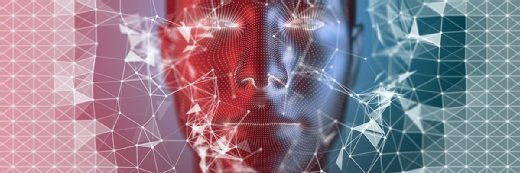
Getty Images
The Healthcare Data Cycle: Generation, Collection, and Processing
Understanding data generation, collection, and processing can guide stakeholders looking to tackle various data analytics projects in healthcare.
As data analytics become more necessary to advance population and public health, healthcare stakeholders may find themselves increasingly working on analytics projects. The outcomes of these projects depend on many factors, but healthcare organizations can increase the likelihood of success by understanding the basics of the data lifecycle or data processing cycle.
The data processing cycle generally consists of the following steps: data generation, collection, processing, storage, management, analysis, visualization, interpretation, and disposal. While these phases are essentially the same across projects and industries, in healthcare, there are particular considerations that can help drive improved project outcomes.
This primer is the first entry in a series that will break down these steps and dive into how healthcare stakeholders can navigate each successfully. Some of the terms related to each phase of the data processing cycle have been explained in more detail here.
Below, HealthITAnalytics will outline data generation, collection, and processing.
DATA GENERATION
Data generation is simply the creation of data. In healthcare, data are nearly constantly generated as patients interact with the health system and payers, receive care, and navigate the billing process.
While data are being generated all the time, not all of those data are captured. Further, the extent to which the data are captured can vary. In some cases, synthetic data are generated whenever appropriate or high-quality real-world data are unavailable for analysis.
Data generation can present a unique challenge for biomedical research, particularly studies aimed at testing healthcare artificial intelligence (AI). These AI models often require massive datasets for training and validation before they can be deemed equitable and safe for clinical use.
The University of Florida is working to address these challenges through a new data generation project, in which researchers will generate and expand biomedical datasets used to monitor patients with critical illnesses. These data are set to advance research into AI algorithms for critical care.
After data are generated, they are collected in the next step of the data processing cycle.
DATA COLLECTION
The United States Department of Health and Human Services (HHS) Office of Research Integrity (ORI) defines data collection as “the process of gathering and measuring information on variables of interest, in an established systematic fashion that enables one to answer stated research questions, test hypotheses, and evaluate outcomes…While methods vary by discipline, the emphasis on ensuring accurate and honest collection remains the same.”
Healthcare data can be collected from various sources: electronic health records (EHRs), patient surveys, claims, administrative data, social determinants of health (SDOH) information, disease registries, public health surveillance, epidemiologic investigations, clinical trials, and peer-reviewed research.
In addition to the data already collected by a health system, other health data resources are also available. The Massachusetts General Hospital (MGH) Institute of Health Professions maintains a collection of frequently used health data sources for US-focused research, while the World Health Organization (WHO) provides data collection and analysis resources for those who wish to leverage global health data.
Stakeholders engaging in a healthcare analytics project will need to select an appropriate data collection approach and create a data collection plan, which helps ensure the collected data are useful, reliable, and cost-effective for the project.
During the data collection step, the Network of the National Library of Medicine (NNLM) recommends that stakeholders give special attention to potential data quality issues and establish a data stewardship approach that prioritizes data validation, backup, and security.
To address potential data quality pitfalls at this stage, the National Association of Healthcare Access Management (NAHAM) also outlined practices for collecting patient attribute data.
Successful data collection has the potential to bolster various care quality efforts.
In 2021, University of Pennsylvania Medical Center (UPMC) leadership detailed how the collection of high-quality, real-world data is required to improve AI applications for advancing chronic disease management.
Data collection can also be used to help address health equity, as the Agency for Healthcare Research and Quality (AHRQ) points out in a recommendation advising healthcare organizations to standardize race, ethnicity, and language data to improve care quality.
However, healthcare data collection does pose potential issues for stakeholders.
In one review article published in the International Journal of Medical Informatics, researchers underscored the ethical concerns associated with passive data collection in healthcare.
Passive data is collected from patients without their active participation through devices like smartphones and wearables. These data can provide important insights into a patient’s health, making them valuable to clinicians and researchers.
Despite the potential value of these data, the authors indicated that informational privacy, informed consent, data security, equity, and data ownership are significant ethical issues posed by this type of data collection and use.
To combat these concerns, the researchers recommend that experts establish an ethical framework prioritizing both patient integrity protections and passive data-driven innovation.
Once data have been collected, stakeholders can move on to the processing phase.
DATA PROCESSING
Data processing refers to converting raw data into a usable and understandable format. This phase of the data cycle can help ensure that the collected information is reliable and in the most appropriate available medium for stakeholders.
During this stage, data may be standardized, normalized, or otherwise transformed depending on data type or intended use.
Researchers writing in Diagnostics earlier this year indicated that data processing plays a key role in advancing medical decision-making, diagnosis, and treatment. This has led to the development of multiple analytics and business intelligence (ABI) tools for data processing.
According to research published in the International Journal of Information Management, stakeholders are also interested in cloud computing’s role in healthcare data processing. Cloud technology can significantly improve health data processing by prioritizing secure data sharing, according to a study published in 2021 in Transactions on Industrial Informatics.
However, a cloud-based approach to data processing is not without its limitations. Despite the focus on security, cloud computing can be vulnerable to information confidentiality and network security challenges.
Some of these can be addressed by leveraging confidential computing or an architectural or algorithmic privacy-enhancing technology (PET).
Technologies like edge computing and AI can also support better data processing during a healthcare analytics project.
Last year, researchers from the University of Chicago’s Pritzker School of Molecular Engineering (PME) developed a stretchable, flexible computing chip that utilizes AI algorithms to bolster personalized health data processing.
Research from the University of California Los Angeles (UCLA) further demonstrated that AI could effectively be used to automate the recording of drug overdose death data and increase data processing speeds, which could spur faster public health response.
Following data processing, a healthcare analytics project can then move into the storage, management, analysis, visualization, interpretation, and disposal, which will be covered in the next installment of the series.
Correction [10/03/2023]: A previous version of this article omitted data disposal as the last step of the healthcare data cycle. The current version corrects this omission.