor patients allows Phoenix Children’s to flag high-risk cases just hours after onset of the initial injury, allowing providers to take action much more quickly than if the risk score used traditional creatinine levels to fuel the algorithm.
As a result of deploying the strategy, the number of AKI cases dropped by 34 percent within one year, despite the fact that the hospital admitted more patients during that time.
The progression of AKI through the three stages of injury also slowed, with significantly fewer patients reaching the most severe grades of the condition.
“We’re very pleased with the progress so far, but we know there is a lot more we can do with the data we have and the tools that are going to allow us to continue improving prediction and prevention,” remarked Kher.
Similar projects around detecting sepsis, reducing medication errors, and improving the stewardship of blood resources also rely on innovative big data analytics to identify patient safety concerns early and give providers the information they need to make better decisions about patient care.
Healthcare Big Data Success Starts with the Right Questions
Machine Learning Offers Timely Predictive Analytics for AKI
Steps for developing and implementing patient safety analytics
Beginning a quality improvement program that centers on patient safety requires healthcare organizations to engage in proactive planning and collaborative development.
As with any other data-driven healthcare project, securing buy-in from executives, technical experts, and clinical staff members is a critical requirement for success.
In order to secure this support and create a scalable, sustainable patient safety analytics initiative, organizations should consider creating a roadmap that follows these broad steps.
Recruiting the right team and create a culture of change
Patient safety is as much a communication issue as a clinical one. Errors can easily occur when information is lost, misinterpreted, inaccessible in the EHR, or even misremembered.
A coordinated team of healthcare professionals that can communicate clearly and openly is vital to ensuring that risk scores and clinical alerts are received and that the right person follows up on the suggestion or warning in a timely manner.
Patient safety teams may be composed of different staff members depending on the setting and need, but generally include nursing staff, physicians, epidemiologists, and infection prevention specialists.
Nurses play a particularly important in the effort to improve patient safety due to the frequency with which they interact with patients in the inpatient setting. Recruiting nursing champions to explain patient safety procedures, monitor behavior appropriately, and model best practices can help organizations create a solid foundation for improvement.
In one study from 2007, nurses filed 89 percent of patient safety incident reports – compared to just 1.9 percent of reports filed by physicians – indicating that nursing staff may be best positioned to identify errors in the hospital setting.
Nurses are increasingly becoming leaders of data analytics and informatics projects, as well, which may help organizations optimize data collection and dissemination in the EHR.
Physicians must also take the lead in terms of fostering communication between team members due to the authority and influence they hold over their peers. Physicians and surgeons who encourage other staff members to speak up when they feel a patient safety issue is likely to occur can help to craft a culture of non-punitive reporting and patient-centered care.
Several industry leadership groups, including AHRQ, offer detailed toolkits and other resources to help organizations pinpoint gaps in their existing processes and cultural approaches to patient safety prevention and reporting.
These kits include outlines for implementing and sustaining patient safety improvement projects across a number of key areas of concern.
Identifing a well-defined use case
Every item on the Medicare HAC list offers a potentially impactful use case for developing predictive patient safety analytics, but tacking the entire constellation of adverse events at once may not be feasible for most organizations.
Choosing a clearly defined use case with both a financial and a quality component can help organizations target their efforts to reduce the most harm with the most efficiency.
CAUTIs are among the most common HACs, and may be a promising place for organizations to start implementing protocol changes. Up to a quarter of hospital patients receive a urinary catheter at some point during their stay. Approximately 75 percent of urinary tract infections are associated with catheter use.
The Centers for Disease Control and Prevention (CDC) is one of several organizations offering extensive guidelines for CAUTI prevention, starting with carefully evaluating which patients will receive a net benefit from the procedure.
Developing an EHR risk score based on the expected or actual duration of catheterization, patient comorbidities, and known risk factors such as age and gender could help clinicians avoid unnecessary usage and reduce the likelihood of infection.
An analytics tool could alert providers when catheters have been used for longer than recommended based on the patient’s status, or if a staff member failed to complete or document a care task within the suggested time window.
Sepsis is also a high-impact area to consider. While the disease often goes undetected by human clinicians in its early stages when it is most treatable, analytics tools have shown substantial promise with getting as far ahead of the deadly disease as possible.
Using time-stamped EHR data to chart a patient’s deterioration over time can identify subtle changes in key metrics such as blood pressure and lactic acid levels.
“EHR data is very detailed. There’s a lot of time-stamped information,” said Jeremy Weiss, MD, PhD, Assistant Professor of Health Informatics at Carnegie Mellon University’s Heinz College, who is using a machine learning algorithm to provide early warnings for the disease.
“A lot of classical analyses don’t get to capture that kind of information. With EHRs, where this data is automatically entered, we can look at the temporal progression of disease and update our risk models more adeptly.”
Balancing big data, fast data, and smart data
Time is of the essence when working to save a patient with sepsis, and accessing data in as close to real-time as possible is vital for equipping providers to react to a patient downturn.
But not all data needs to be available at lightning speed, and trying to develop an infrastructure that allows for universal immediate access may not be the most effective use of resources, cautions Dr. Danyal Ibrahim, Chief Data and Analytics Officer at Saint Francis Care in Connecticut.
Velocity, one of the many “V’s” of big data analytics, is a key consideration for designing the underlying data pipelines.
“We have a high velocity alert program [for sepsis alerts] that fits within the workflow and the electronic health record,” said Ibrahim. “If the patient meets certain criteria – they may have a fever and have vital signs or lab results within the warning range – the care team will receive an alert that this patient may be septic.”
“The team can immediately reorient their approach to the patient and take action to give him antibiotics or fluids or conduct other tasks based on best practices to mitigate the problem.”
For longer-term observation of patient safety improvements, however, real-time data may not be the top priority.
“For quality improvement, we will need to look at reaction times and outcomes across the whole hospital,” he added.
“How many patients did we manage correctly? How many cases could we have addressed more quickly? What teams performed better than others, or what areas in the hospital did better, and what are our opportunities to improve?”
The data to support the work of a patient safety improvement team will need to be gathered over weeks or months to give organizations a broader view of performance. Providers will need to be able to integrate multiple data sets, such as claims, EHR time stamps, lab reports, and diagnosis codes. But they don’t need to do so at high velocity.
Understanding how to tailor data access and reporting to specific use cases will help organizations move from volume to value with their analytics programs as well as their financial contracting models.
Integrating analytics seamlessly into the workflow
Focusing on “smart data” instead of just big data will also allow organizations to succeed with one of the most challenging aspects of patient safety: creating meaningful alerts and alarms that do not overwhelm providers or negatively impact the workflow.
Alert fatigue is a major contributor to EHR dissatisfaction and provider burnout, and consistently ranks among the top patient safety hazards and technology use pain-points across the industry.
For more than half a decade, experts have been warning that too many alarms, pop-ups, hard stops, and messages could distract providers, cause clinicians to tune out important alerts, and even lead to early retirements.
A 2016 study in JAMA Internal Medicine found that physicians spend around 66.8 minutes per day processing an average of 77 notifications from their EHRs, many of which are low-value or do not require immediate attention.
Conducting comprehensive usability testing before launching a new patient safety alert function can help to ensure that providers receive the right alerts at the right time with the right information, says the Pew Charitable Trusts.
“Usability testing focused on safety should include all parties, including developers, doctors, nurses, pharmacists, and all other healthcare professionals who will regularly use the system,” the group advises.
“Those tests should take place throughout the lifecycle of an EHR, including during development and after implementation and customization at a facility.”
Creating a patient safety collaborative group to identify and report concerns about EHR functionalities can also foster discussion and give users a chance to share their challenges in a structured, productive manner.
EHR optimization workgroups can take advantage of the ONC SAFER Guides, which focus on identifying and addressing vulnerabilities in EHR workflow design to improve safety and streamline the user experience.
Collecting feedback from clinical users is a critical step for deploying iterative design principles into the health IT environment, notes Malaz Boustani, MD, MPH, Founding Director of the Indiana University Center for Health Innovation and Implementation Science of the Indiana Clinical and Translational Sciences Institute.
“We need a strategy that can account for the fact that humans, with all their quirks and individuality, are making a lot of the decisions in the care environment,” he said of the challenging task of creating quality improvements across highly complex systems of care.
“Agile implementation allows you to avoid being paralyzed by perfection. The first version of anything will not be perfect. If you can accept that your technique doesn’t need to be flawless before appropriate implementation, you can do much more – and do it quickly. Develop a prototype, make sure you have sensors in place to collect meaningful feedback, and use that data to revise and react to what isn’t functioning at its best.”
Carefully monitoring the deployment of new alerts or clinical decision support features while integrating user feedback into the optimization process can be an effective way to fine-tune digital patient safety improvement efforts.
“If your industry is primarily dominated by humans, you need to remember that humans are emotionally-driven decision makers,” Boustani said. “They don’t adhere to logic all of the time. So you need to build your solutions to match that environment, not expect people to match your software.”
“Agile implementation helps to bridge some of those gaps and provide a framework for achieving results, but if you don’t design your processes and health IT systems with the user top of mind, you aren’t going to get the outcomes you’re looking for.”
EHR, CPOE “Value Chain” Begins and Ends with Patient Safety
Patient Safety Errors are Common with Electronic Health Record Use
What defines success?
In the clinical environment, “success” takes multiple forms.
The primary goal, of course, is avoiding harm to the patient. Reducing the number of hospital acquired conditions over a given period of time is a fairly concrete metric for success.
But organizations can also monitor their progress by using additional performance indicators, such as:
- Reducing the severity of events by catching pressure ulcers earlier or becoming more proactive with appropriate antibiotic use in cases of infection
- Recording and reviewing “near miss” events such as medication errors that are identified before administration
- Observing the number of voluntary reports of patient safety concerns before and after education about communication and accountability
- Collecting feedback from patients and family members about their level of trust and comfort with staff actions such as hand hygiene or transitions of care
- Analyzing utilization metrics, such as 30-day readmissions or emergency department use to understand patterns in healthcare associated events
- Recruiting external experts or consultants to provide regular reviews of safety and suggestions for how to improve
In general, organizations should be looking at more than just their numbers as they work to create a culture of accountability and safety.
Clinicians, patients, caregivers, and other staff members should receive encouragement from the very top levels of the organization to report concerns, volunteer ideas for improvement, and communicate honestly in an open, non-punitive environment about how to keep patients healthier.
Providers must be comfortable with identifying weaknesses in the care process that may lead to harm, and should be empowered to participate in the process of optimizing health IT tools and developing reliable, repeatable, standardized workflows to prevent negative outcomes.
Developing and sustaining such a culture may be the ultimate measure of success with a patient safety improvement initiative, even if it is difficult to quantify.
Leveraging a combination of big data analytics tools and patient-centered workflows can help hospitals and health systems avoid penalties while keeping patient safety at the core of their quality improvement goals.