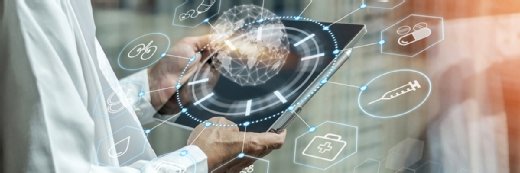
Chinnapong - stock.adobe.com
Top data analytics tools for chronic disease management
Effective chronic disease management requires healthcare stakeholders to effectively utilize data, population health management systems and predictive analytics tools.
Addressing the burden of chronic diseases like heart disease, diabetes, cancer and hypertension across patient populations creates a significant challenge for the U.S. healthcare industry.
To date, an estimated 129 million Americans have at least one chronic disease, with prevalence increasing nationwide. The resulting strain on the healthcare system is also expected to increase, leading stakeholders to pursue chronic disease management strategies focused on disease management programs, care coordination, member education and preventive care.
But to support these efforts, healthcare organizations must deploy a host of tools and resources -- including health IT and data analytics. In the era of EHRs, the collection and storage of data have become much more accessible than they once were, allowing health systems to use that information to bolster population-level initiatives to improve health outcomes.
The Agency for Healthcare Research and Quality indicates that the deployment of health IT tools can have a significant positive impact on chronic disease management by enabling stakeholders to enhance care delivery, provider information-sharing and patient education.
But recent trends in chronic disease management show that choosing and adopting analytics tools for these purposes remains a challenge.
This primer will detail, in alphabetical order, five of the top data analytics technologies that can be used to drive chronic disease management efforts.
Population health management technologies
Chronic diseases impact not only individual patients, but entire patient populations. Further, chronic disease burden varies significantly from health system to health system, which makes finding one-size-fits-all solutions impossible.
Developing a population health management (PHM) strategy can help. Addressing chronic disease with PHM strategies is not a new concept, as population health and chronic disease management initiatives are often inextricably linked.
In both cases, translating clinical knowledge -- in the form of data -- into actionable insights is a top priority. To do so, healthcare organizations are increasingly investing in data analytics tools for PHM.
Technologies designed to improve population health-based data integration and sharing are just one option that can bolster chronic care management. These tools aim to help consolidate data from a variety of sources -- such as EHRs, disease registries, social determinants of health (SDOH) information and claims data -- into data repositories. In turn, these repositories are useful for identifying chronic disease populations, assessing their care and streamlining care delivery.
These tools are also valuable for identifying and reducing health disparities, which disproportionately impact certain chronic disease subgroups.
Other data-driven tools, including patient engagement technologies, are also critical to the success of PHM and chronic care management efforts. These tools can help emphasize patient access to care, the use of self-management apps to encourage behavior change and digital health literacy.
Alongside these technologies, many chronic disease management tools also incorporate predictive analytics.
Predictive analytics
Predictive tools are becoming more prevalent across the healthcare industry as high-value use cases become more apparent, but what these use cases have in common is that they allow health systems and payers to forecast potential future patterns and trends.
Predictive modeling provides a host of benefits for healthcare, but in the context of chronic disease management, predictive analytics can help track disease prevalence, comorbidities and patient outcomes over time, enabling more proactive care coordination.
In general, healthcare big data analytics is useful for informing preventive health initiatives, including flagging gaps in chronic care management. Predictive analytics can help healthcare organizations dig into these insights, enabling the identification of at-risk patients likely to benefit from interventions to prevent chronic disease occurrence or progression.
Predictive analytics tools can also be utilized for a host of other disease management tasks outside of prevention, such as early detection, the development of personalized treatment plans, improved healthcare resource allocation and enhanced operational efficiency.
Predictive analytics is also often a core component of AI-driven chronic disease management software. The use of AI technologies in healthcare has generated significant hype across the industry, as these tools can process more data at faster speeds than other approaches.
For chronic disease, AI holds significant promise for tackling the inherent complexities that patients and providers face, including risk screening, addressing immediate, near-term and long-term care needs, and exploring precision medicine approaches for disease management.
Predictive analytics and AI are also closely related to risk stratification, which health systems might wish to pursue as part of their chronic disease management work.
Risk stratification
Risk scores and risk stratification are key pillars of population-level care management initiatives, including those focused on chronic disease.
Risk stratification is useful for helping healthcare stakeholders categorize patients based on health status, alongside clinical, behavioral and social characteristics. In doing so, stakeholders can manage patient populations based on risk level and make resource allocation more efficient, enabling proactive, risk-stratified care coordination.
This is particularly valuable for chronic disease management, especially when incorporated into predictive analytics tools. Utilizing risk scores can generate insights into the needs of a given patient population, creating opportunities for improved care quality.
When these insights are incorporated into predictive models for chronic disease, however, they can be used to guide early detection and diagnosis efforts, inform potential disease progression trends and personalize treatment plans.
Risk stratification can also bolster health equity by making SDOH information more actionable. Patients living with chronic disease face a host of hurdles to care management, many of which are related to factors like care access and discrimination.
Integrating SDOH into risk prediction models helps not only measure how these factors impact care, but also plays a key role in empowering stakeholders and patients to work together to address them. Further, these strategies can contribute significantly to value-based care success.
But developing risk scores and risk stratification tools requires a wealth of clinical and other data, some of which can be sourced from patient wearables.
Wearables and remote patient monitoring
Wearables, such as smartwatches, are just one of many remote patient monitoring (RPM) technologies. At its core, RPM serves to help care teams monitor patients between clinic visits or in scenarios where in-person care isn't feasible.
These tools -- including continuous blood glucose monitors, pulse oximeters, blood pressure monitors and other types of sensors -- can be used for both acute and chronic care management.
The modality offers a host of benefits for those managing chronic conditions, giving providers the ability to monitor patients in near real time and make any necessary adjustments to care to improve outcomes. RPM also provides an additional source of data that can be used to inform chronic care management strategies for conditions like diabetes, hypertension and cancer.
To date, healthcare organizations utilizing these tools and the data they generate are finding success in reducing chronic disease care gaps for Louisiana's Medicaid beneficiaries and enhancing chronic cardiac care.
Those with existing RPM programs can also scale them to advance chronic disease management and boost patient outcomes.
The data from these tools and other technologies can also be pooled for use in visualization.
Visualization
Data visualization refers to "the practice of translating information into a visual context, such as a map or graph, to make data easier for the human brain to understand and pull insights from. The main goal of data visualization is to make it easier to identify patterns, trends and outliers in large data sets."
Healthcare data visualization is critical as the industry generates more and more data over time. Visualizations help communicate information, such as data trends, more effectively than if the same insights were presented in the form of raw data.
There are various data visualization techniques healthcare organizations can use, depending on the data being presented, including tables, charts, graphs, maps and big data dashboards.
Dashboards are particularly useful for displaying insights generated via EHRs and other data.
In a 2023 interview, Regenstrief Institute leadership detailed how information pulled from EHRs could significantly bolster both public health and chronic disease management by illustrating granular trends in state and local disease burden.
This work has recently shown promise for helping those living with sickle cell disease -- inherited blood disorders that create significant chronic care burdens for those living with the condition -- through the release of a public health dashboard.
The Indiana Sickle Cell Dashboard is designed to provide interactive visualizations of sickle cell disease prevalence and burden, enabling patients, providers and public health officials to guide care management and population health initiatives.
Similar tools, like the CDC's Chronic Disease Indicators (CDI) tool, provide data on state and national estimates for a host of chronic diseases and their risk factors. Many of these can break down and visualize data to highlight chronic disease trends based on race and ethnicity, age and sex, which can promote evidence-based decision-making for PHM and promote health equity.
These five subsets of analytics technologies are not the only tools that can support chronic disease management initiatives, but they provide a robust foundation for healthcare organizations to build on.
Shania Kennedy has been covering news related to health IT and analytics since 2022.