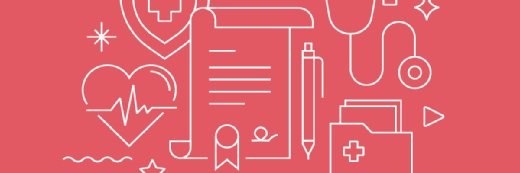
cnythzl/DigitalVision Vectors vi
Turning Healthcare Big Data into Actionable Clinical Intelligence
How can healthcare organizations turn their big data assets into actionable clinical intelligence?
Healthcare organizations on the hunt for lower costs, better outcomes, and value-based care bonuses have invested heavily in hoarding as much big data as they can get their hands on.
From customer service call logs and clinical documentation to satisfaction surveys and patient-generated health data from the Internet of Things, providers of every size and specialty have fully accepted the notion that no scrap of information will go to waste in the era of machine learning, artificial intelligence, and semantic data lakes.
This may be true in the very near future. In just the past few years, the healthcare industry has made huge leaps forward in clinical decision support and predictive analytics.
The use cases for big data are proliferating rapidly as organizations move deeper into population health management and accountable care, and consumers are keeping pace with their growing demand for cost-effective services that leverage the convenience of their favorite apps and devices.
But despite the data-driven promises looming just over the horizon, the majority of healthcare organizations still have a great deal of work to do before they can turn their budding big data analytics competencies into truly actionable clinical intelligence.
A chronic lack of direction, exacerbated by deeply entrenched interoperability issues and a widespread inability to secure a qualified data science team, have left organizations in something of a slump. A series of industry surveys from recent months point out significant staffing gaps, frustrating health data exchange roadblocks, and organizational planning deficiencies that are keeping providers from breaking through their data doldrums.
“The point of analytics is to help make better decisions on a timelier basis,” says Dr. Danyal Ibrahim, Chief Data and Analytics Officer at Saint Francis Care. “But as we all know, there are so many times when our data ends up siloed. One component goes to the finance department, another to IT, and another to the quality improvement team.”
“So even though the data is supposed to be connected around a single patient’s story, ultimately it lands in different siloes all around the organization, and that can be a big barrier to using data to improve care.”
In order to develop a successful big data analytics initiative that can overcome every obstacle from data collection to point-of-care reporting, providers must not only understand where the challenges lie, but also what lies ahead once they overcome their issues.
What does it mean to achieve success with big data analytics, and how can healthcare providers reach their ultimate goal of extracting valuable insights from their rapidly expanding data stores?
Understanding the big data analytics spectrum
If “big data analytics” still seems like a vague and nebulous concept despite its rise to buzzword prominence, that’s because it is. It is little more than a broad term for the act of smashing two or more data sets together to generate information unavailable from a single source.
Underneath the umbrella of big data analytics, however, are some more helpful distinctions. The idea of analytics is generally divided into three major categories: descriptive, predictive, and prescriptive.
“Even though the data is supposed to be connected…if it lands in different siloes, that can be a big barrier to improving care.”
Having a clear idea of where a provider falls on this continuum can help the organization identify the next key competencies to develop on the way to turning its big data into smart data.
Descriptive analytics is where most organizations start. The majority of healthcare data, including clinical documentation, claims data, patient surveys, and lab tests, tell clinicians what has already happened.
The data describes events in the past to answer basic queries such as how many patients acquired an infection in the ICU last June, when is the busiest time of day for the walk-in clinic, or how many individuals in a given population have a diabetes diagnosis in their records.
While this information is a good first step, and it can be valuable for clinical and operational management, there is only so much one can glean from a historical record. Descriptive analytics does not include forecasting or trending. It is simply the process of turning raw data into a report or other format that humans can consume.
Predictive analytics is the ability to extrapolate the course of future events from descriptive data. In order to tell a provider what is likely to happen in the near future, predictive analytics typically requires large volumes of real-time data, or something close to it, to generate an accurate, detailed, and precise trend line or risk score.
This can be a challenge for organizations that have been unable to break down data siloes that keep packets of information separate from one another. And it typically requires more robust health IT capabilities than a basic electronic health record can provide.
As of September 2016, just a third of hospitals were using some form of predictive analytics for patient care or financial modeling, which shows just how difficult it can be to make the leap from having data to using it for actionable decision-making.
Prescriptive analytics is an even loftier goal. It doesn’t just answer the question of what is probably going to happen. Prescriptive analytics can tell a user what course of action would produce the highest likelihood of maximum benefit when a predicted event does occur.
This is the aim of clinical decision support applications and many of the artificial intelligence initiatives that are currently cropping up across the industry.
Prescriptive analytics is starting to emerge most strongly in precision medicine, where algorithms can suggest a therapy that is most likely to produce a positive response based on the patient’s unique genetic makeup and clinical situation.
Elsewhere in the industry, however, prescriptive analytics is currently more or less a pipe dream. Healthcare organizations are still struggling to crack the code of descriptive analytics, especially when meaningful insights require more than one type of data.
The Difference Between Big Data and Smart Data in Healthcare
How to move from description to prescription
Climbing the clinical intelligence ladder requires healthcare organizations to fundamentally reevaluate how they generate, collect, store, process, and report upon their data assets. That means developing a roadmap that identifies every pain point along the journey.
“You need to start with a plan, and that plan has to stem from your leadership – on the clinical side, the business side, and the technical side,” said Sanket Shah, Professor of Health Informatics at the University of Illinois at Chicago.
“The game plan needs to be comprehensive before you begin. You can’t just get going and then decide that you’ll wing it when it’s time to make a decision.”
Organizations should begin the big data process by bringing together a multidisciplinary team, headed by an executive champion, which includes physicians and nurses as well as strong representation from the IT department and health information management department.
"You can’t just get going and then decide that you’ll wing it when it’s time to make a decision.”
Ibrahim suggests that the team tackle six fundamental questions about data access and analytics capabilities in order to pinpoint the organization’s location on the big data competency spectrum.
- Do we have the data and analytics to connect to the important data sets involved in care quality and patient safety, operations, and revenue cycle management?
- If we have access to the data, is it integrated in a meaningful way? Can we look at that data and tell meaningful stories about what is happening, where it’s happening, and why it’s happening?
- Even if we have the data and it’s integrated meaningfully and we can start to tell that story, do we apply some statistical methodology to the data where we aggregate and report on it?
- If we have the data, and it can tell us a story, and we use a good analytics methodology, are we able to present it in an understandable way to all our stakeholders, from the front-line clinician all the way up to the chief executive?
- Are the analytics really meaningful? Does the information help to make decisions? Is it rich enough that we can really figure out why something is happening?
- Lastly, even if we have accomplished all these other goals, can we deliver the information in a timely fashion to the people who need this data to do their jobs?
This process can help providers uncover which areas of the data collection and analytics process need the most attention.
Once the analytics leadership team has identified opportunities for improvement, they can develop change management strategies aimed at improving the quality of documentation, establishing more robust data governance processes, or purchasing new technologies to fill a data management need.
It is important for providers to secure buy-in among all staff members who are likely to be affected by these changes, says Todd Ellis, Principal of Management Consulting at KPMG.
“You have to look at change management as an issue that impacts the entire continuum of care,” he said.
“You never want to have a push-down mandate where you just tell everyone that this is the way you’re going to do things. It’s important for the C-suite to have a vision, but they have to be strategic about turning that vision into a reality that everyone can believe in – and more importantly, a vision that everybody wants to work towards.”
At some point during the process, organizations are likely to discover a need for new software to support their progress.
Providers have a number of infrastructure options depending on the use cases at hand. From quality reporting tools and population health management platforms to supply chain management and patient flow analytics, vendors are offering packages and modules that address every conceivable business problem.
“It’s important for the C-suite to have a vision, but [it has to be] a vision that everybody wants to work towards.”
Employing the same comprehensive, goal-oriented planning techniques will be essential for avoiding wasted effort and purchasing technology tools that accelerate the organization’s progress towards prescriptive insights.
Organizations may benefit from adding the following set of questions to their data analytics assessment process.
- What areas of the organization are in need of support from a data-driven analytics platform? Do we have representatives from each of these areas on our big data team?
- Is there a specific project or use case that will produce a short-term return on investment to build confidence, experience, and demonstrate success?
- What are our upcoming regulatory and financial challenges? How can we use big data to address them?
- Where do we see our organization in five years? Ten years? Do we want to prioritize growth, cooperative partnerships, patient care quality, or our reputation within the community? What data do we need to achieve those targets?
Developing a firm grasp on these issues will help providers identify what health IT tools can help them achieve their goals, as well as flagging areas where the organization might benefit from a consultant, a vendor partner, or an outsourcing initiative.
The Role of Healthcare Data Governance in Big Data Analytics
Preventing Big Data Pain Points During a Healthcare Encounter
Reaping the rewards of clinical intelligence
Architecting a big data analytics initiative with prescriptive insights in mind can be complex and challenging, but there are prizes to be won every step of the way. Improvements in patient safety, outcomes, and satisfaction are possible even with descriptive reports.
In the oncology infusion center at Wake Forest Baptist Health in North Carolina, an analytics product that generated a closer look at patient utilization rates allowed Karen Craver, Clinical Practice Administrator to improve a patient flow pattern that frequently put the center over capacity.
“The nursing staff seems to be less harried,” she told HealthITAnalytics.com after the implementation. “The pharmacy is able to handle requests in a timelier manner. And patients are seeing a reduction of delays in the treatment area, which makes them happier. We have gained about a ten percent increase in overall chair utilization, and we have definitely gained a more positive working atmosphere.”
“You may think that you have a good feel for what is going on in your organization, but until you can actually pull some data points and take a good hard look at it, you probably don’t know as much as you think,” she added.
When organizations add predictive analytics to the mix, the results keep getting better. Sepsis patients have been among the greatest beneficiaries of the industry’s big data analytics push. Data-driven risk assessments and alerting tools can significantly improve the speed and thoroughness of care for this fast-moving killer.
One predictive analytics tool developed at the Mayo Clinic cut the time between initial symptoms and treatment by half. It also reduced manual screening hours by 72 percent, allowing nurses to focus on other care duties without putting patients at risk.
Other predictive tools can prevent falls among nursing home patients, forecast spikes in emergency department visits for asthma, and improve medication adherence rates for diabetics.
"Until you can actually pull some data points and take a good hard look at it, you probably don’t know as much as you think.”
In 2016, Montefiore Health System launched a pilot aimed at identifying any patient at a high risk of death or intubation within 48 hours of hospitalization.
“[The algorithm] creates risk scores based on the patient’s likelihood of a major event within 48 hours,” explained researcher Parsa Mirhaji, MD, PhD. The project, which leverages the health system’s semantic data lake, edges into prescriptive analytics as well.
“There’s another engine that kicks in based on those risk scores and other factors to determine what we can do for that particular patient to avoid the crisis,” he added. “It can send a personalized checklist of proposed interventions to the practitioner in charge of that case.”
Oncology is headed in the same direction, with clinical decision support platforms like CancerLinQ that deliver personalized recommendations to providers based on their patients’ data.
And no discussion of prescriptive analytics would be complete without a mention of the burgeoning machine learning ecosystem that is helping some providers skip over the drudgery of preparing and leveraging their own data at all.
The growing machine learning as a service (MLaaS) industry allows providers to tap into pre-curated datasets drawn from a wide variety of sources.
IBM Watson, for example, does the hard work of churning through millions of lines of text from journal articles and studies, thousands of patient genomes, and petabytes of electronic health record data before providing a recommendation to the end-user about the right drug, treatment, or diagnosis for an individual patient.
Partners HealthCare is taking a similar approach with its Integrated Data Environment for Analytics (IDEA) platform, which uses data lake technology to let researchers reuse existing data sets for study after study.
Machine learning, which can reuse past results to inform its next predictions, promises to open up the door to widespread prescriptive analytics and truly actionable clinical intelligence.
While healthcare providers are still some time away from integrating these approaches into every facet of the organization, they should be preparing right now for a future in which big data is at the core of each and every function.
Doing so will allow them to take advantage of what healthcare analytics has to offer: a patient-centered ecosystem in which timely, accurate, and meaningful data drives the informed decisions of clinicians and helps to produce better outcomes across the entire continuum of care.
How Healthcare Can Prep for Artificial Intelligence, Machine Learning
Artificial Intelligence in Healthcare: Augmentation or Companionship?