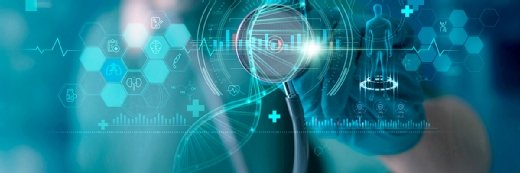
ipopba/istock via Getty Images
Supporting GLP-1 prescribing with digital twin technology
GLP-1s have exploded in popularity, but are not suitable for every patient. A digital twin could provide decision support for clinicians without access to expert second opinions.
Glucagon-like peptide-1 receptor agonists -- a class of medications that mimic the effects of incretin hormones released during digestion -- have surged in popularity recently for use in weight management, diabetes management and other clinical use cases.
Prescribing rates for these drugs have spiked recently, with 1.7% of Americans being prescribed the drugs in 2023 -- a 40-fold increase over the past five years. As demand for GLP-1s has skyrocketed, clinicians are tasked with assessing whether the drugs are suitable for their patients, taking into account side effects like stomach pain, nausea and increased risk of gastrointestinal adverse events.
However, many healthcare organizations might not have access to experts in the use of these medications, creating hurdles for clinical decision-making.
This lack of expertise led leaders at Emory University to explore how digital twin technology could provide clinical decision support for GLP-1 prescribing.
Creating a clinician's 'decision-making digital twin'
Digital twins are virtual representations of real-world entities and processes. In healthcare, these tools can be used to build digital replicas of real communities served by a health system to advance health equity or model the expertise of a clinician.
The latter use case is what Emory is pursuing to improve GLP-1 prescribing. Using an AI-driven approach known as the Tacit Object Modeler (TOM), researchers can select an expert clinician and teach the model how that individual decides whether to prescribe a GLP-1 to a patient.
The tool is designed to "peek into the mind" of Caroline Collins, M.D., a primary care physician with Emory Healthcare and assistant professor at Emory School of Medicine, who has significant experience in lifestyle medicine and an understanding of how people use GLP-1s.
One of the challenges of building a tool like TOM lies in finding a suitable expert.
"It's all dependent on who the twin is modeled after because my clinical judgment may be different from another provider's clinical judgment based on our expertise and our background," Collins stated. "For instance, I'm a lifestyle medicine internal medicine provider. Someone who is a bariatric surgeon might have a different take on the use of GLP-1s."
Choosing the right expert is critical, as these different viewpoints can lead to slightly different approaches to a clinical question like GLP-1 prescribing. Determining what kind of viewpoint one is looking for -- such as expertise in primary care and lifestyle medicine -- is key for ensuring that the model is providing clinical decision support in a way that is appropriate for the setting in which it might be deployed.
To select an expert for the development of a digital twin like TOM, Collins recommended considering relevant certifications -- like board certifications for obesity medicine and lifestyle medicine. Further, choosing an expert who is actively practicing in those fields and has published research in those areas is useful, she added.
Collins underscored that thinking about how rapidly those fields are changing is also crucial. Obesity medicine is evolving rapidly, she explained, in part because of the growing reach of GLP-1s. Selecting an expert who is on top of these shifts and developments is important for deciding how often a tool like TOM needs to be updated to reflect those changes.
But once an expert has been selected, researchers can begin the process of training the model, explained Karl Kuhnert, Ph.D., professor in the practice of organization and management at Emory University's Goizueta Business School, who helped develop the tool.
"In very general terms, what we do is we sit down and interview [the expert] and we ask, 'What do you think about when you think about prescribing a GLP-1?'" he indicated. "This elicits variables that they see as very important."
He further noted that the process takes roughly an hour and a half, and that in Collins' case, she generated 20 key variables. From there, the research team considers each variable and how best to measure each.
This is where the software comes in, producing 150-200 unique clinical scenarios based on these variables and measures. Each scenario is then presented to Collins, who is asked to make GLP-1 prescribing decisions based on that information.
This enables TOM to learn how she weighs each variable in different scenarios to reach her decision. Once the tool can replicate Collins' decision-making with high accuracy, it is ready to provide clinical decision support.
Kuhnert emphasized that the availability of TOM and similar tools on a smartphone could be particularly valuable for clinicians in rural areas or health systems without access to an expert in obesity or lifestyle medicine by surfacing insights they might not have already considered.
Making the model generalizable
However, for TOM to effectively augment clinical decision-making, it must be generalizable to a wide variety of patients. Presenting a variety of clinical scenarios to the clinician can help with this, but providers using the tool must also take into account the unique characteristics of their patients.
Collins underscored that no AI tool is completely free of bias, but by focusing on core variables flagged by an expert to inform decision-making, there is significant potential to mitigate potential biases. Factors like race and socioeconomic status are not incorporated into TOM, but sex is, as a patient's ability to become pregnant is clinically relevant when determining whether a GLP-1 prescription is appropriate.
Characteristics like socioeconomic status can be useful to inform treatment plans and should be considered in shared decision-making with a patient, but as these characteristics do not directly relate to whether that individual would respond well to the medication, they are intentionally excluded from TOM.
Collins noted that by distilling the core variables down in this way, the model is relying on the most important aspects that guide expert decision-making.
Kuhnert explained that traditional AI models rely on large data sets to inform their outputs, which presents potential pitfalls for accuracy and generalizability. With those tools, it might not be clear how current the data is, from where it was sourced or what its quality is.
"What we're really tapping into here is Dr. Collins' wisdom," he stated. "And when we talk about wisdom, we're talking about tacit knowledge -- the know-how that is rooted in context, practice values and, most importantly, lived experience. It's distinct from explicit knowledge, which is codified knowledge in documents [or] databases."
He further highlighted that this wisdom is timely and transparent, characteristics that don't necessarily apply to some traditional AI models or their data sources.
This is particularly important in the realm of GLP-1 prescribing, as the field is changing so rapidly that being able to update TOM as new insights come out is crucial.
"It's a very different way of thinking about using AI, this idea of digital twins," he said. "We have the ability to change when new data or new findings come in, when new science is done on the subject, and so, we're always going to be in the present tense."
Digital twins like TOM also have the potential to transform the way that medical professionals distribute knowledge, he continued. Currently, experts must distribute their knowledge via research papers, books and other academic and clinical mediums.
These tools are valuable, but already over-burdened clinicians might find it difficult to find, review and pull from these sources when needed. Technologies like TOM hold promise for streamlining this process, helping providers spend more time caring for patients.
"The way we're using AI is not to replace people's jobs. In fact, we need the experts because of the nature of change -- things aren't slowing down in this world, especially in healthcare. So, having this technology allows us to actually create more wisdom for physicians, as well as patients," Kuhnert concluded.
Shania Kennedy has been covering news related to health IT and analytics since 2022.