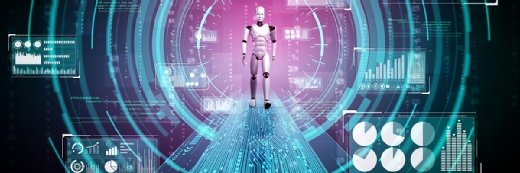
Getty Images
Storage, Management, and Analysis in the Health Data Lifecycle
Data storage, management, and analysis are three of the most crucial steps in a healthcare analytics project, but what does each entail?
The data lifecycle drives data analytics projects across industries, and healthcare is no exception. Healthcare stakeholders need to have a firm grasp on each of the steps in the cycle — data generation, collection, processing, storage, management, analysis, visualization, interpretation, and disposal — for their analytics initiatives to succeed.
This is the second installment in a series devoted to the healthcare data lifecycle, following the first, which explored the data generation, collection, and processing phases.
Here, HealthITAnalytics will dive into data storage, management, and analysis.
DATA STORAGE
Data storage is the “magnetic, optical or mechanical media that records and preserves digital information for ongoing or future operations,” according to the International Business Machines Corporation (IBM).
Data storage involves computers, also called terminals, connecting to a storage device directly or through a network. Under this framework, a user can then instruct a terminal to access and store data held within data storage devices.
Data storage has two foundational components: the form in which the data are recorded and the device used to store that data.
There are three main forms of data storage: file, block, and object.
File storage is the form that most laypeople would be familiar with, as it is a common data storage form used in both personal and professional settings. File storage leverages a hierarchical storage methodology to store and organize data. In the hierarchy, data is stored in files, organized into folders and further classified under directories and subdirectories.
The next form of data storage is block storage, which, as the name suggests, helps store data in blocks. These blocks are stored as separate entities, each assigned a unique identifier. This type of storage prioritizes reliable and efficient data transfer.
Object storage is designed to provide a data storage architecture for large amounts of unstructured information. Data stored in this form either does not conform to the parameters of a traditional relational database — which utilizes columns and rows — or cannot be organized to fit those parameters.
Data from sensors, emails, photos, videos, audio files, web pages, and other textual or non-textual data are often stored in this form.
Two main data storage device types are most common: direct area storage and network-based storage.
Direct area storage, or direct-attached storage (DAS), refers to storage that is directly connected to or in the immediate vicinity of the terminal accessing it. Flash drives, solid state drives (SSDs), and hard disk drives (HDDs) are examples of direct area storage.
Network-based storage devices can enable multiple terminals to access the stored data using a network. This approach can be better than direct area storage because of its off-site storage capability, which can provide improved data protection and backup capabilities. These devices are also useful for data sharing and collaboration.
Health data storage options are typically categorized as on-premise, cloud, or hybrid.
Healthcare organizations are increasingly investing in cloud technologies, according to insights from Gartner.
The rapid growth of health information technology (IT) and digital data over the past few years has spurred the adoption of cloud computing, but healthcare stakeholders looking to deploy one of these tools will have to assess whether public, private, or hybrid cloud storage is suitable for their organization.
To choose the best healthcare data storage option, organizations must consider both their current health IT infrastructure and their future data needs. Considerations related to what kind of data is being stored, where and how those data will be stored, and the storage solution’s ability to scale to meet future needs can provide a place for stakeholders to start.
Virtualization tools can also help stakeholders make the most of their existing infrastructure.
However, those looking to deploy any of these data storage tools must also consider the limitations and challenges they come with, such as privacy, security, scalability, and cost. Outages in cloud storage can also make data stored more difficult to access,
Leveraging solutions like object storage and blockchain can help overcome some of the limitations and challenges, according to experts in The Fusion of Internet of Things, Artificial Intelligence and Cloud Computing in Healthcare in 2021.
The concept of data storage is closely linked to that of data management.
DATA MANAGEMENT
Data management involves “validating, organizing, protecting, maintaining, and processing” data to ensure it is accessible, reliable, and of high quality, according to the National Institutes of Health (NIH). In biomedical studies, proper data management is key to maintaining research integrity, allowing data to be used by more people to drive breakthroughs without compromising scientific rigor or research communities’ best practices.
Health systems can utilize a data management system to help ensure that data is cleaned, standardized, and unified in a way that supports interoperability and internal analytics projects.
The University of Pittsburgh underscores that health data management can bolster patient–provider communication, simplify the diagnostic process, help clinicians provide preventive care, support clinical decision-making, and improve patient outcomes.
Those moving to this step in the data cycle must develop a data management plan, which can better position healthcare organizations to meet their specific needs.
Harvard’s Longwood Medical Area Research Data Management Working Group (LMA RDMWG) conceptualizes data management plans as formal, living documents that outline the types of data used in a project, along with considerations related to the storage, security, privacy, and sharing of those data.
When creating a data management plan, stakeholders should also consider the weaknesses within their approach and data management systems more broadly. One 2020 study published in the Journal of Medical Internet Research posited that more efficient, secure data management systems are needed to improve medical research and clinical care.
The authors recommended that these systems meet a series of requirements related to medical record data, real-time data, patient participation, sharing, security, privacy, and public insights.
Insights from Touro College Illinois indicate that data operating and storage solutions are the foundation for a well-designed healthcare data management system. Such a system should efficiently create structured, searchable, and maintainable databases.
Further, the data management system should integrate with a vendor-neutral archive (VNA) — a technology that standardizes data in a format that works regardless of the data management solution being deployed.
Data management has already shown promise in addressing some of healthcare’s most significant challenges.
In 2021, the Helping to End Addiction Long-term (HEAL) Initiative tapped data management and stewardship expertise from the Renaissance Computing Institute (RENCI) at the University of North Carolina at Chapel Hill and RTI International to advance opioid crisis research.
The initiative will provide $21.4 million over five years to the organizations as they assist NIH-funded researchers from more than 500 studies with preparing and sustaining their data.
RENCI and RTI are also collaborating with a HEAL-funded team at the University of Chicago to build a cloud-based platform, enabling HEAL researchers, providers, and policymakers to find and use NIH HEAL Initiative research results.
Following data management, stakeholders can then move to analysis.
ANALYSIS
The American Health Information Management Association (AHIMA) defines data analytics as “analysis of the data in some way using quantitative and qualitative techniques to be able to explore for trends and patterns in the data.”
Analytics is closely related to the concept of informatics, which AHIMA characterizes as “a collaborative activity that involves people, processes, and technologies to produce and use trusted data for better decision-making. Informatics involves using the data, information, and knowledge to both improve the delivery of healthcare services and improve patient outcomes.”
While the two are related, they are distinct: analytics focuses on the analysis of data, while informatics is concerned with applying the information gathered from that analysis.
Healthcare data analytics can be broken down into four categories: descriptive, diagnostic, predictive, and prescriptive analytics.
Descriptive analytics helps answer the question, “What happened?”
This type of analytics allows users to identify and describe trends in their data that capture what has happened or is currently happening within a healthcare organization. Doing so can provide a surface-level picture of key performance indicator (KPI)-related aspects of the business, like revenue or health system operations.
Since this type of analytics is the most accessible and straightforward of the four and often leverages basic raw data that most organizations already possess, it can be a good place for stakeholders to start when pursuing analytics projects.
Diagnostic analytics helps reveal why a phenomenon highlighted during descriptive analytics is happening.
This type of analytics helps stakeholders dig deeper into unexpected anomalies identified using descriptive analytics data. This enables users to gather additional data or expand the scope of their analysis to highlight what may have caused such a shift in the trends or relationships.
Predictive analytics takes this one step further by providing insights into what’s likely to happen in the future based on descriptive and diagnostic analytics data. By leveraging historical patterns and trends, predictive analytics can infer future patterns.
This makes predictive analytics particularly valuable, as it allows stakeholders to dive deep into the causes behind unmet KPIs.
However, this type of analytics requires large amounts of data, sufficient resources, and personnel to support the analysis, which smaller healthcare organizations may lack.
The final type of analytics, prescriptive analytics, takes all the insights generated from the previous three types and uses them to provide stakeholders with guidance on what should be done or changed to improve performance on a KPI.
This makes prescriptive analytics the most valuable of the four but also the most advanced and elusive. Here, the analysis relies on huge amounts of data and computing ability, making it nearly impossible for most healthcare organizations today.
However, a few health systems are using more advanced technologies, such as quantum computing, to support their data analytics efforts, which may allow them to engage in prescriptive analytics in the future.
There are multiple other technologies that also support analytics of all types, many of which are rooted in healthcare artificial intelligence (AI): machine learning (ML), deep learning (DL), cognitive computing, natural language processing (NLP), and semantic computing.
These tools can boost initiatives related to many of healthcare’s biggest pain points, from population health management to value-based care delivery.
After the analytics step, stakeholders can proceed to the data visualization, interpretation, and disposal stages, which will be explored in the final installment of the series.