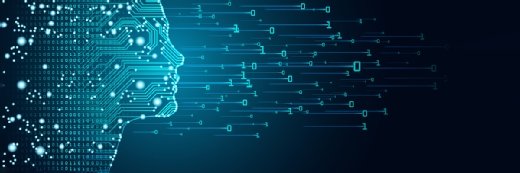
Getty Images
Patient Privacy in Healthcare Analytics: The Role of Augmentation PETs
Balancing data privacy and access is necessary for healthcare analytics stakeholders, and augmentation privacy-enhancing technologies can help.
Healthcare big data analytics efforts must strike a balance between making patient data accessible to researchers and guaranteeing that it is private and protected from unauthorized individuals.
To do so, stakeholders can utilize privacy-enhancing technologies (PETs). For healthcare analytics, experts recommend combining algorithmic, architectural, and augmentation PETs to ensure data security.
This is the third and final installment of a series exploring each PET type and its potential healthcare applications, following breakdowns of algorithmic and architectural PETs.
Below, HealthITAnalytics will take a deep dive into augmentation PETs.
SYNTHETIC DATA
Augmentation PETs can help protect patient privacy by leveraging historical data distributions to generate realistic datasets. These can be used to augment existing data sources, like enhancing a small dataset, or to create fully synthetic datasets. Doing so can improve the utility and availability of datasets used in analytics projects.
Synthetic data are designed to have the same mathematical properties as the real-world data they are based on without containing any of the same information, according to the Massachusetts Institute of Technology (MIT). By using a relational database to create a generative machine-learning model, stakeholders can generate a second synthetic dataset.
Some broad use cases for synthetic data involve using them to mitigate bias and improve artificial intelligence (AI) models, but they are also useful for protecting sensitive data, a top concern in healthcare analytics.
While researchers still prefer real-world data, synthetic data creates opportunities to bridge data access gaps in policymaking and research, according to a study published earlier this year in PLOS Digital Health.
In it, the researchers highlighted seven potential applications of synthetic data in healthcare: simulation and prediction research; hypothesis, methods, and algorithm testing; epidemiology and public health research; health information technology (IT) development; education and training; public release of datasets; and linking data.
Synthetic data has also been used to accelerate COVID-19 research.
The potential for synthetic data in healthcare has also caught the attention of national stakeholders.
The Office of the National Coordinator for Health Information Technology (ONC)'s Synthetic Health Data Challenge launched in 2021 to encourage innovators in the health IT space to enhance Synthea, an open-source synthetic patient generator, or to demonstrate novel uses of the tool’s data.
To enhance Synthea’s ability to generate high-quality synthetic datasets for pediatric populations, patients with complex care needs, and individuals struggling with opioid use, ONC spearheaded the Synthetic Health Data Generation to Accelerate Patient-Centered Outcomes Research initiative.
Experts argue that synthetic data is one of the most promising solutions to address the fact that machine-learning (ML) models can identify patient characteristics—such as sex, age, blood pressure, smoking, diabetes, and COVID-19 status—from anonymized data.
Synthetic data can also help diversify datasets and bolster clinical research while ensuring patient privacy.
Despite these benefits, researchers exploring the vulnerabilities associated with synthetic data in healthcare note that malicious actors can use these data to spread misinformation and deceive facial recognition software through fake impersonation videos, also known as deepfakes.
Additionally, while synthetic data can help develop and improve AI-based medical devices, its role in current regulatory frameworks for modifying AI algorithms in healthcare has not been established. Doing so is crucial to ensure that synthetic data can be used to protect patient privacy and improve clinical decision-making.
Currently, the healthcare industry also lacks objective, robust methods for determining if synthetic data is sufficiently different from the real-world data they are based on, raising questions as to whether these datasets can then be classified as truly anonymous, researchers note. There are also no concrete restrictions to disseminating these synthetic representations of sensitive healthcare data.
The potential of synthetic data in healthcare may prove valuable in the future, but experts writing in BMJ Medicine indicate that additional research is needed to explore the risks and cost-effectiveness associated with these datasets, including to what extent they can be relied upon in formal analysis.
GENERATIVE ADVERSARIAL NETWORKS
Generative adversarial networks (GANs) are a type of deep learning (DL) that leverages neural networks to generate synthetic data. A GAN consists of a generative and an adversarial network to generate realistic images, videos, voice recordings, and other types of data.
The generative network takes the input data and uses it to produce a synthetic version of that data. The results of this process will vary depending on the input and how well-trained the model’s layers are for the desired use case.
The adversarial network pits the real data against the synthetic data, using a discriminator mechanism to differentiate between the two data types.
As the two networks perform these tasks, the results should theoretically improve until the synthetic data is virtually indistinguishable from its real-world counterpart.
Research shows that GAN applications in medicine mostly involve medical image processing, synthesis, segmentation, generation, and denoising.
Other potential use cases for this PET in healthcare include generating synthetic abnormal magnetic resonance images of brain tumors, generating synthetic EHR data, improving AI-based cancer imaging, bolstering single-cell RNA-sequencing, and supporting medical education.
Experts posit that GANs and the synthetic data that they produce have the potential to revolutionize clinical research while protecting patient privacy. They state that using these approaches makes it possible to fully anonymize healthcare data to the point where none of the information is traceable to a real individual within the dataset.
This could enable researchers to replace the use of real patient data in appropriate contexts, in addition to balancing and expanding existing datasets.
However, GANs could also be used by bad actors to engage in “adversarial attacks” on healthcare AI. In such an attack, the GAN could be used to create false images or alter data points to make the AI draw an incorrect conclusion, which would significantly impact patient safety.
Further, GANs are computationally expensive to train, requiring significant investment and resources like graphics processing units (GPUs).
Once the GAN is trained, it can theoretically generate an unlimited amount of synthetic data, but labeling that data is a challenge in healthcare. Accurate “ground truth labeling” is necessary for the development of healthcare AI models, and failing to label data used to train these tools can significantly limit their performance and clinical utility.
Data labeling is typically performed by humans in a labor- and time-intensive manner, hindering how much synthetic data could realistically be labeled and, as a result, used.
The researchers note that it may be possible to label these synthetic data using mature machine-learning models trained on real data in the future. For now, though, this is not feasible, cementing the burden of synthetic data labeling on human stakeholders and limiting the potential of GANs in healthcare.
DIGITAL TWINS
A digital twin is a digital or virtual representation of a physical object, process, system, or person designed to help organizations simulate potential outcomes. The digital twin is typically intended to span the physical twin’s lifecycle, leveraging real-time data updates and machine learning to help support decision-making, according to IBM.
Unlike a standard simulation, digital twins can be scaled to run studies and simulate multiple processes at once, making this PET intriguing for healthcare stakeholders interested in modeling and visualization. Healthcare digital twins could be used to create 3D visualizations of the human body, to assist with diagnosis and treatment, to advance precision medicine, and for predictive analytics. The technology has also been leveraged to streamline hospital operations.
Healthcare digital twins may also help improve health equity.
In February, researchers from Cleveland Clinic and MetroHealth were awarded a $3.14 million National Institutes of Health (NIH) grant to develop digital twin technology to better understand and tackle health disparities within the health system’s population.
The research will build digital twin models using 250,000 patients’ EHR data. These models will then be used to study health trends and complex social, environmental, and economic factors that impact health disparities.
The grant will also support the development of “Digital Twin Neighborhoods” to help better understand various health inequities specific to the Cleveland area.
The project aims to improve place-based population health and outcomes using the data generated by the digital twins.
A study published last year in npj Digital Medicine highlights that one of the major potential benefits of healthcare digital twins is the possibility of gaining insights into the expected behaviors of the physical twin, often a patient, which could significantly advance clinical trials, precision medicine, and public health.
The researchers indicate that the main considerations for translating digital twin research into clinical practice are computational requirements, product oversight, data governance, and clinical implementation concerns.
In addition, some experts note that difficulties in data collection and fusion, alongside simulation accuracy, are significant limitations for current digital twin applications in the medical field. But, moving forward, stakeholders can create high-quality models of patients to achieve personalized diagnosis and treatment by combining healthcare digital twins, big data, AI, and the Internet of Things (IoT), they state.